Data Lifecycle Management: Benefit or Burden?
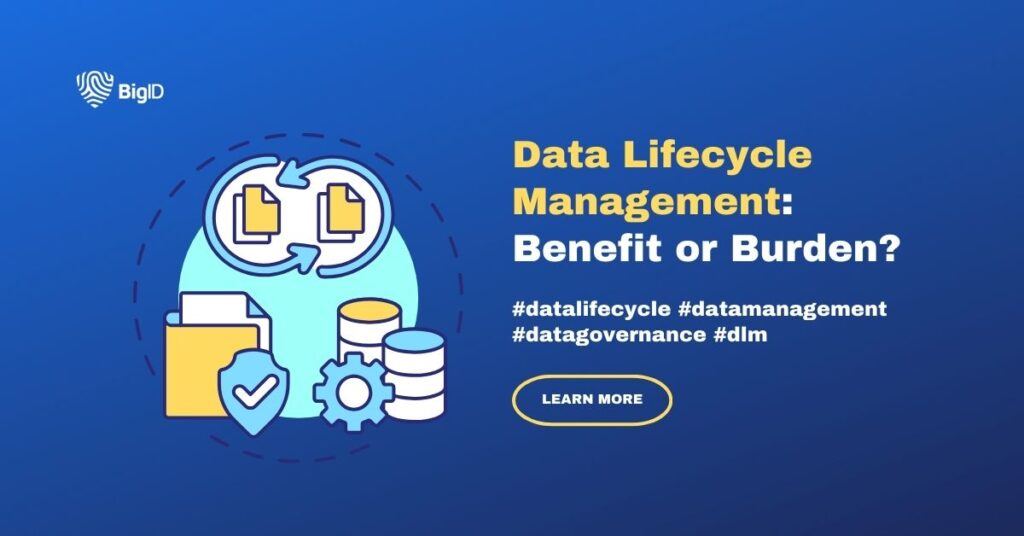
Understanding Data Lifecycle Management: A Complete Guide
In today’s data-driven world, managing data effectively throughout its lifecycle is paramount for businesses aiming to stay competitive and compliant. Data Lifecycle Management (DLM) is the comprehensive approach that guides organizations in handling data from creation to retirement. By understanding the nuances of DLM, businesses can unlock the full potential of their data assets while ensuring regulatory compliance and mitigating risks.
What is Data Lifecycle Management (DLM)?
Data Lifecycle Management (DLM) is the process of managing data from its creation or acquisition to its eventual disposal or archival. It involves organizing, storing, processing, analyzing, and eventually retiring data in a systematic and efficient manner.
Why is DLM Needed?
The need for Data Lifecycle Management arose with the increasing volume, variety, and velocity of data generated by organizations. As businesses began to rely more on digital information for decision-making, they realized the importance of managing data throughout its lifecycle to ensure its integrity, accessibility, and security.
The Evolution of Data Lifecycle Management (DLM)
Over time, Data Lifecycle Management has evolved in response to technological advancements, regulatory requirements, and changing business needs. Initially, DLM focused primarily on data storage and backup strategies to ensure data availability and recovery in case of disasters.
However, as data volumes exploded and regulatory requirements became more stringent, organizations recognized the need for a more comprehensive approach to data management. This led to the development of sophisticated DLM frameworks encompassing data governance, compliance, security, and analytics.
Today, Data Lifecycle Management has evolved into a strategic discipline that enables organizations to extract maximum value from their data assets while ensuring compliance with regulations and safeguarding against security threats. It incorporates advanced technologies such as cloud computing, artificial intelligence, and blockchain to optimize data management processes and drive business outcomes.
