Reference data enables effective data classification. According to a study by the Enterprise Data Management (EDM) Council, 80% of organizations rely on reference data for data classification efforts. Reference data, such as hierarchies or glossaries, helps organizations categorize and classify data, enabling effective data discovery, analysis, and reporting.
Poor reference data management can lead to data quality issues. Research by Experian Data Quality found that 42% of organizations have experienced data quality issues due to poorly managed reference data. Inaccurate, inconsistent, or outdated reference data can result in data errors, duplication, or misinterpretation, leading to unreliable business insights and decision-making.
What is reference data?
Reference data in the context of data discovery and classification refers to data that serves as a standard or point of comparison for other data. It acts as a benchmark or a reference point against which other data can be evaluated or classified. Reference data typically includes predefined sets of values or codes that are used to categorize, classify, or tag other data elements based on their characteristics or attributes.
For example, in a data classification process, reference data could include a predefined list of sensitive data types such as credit card numbers, social security numbers, or email addresses. When scanning or analyzing data for sensitive information, the reference data is used as a reference point to identify and classify data elements that match the predefined values or patterns. This helps in identifying and categorizing data based on predefined rules or criteria, making the data discovery and classification process more efficient and accurate.
Why is reference data important?
Reference data is crucial for data discovery and classification as it provides a standardized benchmark for identifying, categorizing, and tagging data elements. By using predefined sets of values or codes, reference data serves as a consistent framework that enables accurate and efficient data analysis.
One of the key reasons why reference data is important is that it helps in identifying sensitive or relevant data. For example, in data classification, predefined reference data can include sensitive data types such as credit card numbers, social security numbers, or email addresses. By comparing data elements against this reference data, it becomes easier to identify and classify data that matches the predefined values or patterns, enabling organizations to effectively identify and protect sensitive information.
Reference data also aids in maintaining consistency and accuracy in the data discovery and classification process. It ensures that data is evaluated and classified based on standardized criteria, reducing the risk of subjective or inconsistent classification. This promotes data integrity and reliability, which is critical for making informed decisions about data handling, data protection, and compliance with regulatory requirements.
Types of referential data
Referential data refers to data that provides context or reference points for other data, often used as a standard or benchmark. Different types of referential data include:
- Code sets: These are standardized sets of codes used to categorize or classify data, such as industry codes (e.g., NAICS or SIC codes), geographic codes (e.g., postal codes or country codes), or product codes (e.g., UPC or SKU codes).
- Taxonomies: These are hierarchical or multi-level classifications used to categorize data based on specific criteria or characteristics. Examples include product taxonomies, customer segmentation taxonomies, or risk assessment taxonomies.
- Hierarchies: These are structures that represent relationships between data elements in a hierarchical manner, such as organizational hierarchies (e.g., reporting lines or departments), product hierarchies (e.g., product categories, subcategories, and variants), or customer hierarchies (e.g., parent-company and subsidiary relationships).
- Reference tables: These are lookup tables that store reference data values and their corresponding meanings or descriptions. Examples include currency exchange rates, country or region mappings, or product attribute mappings.
- Glossaries: These are collections of definitions or explanations of terms or concepts used in the organization or industry. Glossaries provide a common understanding of data terminology and help ensure consistent data usage and interpretation.
- Standards: These are established guidelines, specifications, or rules used to ensure consistency, interoperability, and compliance in data exchange or integration. Examples include data standards for data formats, data protocols, or data governance.
- Rules or policies: These are predefined rules or policies that govern data validation, data quality, or data usage. Examples include data validation rules, data retention policies, or data access policies.
Finding context – reference data use cases
- Finance: In the finance industry, reference data can be used to categorize financial instruments such as stocks, bonds, and options, based on their attributes such as asset class, currency, or maturity date. This helps in portfolio management, risk assessment, and regulatory reporting.
- Healthcare: In healthcare, reference data can be used to classify medical diagnoses, procedures, and medications based on industry-standard code sets such as ICD-10, CPT, or RxNorm. This aids in patient care coordination, billing and reimbursement, and medical research.
- Retail: In the retail industry, reference data can be used to categorize products based on attributes such as product type, brand, size, or color. This enables efficient inventory management, pricing, and product categorization for online sales platforms.
- Energy: In the energy sector, reference data can be used to categorize energy sources such as oil, gas, or renewable energy types, based on attributes such as energy density, carbon footprint, or location. This aids in energy trading, environmental reporting, and resource planning.
- Government: In the government sector, reference data can be used to classify citizens, businesses, and government entities based on attributes such as demographic information, tax classification, or business type. This aids in public service delivery, regulatory compliance, and policy making.
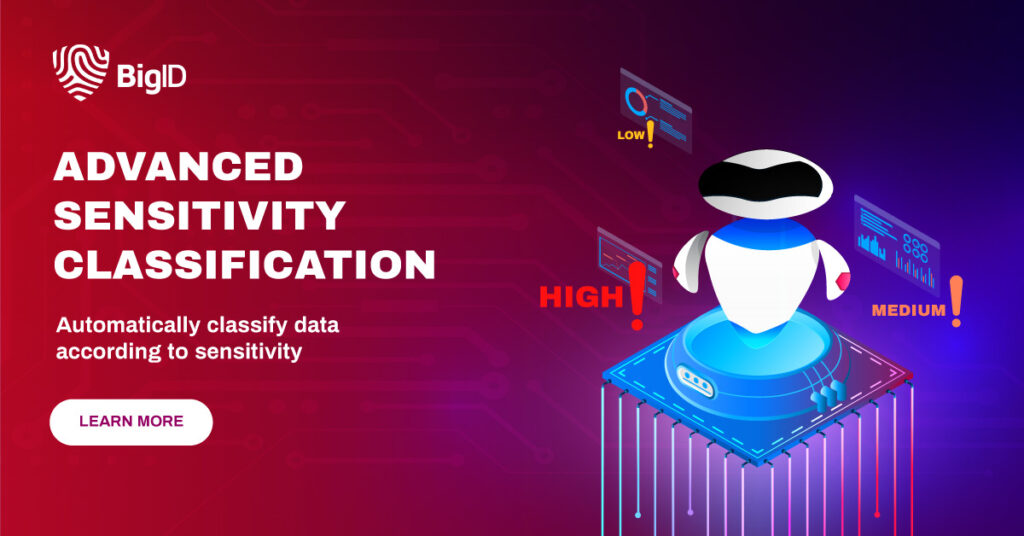
How can organizations manage reference data?
