Data Governance 2021: Is Your Data Steward Equipped?
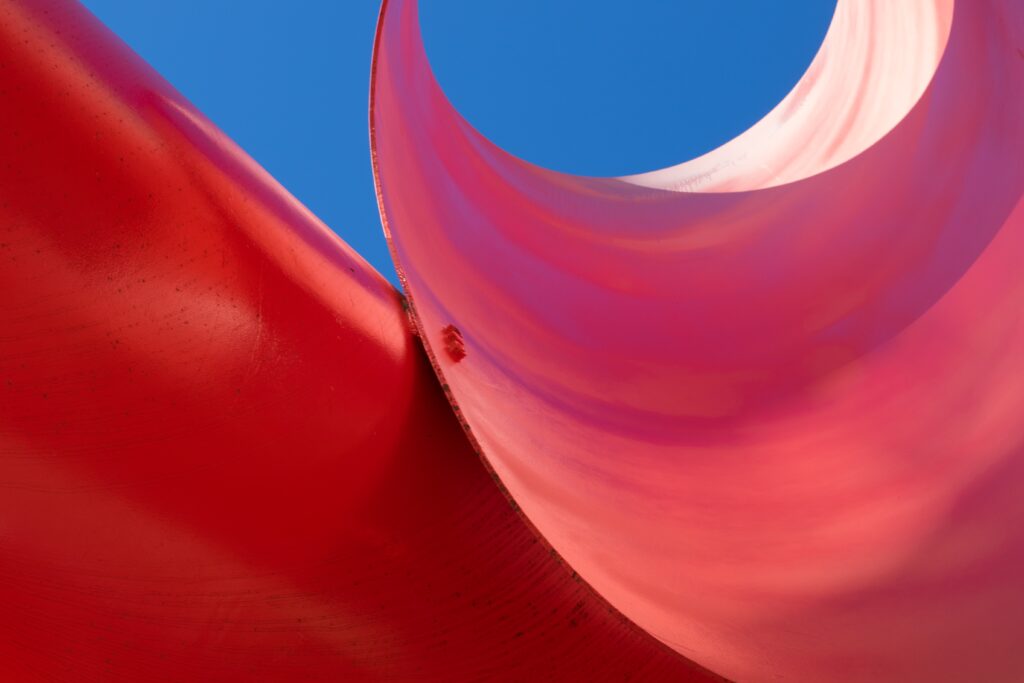
Data stewards serve as gatekeepers to their organization’s data governance programs. These data professionals collect, curate, and standardize data assets across different business units and users — for the purposes of compliance, risk management, and business continuity.
Stewards have traditionally relied on manual processes to gain insights from the data they collect and document — essentially crowd-sourcing knowledge to compile business definitions, identify data sources, and confirm usage patterns.
This top-down approach has given governance programs a reputation. They’re often labeled as policing systems that hinder innovation and agility, which ultimately prevents them from demonstrating business value and gaining broad adoption.
It takes time and resources to scale manual processes to full data coverage — and once data is documented, it immediately becomes stale and unreliable for consumption. Non-data teams resist the slowdown, and executives hesitate to invest in governance programs.
Flipping the Data Stewardship Model Upside-Down
The good news is that enterprises can flip the traditional stewardship model from manual, top-down processes to automated, metadata-driven solutions — and modernize their data governance programs.
By starting with a core discovery platform that automatically finds and identifies data across the enterprise, organizations can tie data assets to meaningful metadata and implement a cohesive, catalog view that empowers data stewards to:
- Uncover context for data usage in a shared catalog
- Reduce time for data consumers to understand impact analysis
- Utilize a single authoritative source for documenting data changes and relationships
- Derive meaningful insights from data and demonstrate business value
Metadata-Driven Capabilities for Data Stewards
By shifting their focus to metadata and data content, data stewards unleash new capabilities they can leverage through automation and machine learning. AI and ML enable organizations to identify, classify, and contextualize data without front-loading their data stewards.
This automated functionality saves data stewards time and allows them to focus on validation over curation. Here are a few BigID capabilities that make this possible:
- Data Discovery: With a deep discovery foundation, find and identify data in multiple systems and applications — including documents and text files that are not normally connected to master data systems. This visibility promotes data citizenship for all business and data users so they can better understand the entire data landscape and answer the ubiquitous question, “Where is my data?”
- Data Profiling: Statistical data profiling promotes data accuracy, completeness, and validity so you can automatically identify data that is fit for purpose — plus calculate and tie data usage to its business application. Data profiling enables large-scale detection of anomalies so you can implement upstream analysis and data remediation workflows.
- Data Access and Entitlements: Manage the data entitlements and access rights of data consumers across the organization — in one single place — and understand the complete string of users for end-to-end data lifecycle management.
- Data Usage: Monitor the consistency of data across all applications, and minimize gaps in the usage and interpretation of the data between data creators and consumers.
- Data Context: Provide transparency, clarity, and meaning into your data — with supporting technical data such as file system owners.
- Data Classification: Use ML-classifiers to identify which metadata, data, and documents to distribute to which data owners — and stay up-to-date with privacy regulations with sensitive, confidential, and personal data classifiers. Label data to support searching, scope selection, and prioritization.
Enable a New Data Governance Model
Whether it’s a technical data steward who needs to manage CRUD (create, read, update, delete) data in building out a new application system, or a business data steward who needs to locate golden copies of data from multiple sources, a metadata-first approach enables data professionals to accelerate and automate their tasks.
With these capabilities, a new data governance model emerges — one that involves scaling and building out data knowledge using machine learning technology. Manual processes of collecting data, mapping it to data sources, and classifying content give way to an innovative approach of automatically validating data, confirming data mappings, and verifying the accuracy of ML-classifiers.
Moving from reactive to active data management allows data stewards to take action faster, make data-driven decisions to support business outcomes, and meet deadlines for regulatory compliance based on complete data.
See how BigID can modernize your data governance programs — and empower data stewards to derive meaningful insights from data and business value.