How to Apply AI & ML For Strategic Data Governance Programs
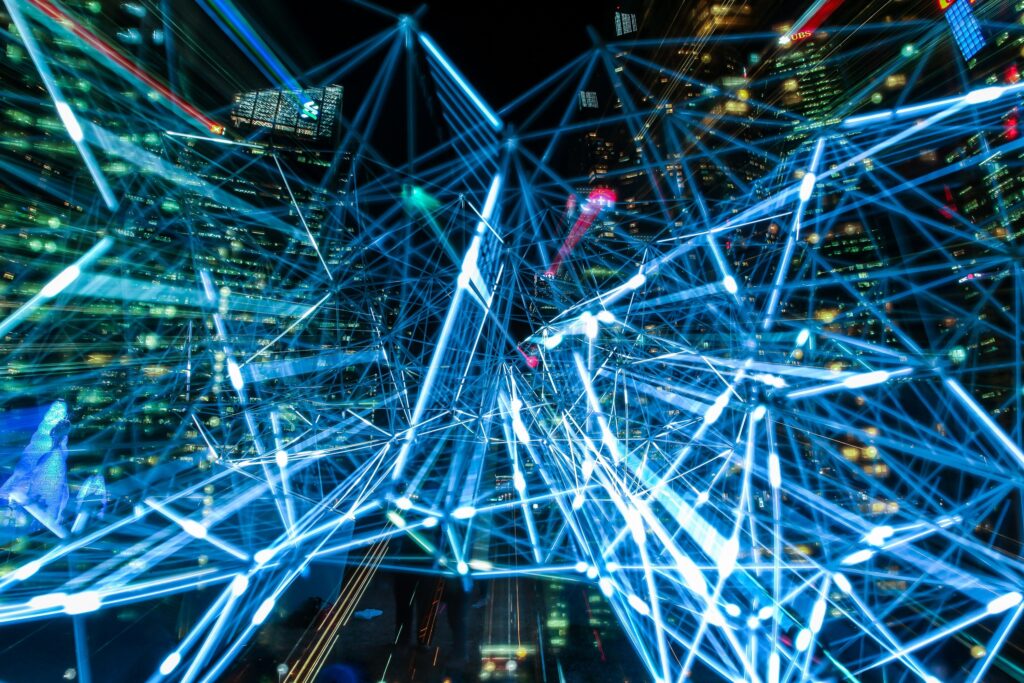
Data leaders around different industries are tackling a common issue: how can they effectively apply artificial intelligence and machine learning when it comes to their data governance programs? Deeper insights, scalability and efficiency are common goals to achieve when looking to implement modern programs and processes in this area. That’s why BigID brought together a group of executives across different industries to have a panel discussion about this specific topic.
In our recent digital summit, Modernizing Data Governance: Leveraging AI and Minimizing Risk, we explored how to address these modern data governance challenges with a panel of data governance experts including Krishna Cheriath, VP of Digital Data and Analytics at Zoetis; Prisca Doe, Advisory data governance lead, at eMoney; and Wendy Turner-Williams, VP of Information Management and Strategy, at Salesforce. Read on for highlights and key takeaways from the panel Leveraging AI/ML to Deliver a Data Governance Program.
Data Governance Programs
When it comes to creating a successful data governance strategy, it greatly depends on the size of the organization. Sometimes data centralization is the best option, while other times a hub and spoke model makes more sense.
In order to empower data custodians and data stewards – and pass the accountability and ownership of the data to them – it’s important for data leaders to drive the shared services and data platforms that allow teams not only to innovate, but to ensure that governance is baked into the Software Development Life Cycle (SDLC) process while making sure that it incorporates trust.
For our panelists, the strategy for building governance programs centers around identifying frameworks, key players and spokes, and working to build trust and partnership with the different teams across the organization to identify what not just their priorities are, but what shared priorities are across the company to drive data management maturity in a faster way.
AI and ML based tools have traditionally been seen as a company-wide solution focused on performing data identification within the organization. Once data is identified and classified, department-specific tools integrate with those solutions to avoid leadership teams imposing on-size-fits-all tools to the business units.
