4 Ways to Mature Your DCAM Capabilities with BigID
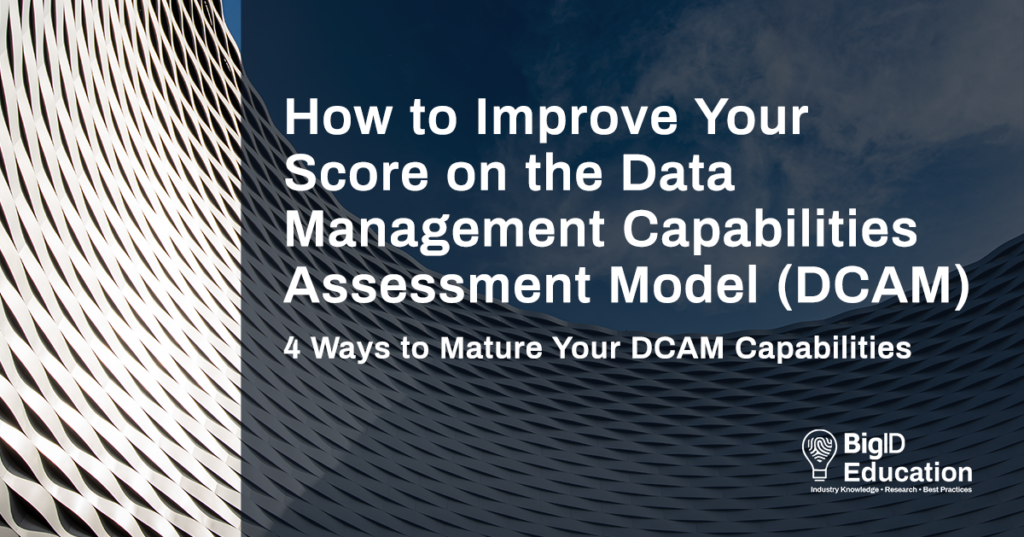
The Data Management Capabilities Assessment Model (DCAM) — established by the EDM Council in 2015 — is an industry-standard framework that more and more companies in Financial Services and beyond are adopting.
Data professionals use the DCAM framework to define, prioritize, organize, measure, and monitor the maturity level of their data governance programs. DCAM addresses the capabilities that organizations need in order to:
- develop the data strategy and business case
- build a sustainable data foundation within an operational framework
- measure consistency and usage of data throughout the organization
- establish stakeholder collaboration and alignment
- integrate the data management program into core aspects of operations
How Is DCAM Scored?
DCAM establishes standards and measures data management capabilities for developing, implementing, and sustaining an effective program. For each capability, a data management program may carry one of six scores:
- not initiated
- conceptual
- developmental
- defined
- achieved
- enhanced
DCAM Challenges: Crossing the Capabilities Chasm
According to the 2020 Global Data Management Benchmark Report, which surveyed finance and non-finance companies on their DCAM scores, 53% of FinServ and 66% of non-FinServ are either “underway/planning” or “not initiated” in the governance and maintenance of authorized data domains, structures, models, definitions, and glossaries.
While many organizations can initiate, conceptualize, and even begin to develop their capabilities by rolling out plans and identifying the right stakeholders to engage in discussions, it’s a taller order to define the engagement, processes, and evidence that need to be established and verified.
The step from “developmental” to “defined” is known as “the capability chasm,” and presents challenges for organizations that include:
- monitoring new business and data models, data inventory, and data sets
- continuously training people and managing turnover
- incorporating data into the business-as-usual practice
- maintaining solid stakeholder support and funding
How AI/ML Closes the Gap on DCAM Capabilities
Analyzing gaps and coming up with a prioritized plan to close them is a critical and time-sensitive concern for data professionals implementing DCAM.
In recent years, the accelerated adoption of artificial intelligence and machine learning capabilities have allowed for faster time to close assessment gaps — plus more efficient fulfillment of audit requirements for regulatory bodies and internal purposes.
