NY Department of Financial Services New Cyber Imperative: Protect Your Customer Data
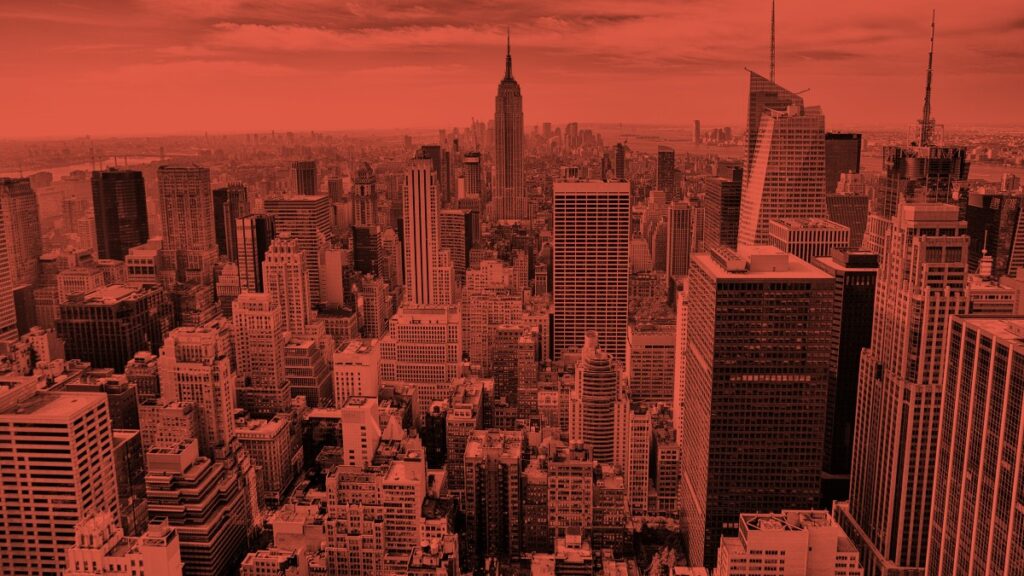
The New York Department of Financial Services (DFS) Cybersecurity Regulation sets new standards for financial services firms doing business in the State of New York to identify and mitigate risk to their business and consumer data. But for all the organizational and technical requirements — like a board-approved cybersecurity program — the real impact of the Regulation will be to force organizations to come to terms with a basic principle: they need first to understand what data they have, whose data it is, where it is in order and ho has access to it to best protect it. And, because the Regulation expands the definition of business and consumer data in scope, a new approach is needed that can learn what data is potentially important and understand data flows.
Information Security Compliance By the Numbers
By its own account, the comprehensive set of information security requirements finalized by the NY DFS on March 1 for 3,000 covered entities is the first of its kind in the US. Certainly, the Regulation breaks with the model underlying many existing compliance mandates of ensuring that basic, “one size fits all” information security policies and procedures are in place. Instead, in line with more recent data privacy and protection mandates like the EU’s General Data Protection Directive, the NY DFS Regulation emphasizes a risk-driven approach to protecting business and consumer information.
As much as the Regulation will impact how organizations invest and manage their information security practices as well as modify their organizational structure to put risk management and mitigation at the center of cybersecurity programs, perhaps the more fundamental shift in focus is what the Regulation sets out to protect.
Under the Regulation’s category of Nonpublic Information, covered entities will not only have to protect personally identifiable financial information as already required under GLBA and healthcare PII under HIPAA, but also any business or consumer data that could have a “material impact” if exposed or stolen by attackers and cybercriminals.
Resolving the Risk Chicken From the Data Egg
The starting point, of necessity, for any of the covered entities will be to assess the risk of unauthorized access or exposure of data that falls under the new Nonpublic Information (NPI) category. Before they can optimize controls to govern who gets access to the data, implement monitoring programs and application security measures as specified by the Regulation, covered entities will have to take a comprehensive inventory of their data and maintain an iterative approach to learning what data could be considered NPI on the basis of its business value and material impact if exposed.
These two novel components of the Regulation — a risk-based approach and scope beyond privacy concerns — make sense when we consider what the overarching intent of the Regulation: to reduce the systemic threat to the integrity of the financial services industry posed by attackers focused on data theft, and stem data breaches that undermine consumer confidence in the system.
By mandating a risk assessment as the point of departure for the cybersecurity program, the Regulation compels covered entities to consider protection in the context of their specific environment, including factors like third party access, rather than go through a checklist to ensure that standard processes and controls are in place. If the objective is reducing the risk of a data breach, allocating resources on the basis of risk mitigation is more likely to have the desired outcome than information security by the numbers — albeit at what will likely be higher levels of investment than currently.
Secondly, even when compared to the EU’s GDPR that also puts risk mitigation front and center, the NY DFS Regulation goes one step further in terms of data coverage scope. If the intent is to limit breaches, not only privacy violations, it follows that the definition of NPI is as broad as it is.
Understand Your Risk to Tackle It
The broader definition of Nonpublic Information incorporated in the Regulation covers not only specific categories of personal identifiers, biometric information, account information, personal access codes and passwords, healthcare information but also any information that if exposed could “cause a material adverse impact to the business, operations or security of the Covered Entity”. The definition also covers data collected in the course of the application process for financial products, which by implication extends the scope beyond the data of existing customers.
Where does this leave covered entities who already struggle to maintain current and comprehensive data inventories and map data flows for the explicitly defined data categories in HIPAA, GLBA and PCI, for example?
By scanning not only structured data sources, but also Big Data repositories, unstructured data sources like file shares and cloud data services, and inferring on the basis of an identifiability score what constitutes Nonpublic Information, covered entities can build a more expansive view of their potential exposure. Without this level of data-driven insight across their infrastructure and the ability to identify and characterize unique attributes through machine learning, covered entities will inevitably define the cybersecurity programs on the basis of an incomplete view of their exposure risk.
This is precisely the challenge that BigID has set out to address: enabling customers to build a complete picture of their data inventory, understand where it lives, iteratively discover new attributes and provide granular insights into how data down to the field level is accessed.
As covered entities look to comply with the Regulation’s requirements, they will need to adopt fresh approaches to discovering and inventorying Nonpublic Information based on data science and machine learning techniques, and structure enforcement such as access controls and targeted data protection based on where their greatest risks lie.
by dimitri sirota & @stavvmc