Data Privacy in the Age of AI: A Recap of Our AWS Webinar
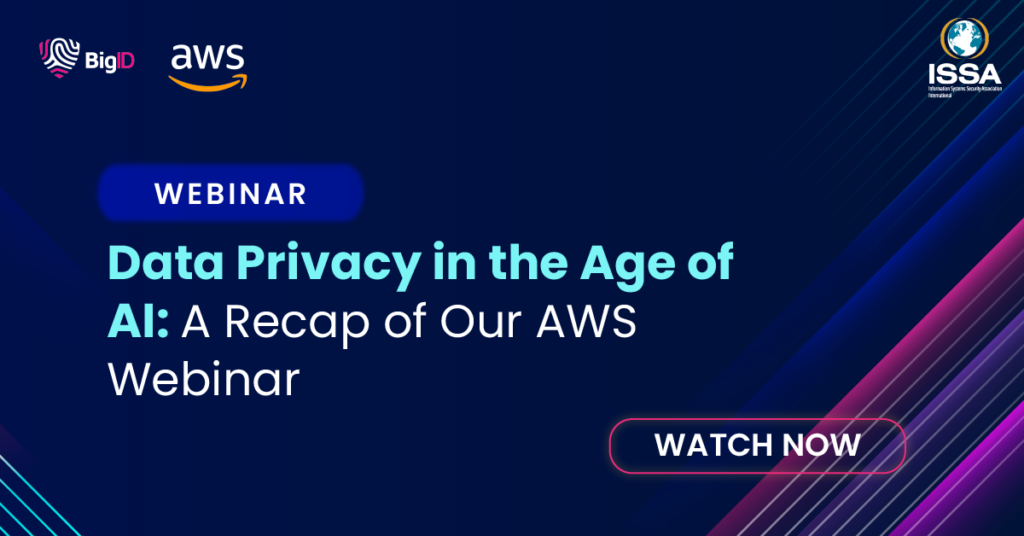
In the ever-evolving landscape of data management, artificial intelligence (AI) has emerged as a game-changer. AI has revolutionized the way we store, process, and analyze data. However, with this revolutionary power comes a great responsibility. Organizations must ensure that AI, especially LLMs, don’t use sensitive, personal, critical, or regulated information as feedstock. Organizations must implement proper AI governance and controls to mitigate privacy risks with unwanted data exposure, leakage, and use.
Our recent joint webinar with AWS explored the challenges and best practices for navigating data privacy and protection in the age of AI and Large Language Models (LLMs). Here are some some of the key areas we covered:
Understanding the Risks in an AI World
In this new era, we discussed the critical importance of comprehending the risks and threats to data privacy. We unveiled the forces shaping data privacy today, from AI’s exponential growth to the evolving regulatory landscape.
One significant risk associated with generative AI, highlighted by Ruben Falk, Capital Markets Specialist at AWS, is the challenge of traceability and verifying the authenticity of generated answers. Falk points out that when you query a generative AI model, it becomes difficult to trace the answer back to its original source or training data. This lack of traceability not only hampers the ability to validate the accuracy of the response but also introduces concerns regarding the potential for hallucinations—responses that might not be grounded in factual information. Falk’s insight emphasizes the complexity of ensuring the reliability of AI-generated content, particularly when understanding the origins and authenticity of the information provided.
The Evolving Landscape of Data Privacy and Protection
We shed light on the latest trends and regulations in data privacy and protection. Staying current with these developments is essential to keep your organization compliant and maintain customer trust.
Christopher Glover, Field CTO at BigID, provides valuable insights into the evolving landscape of data privacy and protection in the context of AI. He highlights the significant challenges companies face as they navigate the intersection of AI and emerging regulations. Glover says, “What’s new is we’re starting to see regulation, and companies are having to prepare for that.” He emphasizes that while various regions are introducing AI regulations, the approaches differ significantly.
Data Governance Challenges in the Age of LLMs
Large Language Models (LLMs) are reshaping AI, but they also introduce unique governance challenges. We examined these intricacies, equipping you with the knowledge to implement robust data governance strategies, especially when LLMs are in the mix.
Ruben Falk, Capital Markets Specialist at AWS, advocates a best practice for LLMs: “Don’t expect the model to know the answer, bring the relevant documents and data to the model… that way you control the fact based on which these answers are based.” This approach enhances traceability, minimizes hallucinations, and allows for cross-verification of LLM-generated responses against source documents, reinforcing the significance of meticulous data control and governance within the LLM context.
Best Practices for a Secure AI-Driven World
When it comes to best practices for ensuring security in an AI-driven world, Christopher Glover, Field CTO at BigID, emphasizes the critical data types that need safeguarding: “Your customer, employee, and vendor data that you have, any kind of sensitive private information… any of the protected classes of data that could be biased… all their internal information.” Glover also highlights the need for vigilance regarding third-party data sources and the potential for malicious code or data introduction. He notes that securing the AI/ML development pipeline is as crucial as protecting the data it processes.
Ruben Falk, Capital Markets Specialist at AWS, adds to this perspective by emphasizing the importance of model security: “Not just the data that needs governance, it’s the models themselves.” He discusses established practices for ML ops, model explainability, and the need to filter and guard against harmful information, especially in the context of generative AI. Falk notes that while some practices are well-established, others, particularly in the generative AI space, are still evolving due to its novelty.
Together, their insights underscore the multifaceted nature of securing AI and the importance of addressing both data and model security comprehensively.
Practical Insights for Real-World Application
We continued beyond theory. Our experts provided actionable advice for applying these insights in real-world scenarios. For those new to AI, the webinar highlighted the importance of education and understanding the technology. Training teams on AI and its appropriate use are essential to lay a strong foundation. Establishing ethical standards and governance controls from the outset helps ensure responsible AI adoption.
The recommendations for those who have already embarked on AI projects included ensuring transparency and explainability in AI models. Implementing frameworks for attribution and explainability is crucial for building trust with stakeholders and end-users. Staying abreast of emerging ethical considerations and societal impacts helps make informed decisions and address evolving risks.
If you missed our webinar, don’t worry. You can watch it on-demand right here. Enhance your understanding of data privacy in the era of AI and LLMs.