Data Governance Meets Privacy
Today, as more privacy and protection regulations continue to emerge, data leaders must work closely with compliance and legal teams. GDPR was the tip of the spear that is now reinvigorating the data governance community. During the discussion, our panelists highlighted that in this GDPR-first world, ensuring trust means ensuring good metadata management and privacy.
The lines between data governance and privacy teams are blurring. Both teams need to work extremely closely together to monitor, operate and manage data to meet trust requirements in a realistic and proactive manner versus a reactive manner. For legal components to be implemented in the systems across the company, data governance is needed first – if you don’t know where your assets are, you cannot ensure trust.
And talking about blurring lines, data retention often falls between privacy and governance teams.The next generation of data governance is all about data sharing, appropriate roles for data consumption, appropriate metadata, privacy, and other enablers to go along with it. It’s about ethical questions and responsible use of the data, and about protecting the data rights of customers, employees, and other stakeholders. These are not discrete elements anymore, and companies need to think of it as a broader data conversation with many pillars that need to work together.
Quite often, when data is collected at the initial stages of a relationship, there is a lot of focus around consent. However, companies also need to think about the entire data lifecycle as the data ages, and how the use of the data goes from primary to secondary use and how companies maintain fidelity to the original concepts under which the data was collected. Data retention, as well as data consumption through a lifecycle, is a concept of extremely importance within any enterprise.
Data Cataloging and Glossary
It’s clear that data governance teams have a broad scope, and as more elements come under their view, two that remain front and center in data governance programs are data cataloging and business glossary functions.
Understanding where your assets are, cataloging them, and making sure you have the right metadata tagging drives decisions in the right ways, whether your focus is lifecycle and data retention management or data classification. We live in a data world where data velocity and scalability is immense. Days of trying to catalog data from a manual perspective are long gone – it’s not scalable.
There are constant data changes that happen on a daily basis and it’s important that organizations have highly efficient and scalable ML-driven data catalogs that are supporting those changes.
Data catalogs can drive adoption of ML and AI, as data teams see substantial improvements in regards to operational costs reductions to answer questions, along with the increased business agility through data democratization and discovery.
Data catalogs are also being leveraged for risk assessments, security assessments, and compliance vetting. There are a lot of terms that need to be scraped from the source systems and make sure that they are being managed in a standardized and repeatable way. ML is a key aspect to this process in order to make those standard rule engines consistent and scalable.
What’s New in Data Quality
Data quality is definitely not a new topic – but for those of us in the data community, high quality data is one of the ultimate goals. Some might think that data quality is a classic iceberg: there is focus above the waterline and below the waterline. Traditionally, there has been a lot of interest below the waterline; data quality metrics and measurements focused around the management of the data and the quality of the data. But recently, there has been more emphasis on above the waterline: what is the application of the data for a business value context? And what is the quality and veracity of the data for that business use case?
Enterprises need to leverage both above and below the waterline approaches to data quality.
In recent years, data quality has been defined more broadly across different dimensions. It’s about putting the quality measures in context of a business value use case. In this way it can be a much more effective strategy that can guarantee successful conversations with business and technology audiences.
There’s not enough data stewardship or human capital to attack data quality challenges alone: it can only be achieved through a human-machine combination. This means applying AI and ML to tackle 80% of the data quality challenges, while using the highly valuable human capital for the remaining 20%. Companies have to take an ML-first approach in solving these challenges, especially with both the increasing heterogeneity of data, as well as the volume that we all have to deal with.
While there is no single recipe for success, one thing we can take away from our panel of experts is that data governance strategy needs to be tailored to each specific organization. A successful governance strategy must align organizational business objectives and use data governance as an essential tool.
Within data governance, AI and ML can be leveraged to introduce significant innovation. With high-quality data, data teams can support a data-driven culture to speed up innovation within the organization: think of data stewardship, quality and governance as a human-machine combination to strategically scale your governance programs.