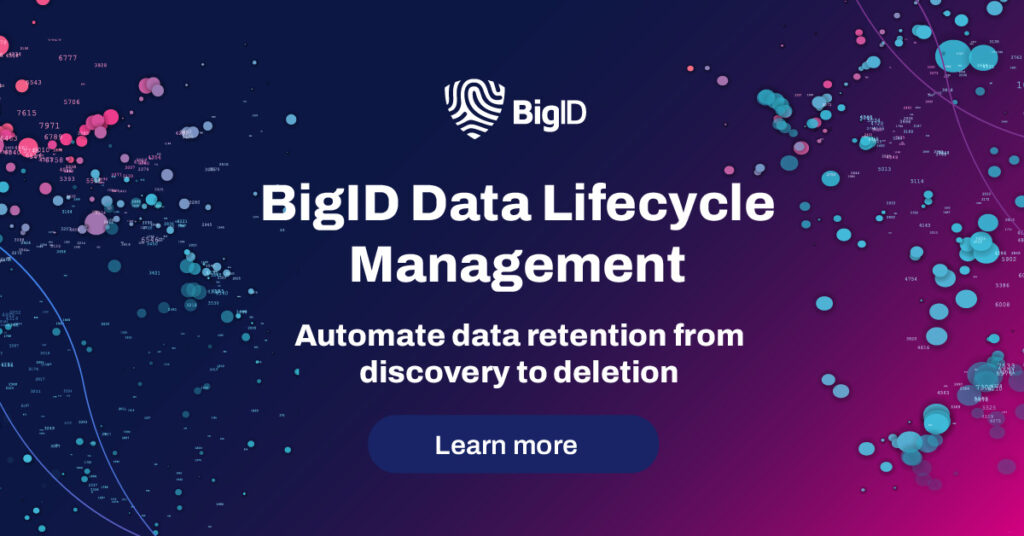
The Importance and Benefits of Data Lifecycle Management
Implementing robust Data Lifecycle Management practices offers several benefits to organizations:
Improved Data Quality
- DLM helps maintain data integrity and accuracy throughout its lifecycle by implementing standardized processes for data collection, storage, and processing.
- By ensuring data quality, organizations can make more informed decisions, reduce errors, and enhance customer satisfaction.
Enhanced Regulatory Compliance
- Compliance with regulatory requirements such as GDPR, CCPA, HIPAA, and SOX is critical for organizations to avoid legal repercussions and protect customer privacy.
- DLM frameworks incorporate policies and procedures to ensure compliance with data protection regulations, including data retention and deletion policies.
Cost Optimization
- Efficient management of data storage and retention reduces unnecessary expenses associated with maintaining redundant or obsolete data.
- By implementing data archival and deletion policies, organizations can optimize storage costs and streamline infrastructure management.
Increased Business Agility
- Access to high-quality, timely data enables organizations to respond quickly to market changes, customer demands, and emerging opportunities.
- DLM facilitates data sharing and collaboration across departments, empowering teams to make data-driven decisions and adapt to evolving business conditions.
Strengthened Data Governance
- DLM provides a framework for establishing clear ownership, accountability, and transparency over data assets.
- By defining roles, responsibilities, and processes for data management, organizations can foster trust and collaboration while minimizing the risk of data misuse or unauthorized access.
Optimized Data Analytics
- DLM facilitates the integration of data from disparate sources and formats, enabling organizations to derive actionable insights and trends from their data assets.
- By leveraging advanced analytics tools and techniques, such as machine learning and predictive modeling, organizations can identify opportunities for innovation, optimization, and growth.
Efficient Resource Utilization
- By automating repetitive data management tasks, such as data cleansing, normalization, and archiving, DLM frees up valuable resources and reduces operational overhead.
- Organizations can reallocate resources to strategic initiatives and innovation projects, driving greater efficiency and competitiveness.
Enhanced Security
- Robust data security measures, including encryption, access controls, and data masking, are essential components of DLM frameworks.
- By implementing proactive security measures throughout the data lifecycle, organizations can mitigate the risk of data breaches, cyberattacks, and insider threats.
Understanding the Data Lifecycle Management Framework
Data Lifecycle Management (DLM) encompasses the processes, policies, and technologies involved in managing data from its inception to disposal. This journey typically comprises several stages:
Data Creation and Acquisition
- This stage involves the generation or acquisition of data from various sources, such as customer interactions, transactions, sensors, or external partners.
- Stakeholders: Data scientists, analysts, IT administrators, and business users are typically involved in data creation and acquisition.
Data Storage and Organization
- Once data is generated, it needs to be stored in a secure and organized manner for easy access and retrieval.
- Best Practice: Utilize a combination of on-premises and cloud storage solutions to balance performance, scalability, and cost-effectiveness.
Data Processing and Analysis
- Data undergoes processing and analysis to derive insights, trends, and patterns that drive decision-making.
- Leveraging advanced analytics tools and techniques, such as machine learning and artificial intelligence, can enhance the value extracted from data.
