What is Data Governance?
Data Governance is the strategy and process of data management in an organization that determines how data will be managed and used throughout the data lifecycle. It refers to the program or rules that define how to govern data. Organizations apply a data governance framework to define how to manage data from collection to deletion, including how to define, access, protect, and share data. A data governance program guides organizations to maximize the value and minimize the risk of data, maintain high data quality, and promote data-driven decisions.
As organizations are becoming more data-driven, there is an increased demand for data needed for analysis and decision-making. While this is a smart move for business outcomes, it means that organizations are experiencing increased data use which comes with an increased interest in making sure that the data is high quality and that all data users have a common understanding of the data so that it is not misused, misinterpreted, or overshared.
Why is it Important?
Organizations generally want to enable data users to have access to the information that they need for business growth and essential operations, but they need to also enforce guidelines to protect data. Data governance is important to maintain compliance with policies and regulations, and be mindful of how to use and protect personal and sensitive data so that they don’t become the next headline for a data breach and lose customer trust.
Data governance challenges
Varied and siloed data
Data exists in various formats and in various sources, often owned by the department that is using or creating it. In some organizations, data lives in business siloes and is managed and governed by those independent siloes. The challenge with siloed data is that the data is managed by different internal policies depending on where it lives in the organization. Without policies that span the siloes to determine how data should be managed, defined, quality checked, accessed, or used, the data is at risk of not being managed consistently. This siloed approach to data management limits the benefit of the data because information would be more valuable if it could be shared across the organization and managed in a consistent way, with understanding so that other departments will find it valuable.
Data sprawl and sharing
Data governance is a challenge also as data sprawls internally and externally. Organizations have deep relationships with customers, vendors, suppliers, distributors, partners, and often data is shared between entities, so data teams need to set the rules for what data can be shared, and how, and with whom.
Remote work and collaboration
Another challenge of managing data is the increase in remote work. Data is everywhere, and now in addition to living in on-prem databases, data lives in our home offices, laptops, phones, and in cloud environments. Data teams need to set the rules about who has access to what data to minimize the risk of overexposure. As teams want to collaborate in a remote environment, understanding who has access to what data and how it is used is a challenge. Many Chief Data Officers (CDOs) building a data culture want to promote data collaboration. Now faced with remote work environments and distributed workforce, it is even more essential to make sure that data is understood and interpreted correctly. Analysis based on incorrect data interpretation could lead to the wrong conclusions and negative business outcomes.
Global Expansion and Regulations
Organizations are expanding globally and facing new challenges with regulations like GDPR, CCPA, and beyond. Data teams need to know what data is sensitive and applies to specific regulations and how to apply policies for compliance. The first step is knowing what data exists in the data environment, and defining what data is considered sensitive and must be protected according to which regulations.
Recent data governance trends and statistics
As data continues to grow in importance and complexity, effective data governance will become even more crucial for organizations. Here are some recent data governance research statistics to consider:
- Increased investment in data governance: Research by Gartner predicts that global spending on data governance will increase by 20% in 2021, reaching a total of $2.1 billion
- Regulatory changes: Data governance is expected to evolve as a result of changes to regulations such as the European Union’s General Data Protection Regulation (GDPR) and the California Consumer Privacy Act (CCPA)
- Data privacy concerns: The importance of data privacy is growing, with 80% of consumers now stating that they are concerned about how their data is being used, according to a survey by IBM
- Challenges with data quality: Research by Experian found that 92% of businesses believe that their customer and prospect data is inaccurate in some way, highlighting the need for effective data governance to improve data quality
- Artificial Intelligence (AI) and Machine Learning (ML): The increasing use of AI and ML in data management and analytics is creating new challenges for data governance, such as ensuring that algorithms are transparent, explainable, and fair
- Adoption of data governance frameworks: Adoption of data governance frameworks, such as DAMA International’s Data Management Body of Knowledge (DMBOK) and the Open Data Governance Framework (ODGF), is growing as organizations seek to establish best practices for data governance
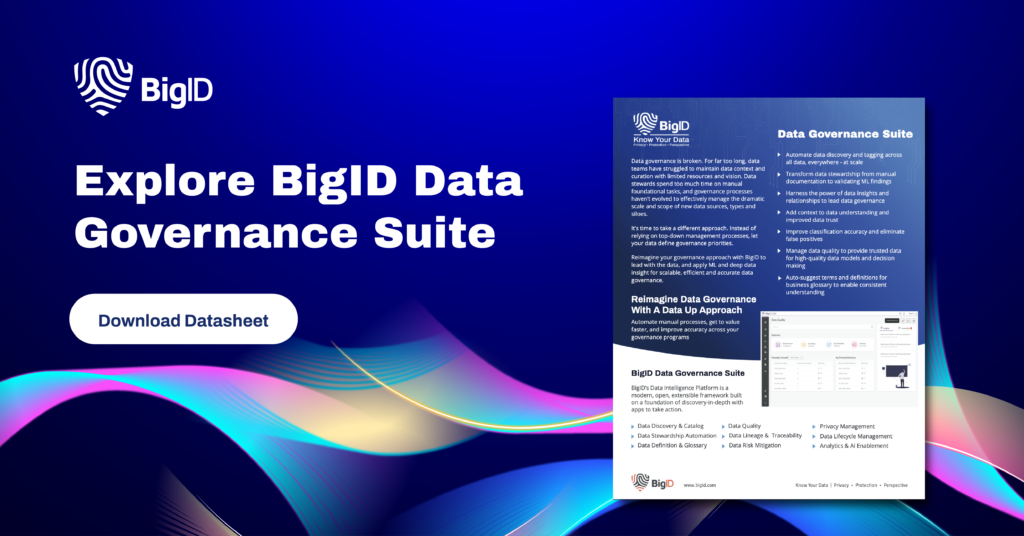
Data governance best practices
Data governance refers to the overall management of data within an organization, including policies, procedures, and controls that are in place to ensure that data is managed effectively, securely, and ethically. These are the best practices for data governance:
