How to Automate Safe Data Analytics for Financial Services
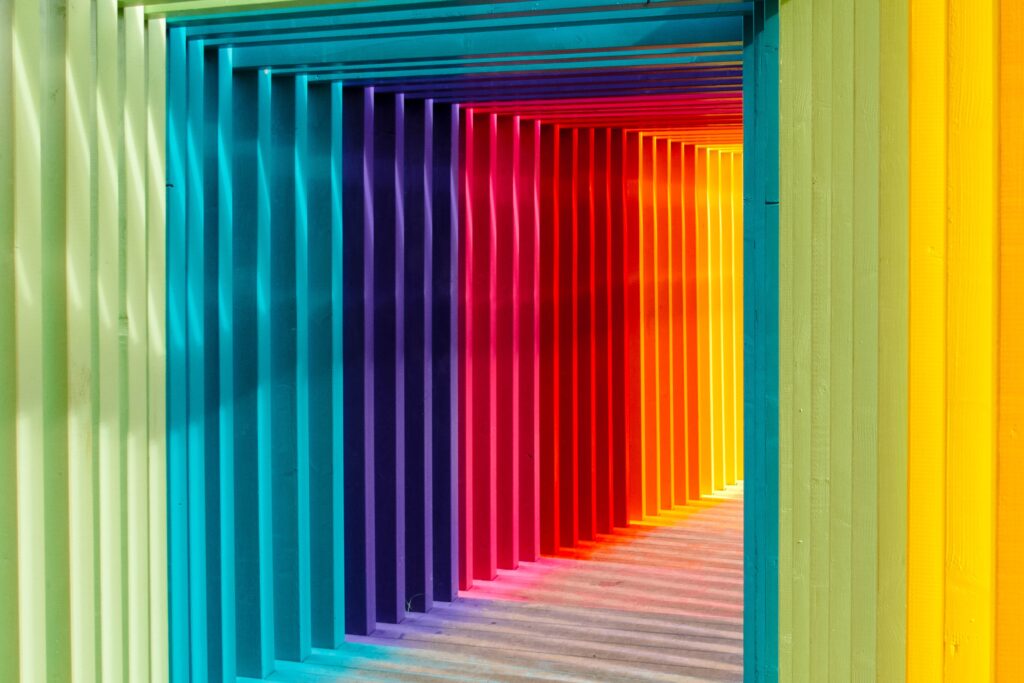
As financial institutions prepare to move more of their data to the cloud, they need to make their systems more agile and scalable while ensuring they have the right approach in place to secure the data and ensure privacy protection.
In “Automating Safe Data Analytics for Financial Services,” BigID’s Technical Director Sachin Khungar sits down with Sebastien Cognet, Privacy Engineer at Privitar, and Ilya Epshteyn, Principal Solutions Architect at Amazon Web Services (AWS). They cover how to automates risk minimization strategies in order to help financial organizations accelerate analytics usage, leveraging services from cloud providers like AWS.
An “Explosion” of Data
Over two million terabytes of new data is generated every day, creating “an explosion in the amount of data that companies are collecting,” says AWS’s Epshteyn.
Another way to think of it: 90% of data worldwide has been generated in just the last two years. Before that, 90% of worldwide data had been generated within the previous five years. Data collection is accelerating at a rapid rate—and companies face the mounting challenge of managing it, protecting it, deriving value from it, and making sure it meets privacy regulations.
This data exists everywhere, in different proverbial shapes and sizes, in various types of data stores. And not all of that data is created equal, from a privacy and security perspective.
“It’s no longer just structured data from mainframes and relational data stores,” says Epshteyn. “Customers are looking to take advantage of semi-structured data—data from social media is an example. Customers are also looking at completely unstructured data, everything from emails to call center recordings.”
Why the sudden proliferation of data? Companies in financial services generate and collect new data for several reasons:
- Compliance and regulatory reporting: The need to comply with regulations (such as GLBA, NYDFS 23 RR 500, FINRA & NY SHIELD) compels companies to create more consolidated audit trails (CAT).