Organizations that use machine learning techniques on their data management programs can:
- find patterns of critical data elements faster and more accurately, by leveraging both supervised and unsupervised learning
- automatically scan larger sets of data faster for scaling governance
- apply labels for faster identification and categorization
How To Get Ahead of DCAM with BigID
Here are four key critical capabilities that organizations can execute on to mature their DCAM capabilities score.
1. Automatically Find and Inventory Data
For business and data architecture, data from physical repositories needs to be located, documented, and inventoried. Given the large volume of data centers spread across global offices, data sprawl is not simple to document using traditional discovery and mapping.
Manual documentation and surveying methods are often used to keep physical repositories updated. This method is slow, resource-intensive, and difficult to maintain in a way that accurately reflects the expanding data landscape.
Even after an organization has cataloged its physical data, data professionals still need to understand the underlying content residing in documents and files. This is a growing issue with unstructured data, which is usually excluded from inventories that use manual methods.
BigID leverages advanced machine learning to automatically inventory all data — structured and unstructured — across the entire enterprise. This enables organizations to capture the basic metadata on the data element’s source, field name, and field location — all of which are required for documenting the physical and data architecture.
An AI-driven, automated inventory provides evidence that verifies metadata based on its underlying data content — and makes it accessible to business stakeholders and users in a single catalog.
2. Identify and Map Similar Data Across Columns
Organizations measuring their DCAM capabilities scores can leverage AI/ML to look at large data sets across different data sources, identify patterns, and infer connections.
As data is defined through the identification of logical data domains, models, and metadata, large-scale pattern matching can help to identify duplicate data.
BigID enables organizations to automatically find similar, duplicate, and redundant data with advanced ML. Insight derived from this automated analysis empowers data teams to find and remediate relevant data, identify relationships between data sets, and make recommendations about data that belongs in the same logical data domain.
3. Discover and Tag Critical Data Elements to Ontologies
To close data gaps, organizations need to identify, define, model, and standardize enterprise entities. In order to sustain these activities, data teams must locate and tag all sensitive or critical data in their data sources — and map that data to internal taxonomies and global standards. Since this information typically resides in structured and unstructured sources, automation is critical to effectively classify, label, and document all data at scale.
BigID’s unique data classification capabilities from fuzzy classification to NLP to graph-based technology enable organizations to discover and classify all types of sensitive, critical, and business data — with fewer false positives, improved accuracy, and faster time to insight.
BigID’s next-gen classification optimizes scanning to focus on the data and metadata — and find, tag, and match data. The outcome is improved privacy, sensitivity, consent requirements, and policy monitoring.
4. Detect Anomalies for Data Quality
To meet DCAM capabilities for data quality management, organizations can leverage automation to identify outliers in large data sets. The initial assessment of the data quality profiling prioritizes the scope of the data based on criticality and materiality.
BigID provides statistical analysis results on a data set — such as completeness (or identifying null values), range of data values, and standard deviation of numerical values.
To further sustain the capability maturity level, ML identifies relevant rules to check for the data set — including proposing acceptable tolerance or threshold values and showing trend analysis on data quality. Data teams can demonstrate that they have achieved a higher maturity in data quality management by leveraging BigID to continually monitor new incoming sets of data.
BigID for DCAM
Traditional data management programs rely on manual resources to inventory, label, classify, and define data quality rules — which has led to a general fatigue in waiting for data programs to deliver on business value.
Professionals in data management can leverage BigID’s automated, machine-learning technology to address capabilities measured by DCAM, close data maturity gaps, and ultimately:
- maintain their data inventory
- Identify and classify the data they need to manage and monitor
- map to data domains
- create new rules around data quality management
- Improve their data posture for successful DCAM audits
Learn more about how BigID can help your data management program more effectively discover, classify, and monitor all of your organization’s data — structured and unstructured — and achieve faster and more sustainable results.