How to Manage Risk and Compliance for Financial Services
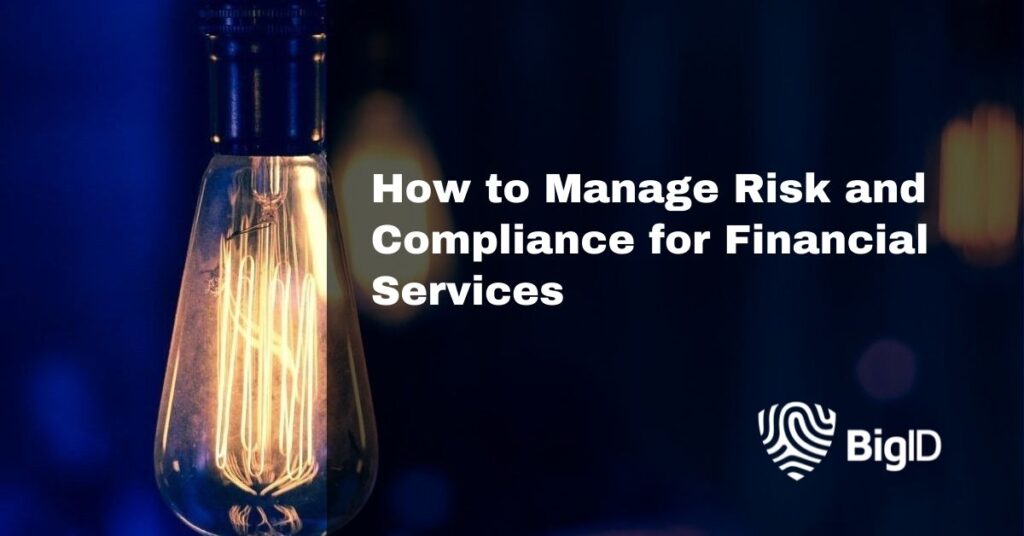
Finance data is sensitive, it’s personal, and it’s highly regulated.
Companies in financial services face specific challenges when it comes to securing the customer data they have, making sure they meet compliance requirements for various regulations, and proactively manage their data to mitigate risk and boost business outcomes.
On top of that, financial institutions tend to sit on high volumes of data, inviting higher risk. It’s difficult to keep up with the data they know they have — to say nothing of dark and potentially high-risk data hiding out (or forgotten) across the enterprise.
Financial institutions need to be able to find, classify, inventory, and manage all of their sensitive data, regardless of where it is or what it is. It’s a momentous task to do so — and requires addressing common challenges like siloed data, lack of visibility and accurate insight, and balancing legacy systems with cloud data. All while meeting a litany of compliance requirements.
This article helps break down these challenges one by one with concrete solutions specifically tailored to unique pain points for financial services.
In This Article, Learn About:
- Financial Industry Regulations
- How to Reduce Risk with Machine Learning
- Align Data Privacy, Securty, and Governance Strategy
- Discover Data to Protect Data
Financial Industry Regulations
Financial services organizations face a lot of regulations. They must comply with constantly evolving laws from various U.S. and global regulating bodies — and properly maintain, secure, and report on their data.
Complicating matters, regulated data varies from law to law. Some regulations focus on nonpublic personal data (NPI), some on material nonpublic information (MNPI), others on personal information (PI), sensitive personal information (SPI), and so on. While the data they encompass overlaps broadly, there are some differences in how each regulation defines that data.
Top regulations facing financial institutions include — but are not limited to:
KYC / AML (Know Your Customer / Anti-Money Laundering)
KYC guidelines — part of the broader scope of a bank’s Anti-Money Laundering (AML) policy — require that professionals make an effort to verify the identity, suitability, and risks involved in maintaining a business relationship.
CCAR (Comprehensive Capital Analysis and Review)
CCAR is a U.S. regulatory framework introduced by the Federal Reserve to assess, regulate, and supervise large banks and financial institutions — otherwise known as bank holding companies (BHCs).
BCBS 239 (Basel Committee on Banking Supervision)
BCBS 239 is a standard aimed at reinforcing banks’ risk data aggregation capabilities and internal risk reporting practices.
SOX (Sarbanes-Oxley Act)
SOX is a U.S. federal law requiring that financial institutions maintain an adequate control structure for their financial records, data protection policies, and reporting.
GLBA (Gramm–Leach–Bliley Act)
GLBA is a U.S. federal law that requires financial organizations to ensure the confidentiality and security of customers’ NPI — and adhere to specific guidelines on how they govern and disclose that data, including third-party sharing.
NYS DFS CRR 500 (The New York Department of Financial Services Cybersecurity Regulation)
The NY DFS requires financial services to maintain a cybersecurity program to protect consumers’ private data and manage cyber risk. It requires a risk-based approach to protecting customer information from being revealed or stolen for illicit purposes.
NIST CSF (National Institute of Standards and Technology Cybersecurity Framework)
The NIST Cybersecurity Framework is a set of guidelines to help organizations manage privacy risk. It establishes a common understanding and set of practices to improve data privacy postures and reduce risk.