- Finding utility in siloed data: Organizations want to identify new market trends and business opportunities from their data, as well as enhance fraud detection capabilities.
- Enhance customer experience: Interaction data, targeted products, and personalized messages help businesses create better experiences for their customers.
- Risk management: Data collection and use help financial organizations leverage market surveillance, portfolio optimization, and other investment strategies.
It’s not a matter of just migrating data to the cloud. Organizations need to ensure that they’re doing it safely, within compliance standards, and in a way that empowers their analytics teams. Under the shared responsibility model, AWS provides a comprehensive set of controls for user authentication, access authorization, data transport encryption, and auditing, while customers are responsible for taking necessary steps to manage, secure, and govern their data in line with policies and regulations.
BigID: A Powerful Discovery Engine
The key consideration “is around data that needs to be used,” says Khungar. “You want to use some of the sensitive data assets for these analytics and ML-based platforms,” and you also “want the analytics data sets to be used in a safe manner.”
In other words, you need visibility into that sensitive data that allows your teams to understand it—whether it originates from structured, semi-structured, or unstructured sources; whether it’s at rest or in motion; whether it’s on-prem or in the cloud.
BigID’s advanced discovery identifies data sets using AI- and ML-based techniques, and classifies data based on sensitivity and data type—including health-related data, personal data, asset-related data, and so on. “What we come back with is a number of different discovery techniques that we have developed over the years to get to that [advanced] level,” says Khungar. They encompass:
- Classification: Identifies all types of personal and sensitive information across your data sources, including document-level classification and file analysis for unstructured data types.
- Correlation: Value-based discovery across all your enterprise assets. This brings together fragments of information that are specific to an individual—and assigns them to the individual.
- Cluster Analysis: Identifies and groups similar content together. This helps you find duplicates and consolidate assets as you move them into the data pipeline onto cloud platforms.
- Catalog: Assimilates data into an object view of all your assets, with granular data elements about what content exists where, it’s classification and categories, why it’s used, etc.
This process allows organizations to view and manage data through the lens of privacy, protection, and perspective.
This is where BigID and Privitar’s combined technologies come in, enabling organizations to build and automate analytics and machine learning pipelines with privacy protection enforced for sensitive data sets.
Privitar: Security Is Not Enough
The sync-up of BigID’s data discovery and classification with Privitar’s privacy engineering allows organizations to de-identify sensitive data for broader use across the organization. This all happens before the data gets loaded into a pipeline for analytics.
“When we look at all the data breaches that happen in the market, we realize that in 70% of cases, the data is coming from people internal to the company,” says Cognet. “If you deliver a solution only based on access control, it doesn’t work. You are still exposed to data breaches.”
Privitar not only helps organizations protect their data through access control and policy management but also de-identifies data, scrubbing it of certain sensitive and identifiable features, while keeping its utility intact.
This means you can “segment between each data set that should be de-identified. For example, you can work with one partner and another partner and be sure they will never share the data set,” says Cognet. “You will be able to deliver to an analytics team, for example, a data set that is protected, but a data set they can [still] use to do their jobs.”
Smart organizations still need to anticipate potential problems and threats to security, however. “Trust me: for all the companies that experience a data breach, the first question they have is, where is the data coming from?”
Business Benefits: Making Data Safe for Analytics
Through unparalleled discovery that provides a global view into sensitive and personal information across data sources, sophisticated privacy engineering that protects data values with features like watermarking and de-identification, and the automation and safe analytics provided by the world’s most comprehensive and broadly adopted cloud platform, companies are in a good position to derive the most value from their data while ensuring privacy compliance.
This opens up opportunities to broaden data use across your organization, accelerate time to insight, maintain control of your data, and innovate with a modernized system that is agile, scalable, safe, and compliant.
Watch the webinar to learn more about how BigID, Privitar, and AWS can help your company execute data-driven strategies for growth and innovation.