- Establish clear data governance policies and procedures: Start by creating a clear set of policies and procedures for managing data within your organization. This should include guidelines for data collection, storage, sharing, and deletion. Make sure that all employees understand and follow these policies
- Assign data ownership: Clearly define who is responsible for managing each dataset within your organization. This will help ensure that data is being managed effectively and that there is accountability for any issues that may arise
- Implement data security controls: Implement a range of security controls to protect sensitive data from unauthorized access or theft. This should include encryption, access controls, and data backups
- Train employees on data governance: Make sure that all employees understand the importance of data governance and are trained on best practices. This includes training on data privacy, security, and compliance
- Conduct regular data audits: Conduct regular audits of your data to ensure that it is accurate, up-to-date, and being managed in accordance with your policies and procedures
- Use a data catalog: Implement a data catalog to help you organize and manage your data assets. This will make it easier to find and use your data effectively
- Monitor data usage: Monitor data usage to ensure that it is being used appropriately and that there are no violations of your policies and procedures
Data governance use cases
Data governance is a critical function in today’s data-driven organizations. It helps ensure that data is managed effectively, securely, and ethically, and can be used to support business objectives. Here are some common use cases and examples of data governance in action:
- Compliance: Data governance is often used to ensure that organizations comply with regulatory requirements, such as data privacy laws like GDPR and CCPA. For example, a healthcare organization may use data governance to ensure that patient data is managed in accordance with HIPAA regulations.
- Data quality: Data governance can also be used to improve data quality. This includes ensuring that data is accurate, complete, and consistent. For example, a financial services company may use data governance to ensure that its customer data is accurate and up-to-date.
- Data security: Data governance can help ensure that sensitive data is protected from unauthorized access or theft. This includes implementing security controls such as encryption, access controls, and data backups. For example, a government agency may use data governance to protect classified information.
- Analytics: Data governance can support data analytics initiatives by ensuring that data is reliable and consistent. This includes establishing standards for data collection and analysis, as well as ensuring that data is properly categorized and labeled. For example, a retail company may use data governance to support its marketing analytics initiatives.
- Data sharing: Data governance can also support data sharing initiatives within and between organizations. This includes establishing policies and procedures for data sharing, as well as ensuring that data is properly secured and protected. For example, a research organization may use data governance to share data with other researchers while ensuring that sensitive data is protected.
- Data management: Finally, data governance can be used to support overall data management efforts. This includes establishing standards and processes for data collection, storage, and deletion. For example, a manufacturing company may use data governance to ensure that its product data is managed effectively throughout the product lifecycle.
Selecting the “right” data governance solution
The scale of data that modern organizations are dealing with is impossible to manage using manual data stewardship alone. Enterprise organizations realize that traditional methods are no longer effective, so data teams are actively seeking modern processes and technology solutions to manage their current data programs. Adopting technology like BigID is critical to get a current and consolidated view of all data to know what needs to be managed, enable dashboards for measurement, and oversee policies and audit enforcement. Using automation and machine learning (ML) is necessary to enable proactive control of the data environment. Deploying the right processes and policies supported by the right technology is the best solution for success.
Streamline Your Data Governance Strategy with BigID
BigID Data Intelligence Platform is a single platform for data governance. With BigID, organizations can scan all of their varied data sources, on-prem or in the cloud, and get a complete unified view of structured and unstructured data to know all of the data in their environment with enhanced metadata in an ml-augmented data catalog.
Where data teams otherwise struggle with manual methods, BigID is able to automatically identify personal and sensitive data, overexposed data, and data that is affected by regulations and policies. Organizations use BigID apps to evaluate data quality, document and share data definitions, and even set retention policies for lifecycle management, including when to remediate expired data or apply policies to retain data for legal holds.
Data teams deploy BigID for data governance to:
- Know what data is in their environment
- Identify sensitive data
- Increase data trust by proactively managing data quality
- Enable understanding with consistent definitions to limit misunderstanding
- Implement privacy policies and regulations for compliance
- Protect personal and sensitive data against misuse
- Minimize the risk of data breaches
- Establish, execute, and audit data lifecycle policies
- Measure, audit, and report data governance results
To learn more about how BigID can help you build a data governance program with automation and machine learning, request a demo to get started.