Data Governance for Conversational AI and LLMs (Large Language Models)
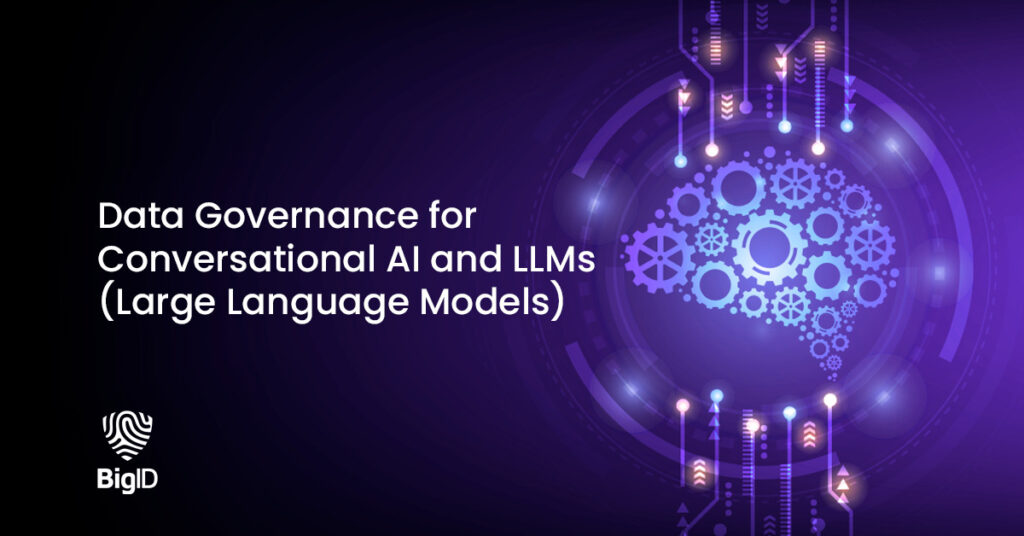
ChatGPT has revolutionized AI in a matter of months. It allows new generative AI frameworks like Large Language Models (LLMs) to essentially impersonate a human. Traditionally, LLMs are trained using a large volume of unsupervised data alongside a smaller set of supervised data – data that’s labeled by humans. Meanwhile, conversational AI is now incorporating unstructured data as well: including repositories like office365, slack, email, files, pdfs, and more.
For organizations experimenting with LLMs, this introduces new risks. Unlike traditional AI frameworks that depend on structured data as input, it’s all about analyzing unstructured data for AI.
This highlights a new risk vector: training LLMs on client data, on customer data, on regulated data – essentially using data outside of the given purpose – can violate consumer privacy and accelerate risk on the data you know, and data you don’t. Even training LLMs on confidential intellectual property likely raises the risk that the confidential information is going to be leaked, breached, or hacked.
What if you could train LLMs on only the data safe for use? Automatically define which data sets are safe for training, effectively governing the data that goes into your AI input data sets.
With BigID, you can. BigID helps organizations find, catalog, filter and govern both structured data for rational AI and unstructured data for newer conversational AI. BigID enables customers to extend data governance and security to modern conversational AI & LLMs, driving innovation responsibly.
BigID catalogs all unstructured and structured data: including files, images, documents, emails, and more – including the data that’s used to fuel generative AI.
Customers can classify, label, and tag data by type, regulation, sensitivity, even purpose of use – across structured data, unstructured data, and everywhere in between. That makes it easier than ever to identify and label sensitive customer, privacy, regulated, intellectual property data, and more. By doing so, organizations can use select appropriate sets of data to train LLMs: data that’s going to be more relevant, low risk, and drive more accurate results.
You can choose to exclude sensitive HR data, for instance, and avoid compromising employee data that is collected and tagged. Or point LLMs to public non-confidential data, ensuring that nothing it’s trained on is going to compromise security or privacy.
As AI and ML become more powerful – through GPT and open source training – it’s more important than ever to manage, protect, and govern that data that’s sourcing the future.