PCI DSS (Payment Card Industry Data Security Standard)
The PCI DSS (2006) is a set of requirements intended to ensure that all companies that process, store, or transmit credit card information securely manage credit card data and cardholder information throughout the transaction process.
GDPR (General Data Protection Regulation)
GDPR is an EU data protection and privacy law aimed at giving individuals control over their personal data. GDPR applies to any enterprise that processes the personal information of data subjects in the EU, regardless of where the organization resides — and also addresses the handling and transfer of personal data outside the EU.
CCPA (California Consumer Privacy Act)
CCPA is a state statute that enhances consumer privacy rights and protections for residents of California, and it applies to any enterprise doing business in California. For financial companies, it establishes a broader definition of personal information than NPI, regulates purpose for use, and institutes a private right of action provision.
While these regulations are varied, ultimately they have a common thread: reducing risk and protecting regulated data. They require that financial institutions be able to not only classify sensitive and personal data, but drill down into data for a deeper level of granularity. These organizations need visibility on regulated data, to be able to report on and validate their data practices, and be proactive about protecting the sensitive, personal, and customer data that they collect and process.
The key to addressing multiple and layered regulatory demands starts with knowing your data: it’s critical to be able to discover, identify, and classify all regulated data across an organization’s entire data landscape in order to align with compliance demands.
How to Reduce Risk with Machine Learning
Regulated data is everywhere: in silos, shadow servers, and data streams — across legacy systems and in modern cloud storage.
Traditional technologies that have previously dominated the discovery landscape virtually guarantee that you’ll miss dark and sensitive data lurking in your organization. These tools may only see one kind of data, or may only find data that you already know about — leaving a host of vulnerable information open to risk.
A machine-learning approach to data discovery upends that uncertainty, making it possible to locate, clean up, and manage decades of legacy data that a financial institution might have — and have no idea that they have.
Organizations can use an ML-based discovery-in-depth approach to inventory their data by identity, content, type, and sensitivity, gaining full visibility into all regulated and critical data of all types — from structured to unstructured. By leveraging machine learning for correlation, financial institutions can find and classify data relationships, align inferred data, and identify associated data down to the identity level.
Align Data Privacy, Security, and Governance Strategy
From cloud migrations to data quality to data minimization, financial institutions should align their strategic data initiatives for maximum success — starting with a foundation of data discovery to inform consistent and defensible action.
Data minimization
BigID enables organizations to identify data redundancy and find similar, duplicate, and derivative data. With BigID, organizations can identify the golden copy of each record and gain meaningful insight from that data. Find the data you need to quickly distinguish between real and noisy data in unstructured data environments.
Cloud migration
In order to balance compliance, privacy, security, risk, and cost and efficiency concerns, execute a strategic cloud migration initiative. With BigID, financial institutions can map, monitor, and inventory sensitive data before it’s migrated to the cloud, uncover data quality issues, identify duplicate data, highlight overexposed data, and apply labels based on classification output for automated enforcement in the cloud.
Protect sensitive and regulated data
Proactively protect sensitive, personal, regulated, and critical data — from legacy stores to cloud environments. With BigID, financial institutions get visibility and complete coverage of their sensitive, regulated, and high-risk data. BigID empowers organizations to uncover dark data, manage risk, automate and enforce security policy, and align a security-by-design approach.
Fulfill data privacy requirements
Get full visibility into personal and regulated data across both U.S. and global regulations that impact Financial Services. With BigID, organizations can leverage a complete PI/PII inventory using correlation to drill down the identity level, automate data subject access requests (DSAR) fulfillment, document record of processing activity (RoPA), identify what data is shared with third parties, and enable consent governance. Get customized reporting for each line of business, and operationalize privacy initiatives across the organization.
Improve data quality
BigID enables organizations to improve their data quality, providing insights within business context. Actively monitor the consistency, accuracy, completeness, and validity of your data — and know if it is fit for purpose and privacy compliant. Evaluate data quality based on data profiling results, and get results automatically in a unified catalog view — no queries required.
Integrate risk insights with data governance
Financial organizations need to maintain a laser focus on reducing risk — and implement effective risk-reduction across the enterprise. By accurately identifying at-risk data with BigID — such as overexposed, incomplete, or ungoverned data; redundant, duplicate, derivative, or similar data; data movement violations; permissions violations, and so on — they can enable their teams to initiate remediation workflows and take swift action on data breaches.
Catalog sensitive data across unstructured and structured sources
BigID empowers financial institutions to automatically identify sensitive, personal, and regulated data for a full dynamic catalog view into all their data, everywhere — across structured and unstructured data. Incorporate active metadata for added business context and data-driven insights — and get full oversight into your data, all within a single pane of glass.
Discover Data to Protect Data
Financial institutions face unique hurdles when it comes to effectively protecting their data, achieving regulatory compliance, modernizing their systems, and gaining insights from data.
Start with a single source of data truth to automatically discover, map, and inventory sensitive, regulated, and personal data — across all data sources and types of data. From on-prem to cloud, mainframe to data lakes, structured to unstructured, financial institutions need to take a proactive approach to knowing their data, wherever it lives.
Schedule a demo to see how BigID helps financial services organizations discover, identify, manage, and protect their data.