- Establish a centralized reference data management process: Create a structured process for managing reference data centrally, ensuring that it is updated, validated, and securely stored. This process should include data governance practices to maintain data quality and integrity.
- Implement data security measures: Deploy robust security measures to protect reference data from unauthorized access, data breaches, and other security threats. This may include encryption, access controls, and data masking techniques to ensure that sensitive reference data is safeguarded.
- Monitor and audit reference data usage: Regularly monitor and audit the usage of reference data to ensure compliance with data security regulations. This includes tracking who has access to reference data, how it is being used, and identifying any potential risks or vulnerabilities.
- Educate employees on data security best practices: Provide training and education to employees on data security best practices, including the proper handling and usage of reference data. This can help prevent inadvertent data breaches and ensure that employees are aware of their responsibilities in managing reference data securely.
- Automate reference data management processes: Utilize automation tools and technologies to streamline reference data management processes, such as data validation, data enrichment, and data integration. This can help reduce manual errors and improve data accuracy while accelerating business pipelines.
- Regularly review and update reference data: Keep reference data up-to-date by regularly reviewing and updating it based on industry standards, regulatory changes, and business requirements. This ensures that reference data remains accurate and relevant, and helps organizations comply with data security regulations while maintaining business agility.
Potential outcomes of poor reference data management
Poor management of reference data can pose several challenges for businesses. Explore the following:
- Inconsistent and inaccurate data: Poorly managed reference data may result in inconsistencies and inaccuracies, leading to data quality issues. This can impact decision-making, reporting, and analytics, as well as cause operational inefficiencies and errors.
- Lack of data integrity: Reference data serves as a benchmark for data classification and tagging. When reference data is poorly managed, it can lead to data integrity issues, with incorrect or outdated values being used in data analysis or processing, resulting in unreliable outcomes.
- Compliance risks: Reference data is often used to ensure compliance with data security regulations, industry standards, and legal requirements. Poorly managed reference data can lead to compliance risks, such as data breaches, unauthorized access, and data privacy violations, resulting in legal and financial repercussions.
- Inefficient data integration and processing: Reference data is often shared across multiple systems or applications. When poorly managed, it can lead to difficulties in integrating and processing data, resulting in data inconsistencies, duplications, and delays in business processes.
- Increased operational costs: Poor management of reference data may require manual efforts to correct data inconsistencies, validate data, and update reference values. This can result in increased operational costs and resource inefficiencies, impacting the overall productivity and profitability of the business.
- Loss of business opportunities: Inaccurate or inconsistent reference data can lead to missed business opportunities. For example, incorrect product categorization or customer segmentation may result in missed sales or marketing opportunities, leading to revenue losses.
- Reduced customer satisfaction: Poor reference data management can impact customer data accuracy, resulting in incorrect or incomplete customer profiles. This can lead to reduced customer satisfaction, as well as negative impacts on customer relationships and loyalty.
Efficient Reference Data Management with BigID
BigID is a data discovery platform for privacy, security, and governance that helps organizations efficiently manage reference data in several ways:
- Automated data discovery: BigID uses next-gen data discovery techniques to automatically identify, classify, and catalog reference data across various sources, such as databases, file systems, cloud storage, and data lakes. This helps organizations quickly and accurately identify reference data, even in large and complex data environments.
- Centralized reference data management: BigID provides a centralized platform for organizations to manage reference data, including code sets, taxonomies, hierarchies, reference tables, glossaries, standards, and rules or policies. This allows organizations to maintain a single source of truth for reference data, ensuring consistency and accuracy across different systems and processes.
- Data lineage and impact analysis: BigID provides data lineage and impact analysis capabilities that help organizations understand how reference data is used across different data flows and processes. This helps organizations identify dependencies, relationships, and impacts of reference data on other data elements, ensuring proper management and usage of reference data throughout the data lifecycle.
- Data quality and validation: BigID includes data quality and validation capabilities that enable organizations to validate and ensure the accuracy and integrity of reference data. This includes data profiling, data validation rules, data enrichment, and data cleansing features that help organizations maintain high-quality reference data.
- Data governance and compliance: BigID’s Data Governance Suite provides robust data governance and compliance capabilities that help organizations manage reference data in accordance with data security regulations, industry standards, and internal policies. This includes data access controls, data masking, data retention policies, and audit trails that ensure proper data governance and compliance with regulatory requirements.
- Automation and machine learning: BigID leverages automation and machine learning technologies to streamline reference data management processes. This includes automated data discovery, data classification, data lineage mapping, and data quality validation, which helps organizations efficiently manage reference data and accelerate business pipelines.
To more efficiently manage your reference data with automated data discovery—schedule a 1:1 demo with BigID today.