Generative AI (genAI) is shining the spotlight on unstructured data risk, security, sensitivity and usability like never before. To date, most data quality, integration, governance, and analytics were centered around data formatted in rows and columns in databases, data warehouses, and data lakes. Though organizations have long believed that there is value in mining the unstructured data in files, objects, images, messaging and other productivity applications— nothing has been done to pursue the hidden value. GenAI, with accompanying models and LLMs, is doing for unstructured data what Big Data did for structured data years ago – uncovering hidden value in organizational data.
Organizations are both enthralled and hesitant in adopting genAI for internal and external purposes. Though most concerns are aimed at the genAI prompt and response, there is a larger issue looming in the background. Is targeted data AI-ready, or more precisely is the data appropriate and suitable for training the LLMs that feed the genAI models?
- Appropriateness: Should this data be used in the genAI process?
- Suitability: Is the data germain to the model and are results of responses believable and action worthy?
Appropriateness of data is not universal
Employee data might be fitting for senior HR execs, but not for entry level HR personnel, and it is for sure not appropriate for other departments to access. In the world of unstructured data, sensitive and private information is rife across mostly unmanaged and ungoverned file shares, object storage, email, collaboration tools, and much more. As a Gartner analyst, I took 1000s of calls on managing unstructured data and not once did anyone say, “Wow, we found less sensitive data than we thought.” Quite the opposite, the reply was, “Wow, we are in serious trouble here.”
When it comes to appropriateness of data feeding LLMs, “bad data, bad results” should be a rallying cry for the genAI team. Forrester guides organizations to, Emphasize data discovery, inventory, and classification. Develop policy and implement a process with supporting technologies to discover and classify your organization’s data. To help ensure that you protect and appropriately handle sensitive data throughout its useful lifecycle, understand what constitutes sensitive data for your organization, identify what sensitive data you have, and determine what data environments it exists in. In addition, data classification will help you prioritize critical applications and IT assets. Work toward making data discovery and classification an automated and continuous process, rather than a one-time event. – Forrester (Sandy Carielli, Heidi Shey, et al – High-Performance IT: Security, Privacy, And Resilience – January 15, 2024)
BigID provides an AI enhanced and automated solution for quickly discovering, classifying, and cataloging data while providing security and risk controls to ensure that datasets have been thoroughly interrogated and protected and reach for model consumption.
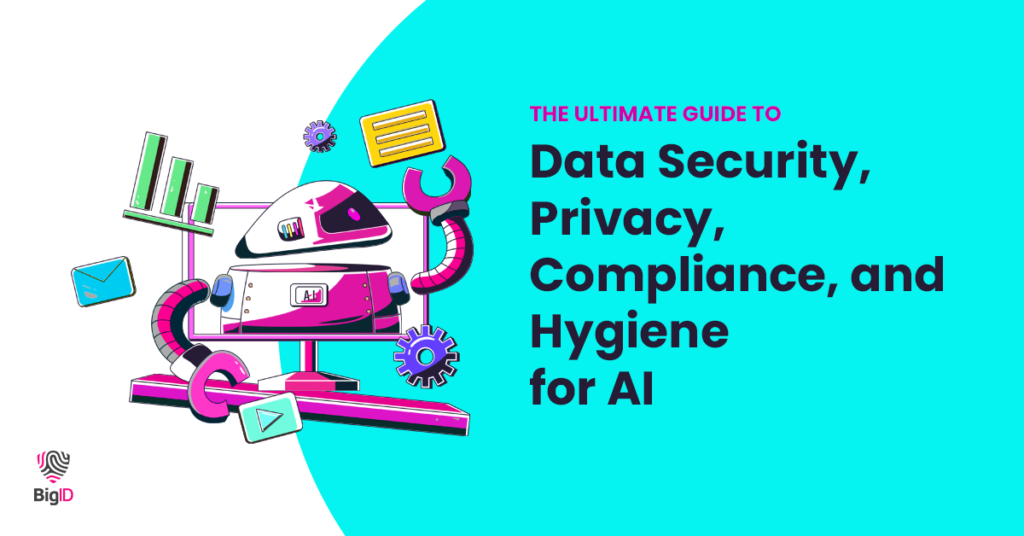
Suitability of data requires a deeper understanding of relationship between the model and the data
When determining suitability of data for genAI, organizations do so in a heavily skewed manner towards specific use cases. Suitability for one use case doesn’t mean it is suitable for all. For example, say I want to build a genAI model to provide a customer-facing bot to assist for support issues. As I look for and scan data sources, support specific information is top of mind. As of today this means that data sets will need to be rescanned for each new purpose. Most unstructured data will remain out of reach to data consumers and unused or unusable until accessibility issues have been addressed. – Gartner® Overcoming Data Quality Risks When Using Semistructured and Unstructured Data for AI/ML Models.)
Most technologies that will help with this are still being developed, with the exception of solutions such as BigID. BigID comes with over 750 OOTB classifiers (additional ones are easy to create) that can identify both metadata and data elements in both unstructured and structured data. BigID uses AI technology to infer additional metadata as well. This, combined with BigID’s identity aware AI and similar document clustering, ensures data sources are AI-ready.
When embarking on a new genAI initiative, it’s just as important to pay attention to the data that is feeding the model, as it is the prompt/response. Early in the process it’s important to look for data sources that are both appropriate and suitable. Appropriate datasets can only be identified via the data discovery, classification, cataloging and de-risking process. Suitable data can be identified at scale only by using solutions like BigID for identity aware AI, similar documents, dissimilar yet related data; and doing this overtime to avoid data drift.
To learn more on how BigID assists organizations by ensuring data is AI-ready and is both appropriate and suitable for genAI — schedule a 1:1 demo with our experts today.
Gartner, Overcoming Data Quality Risks When Using Semistructured and Unstructured Data for AI/ML Models, By Jason Medd, 06 December 2022.
GARTNER is a registered trademark and service mark of Gartner, Inc. and/or its affiliates in the U.S. and internationally and is used herein with permission. All rights reserved.