Data Retention and Archiving
- Not all data retains its value indefinitely. DLM involves determining appropriate retention periods based on regulatory requirements and business needs.
- Compliance regulations, such as GDPR and CCPA, mandate specific data retention and deletion policies to protect individuals’ privacy rights.
Data Disposal and Destruction
- Secure and compliant disposal of data that has reached the end of its lifecycle is crucial to mitigate security risks and ensure regulatory compliance.
- Adopting data sanitization techniques, such as encryption and data masking, prior to disposal safeguards sensitive information.
Common Data Lifecycle Challenges and How to Overcome Them
Despite its benefits, implementing effective Data Lifecycle Management poses several challenges:
Data Silos
Challenge: Disparate systems and departments often lead to data silos, hindering seamless data management and collaboration.
Solution: Implement integrated data management platforms that unify disparate data sources and enable cross-functional collaboration.
Legacy Systems
Challenge: Outdated technology and infrastructure may lack the capabilities needed to support modern DLM practices.
Solution: Gradually modernize legacy systems by adopting cloud-based solutions and leveraging APIs for seamless integration.
Regulatory Complexity
Challenge: Keeping pace with evolving regulatory landscapes requires continuous monitoring and adaptation of DLM policies.
Solution: Invest in compliance management tools and regularly update policies to align with changing regulations.
Data Security Risks
Challenge: Ensuring data security throughout its lifecycle is critical to mitigating the risk of breaches and unauthorized access.
Solution: Implement robust encryption, access controls, and monitoring mechanisms to safeguard data against internal and external threats.
Sample Data Lifecycle Management (DLM) Template
1. Define Data Governance Framework:
- Establish policies and procedures governing data management, including roles, responsibilities, and compliance requirements.
- Define data governance objectives, key performance indicators (KPIs), and metrics for measuring success.
2. Assess Data Needs and Requirements:
- Identify the types of data collected, its sources, and the purposes for which it will be used to inform storage and retention policies.
- Conduct a data inventory and classification exercise to categorize data based on sensitivity, value, and regulatory requirements.
3. Implement Data Classification:
- Classify data based on its sensitivity and regulatory requirements to apply appropriate security measures and retention policies.
- Assign data classification labels or tags to facilitate data management and enforcement of access controls.
4. Deploy Data Management Tools:
- Invest in data management platforms that facilitate data storage, processing, analysis, and compliance monitoring.
- Evaluate and select tools based on scalability, interoperability, security features, and alignment with organizational needs and goals.
5. Establish Data Storage and Retention Policies:
- Define storage requirements, including storage locations, capacity, redundancy, and disaster recovery strategies.
- Establish data retention policies based on regulatory requirements, business needs, and data lifecycle stages (e.g., active, archival, disposal).
6. Implement Data Security Measures:
- Implement encryption, access controls, authentication mechanisms, and monitoring tools to protect data against unauthorized access, theft, and breaches.
- Conduct regular security audits and vulnerability assessments to identify and remediate security gaps and compliance violations.
7. Automate Data Processing and Workflows:
- Automate data processing tasks, such as data cleansing, normalization, and transformation, to improve efficiency and accuracy.
- Implement workflow automation tools to streamline data management processes, reduce manual intervention, and ensure consistency and reliability.
8. Monitor and Audit Data Lifecycle:
- Establish mechanisms for monitoring data usage, access patterns, and compliance with data management policies.
- Conduct regular audits and reviews of data lifecycle processes to identify areas for improvement, address compliance issues, and optimize resource allocation.
9. Provide Ongoing Training and Education:
- Offer training programs and resources to educate employees on data management best practices, security protocols, and compliance requirements.
- Promote a culture of data stewardship and accountability throughout the organization to ensure adherence to DLM policies and procedures.
10. Continuously Evaluate and Improve:
- Regularly assess the effectiveness of DLM practices and technologies in meeting organizational objectives and addressing evolving business needs and regulatory requirements.
- Solicit feedback from stakeholders, benchmark against industry standards, and incorporate lessons learned to refine and enhance the DLM framework over time.
This sample DLM template provides a structured approach to managing data throughout its lifecycle, from creation to disposal, while ensuring compliance, security, and efficiency. Organizations can customize and adapt this template to suit their specific industry, size, and regulatory environment.
The Future of Data Lifecycle Management (DLM)
As biometric data, artificial intelligence (AI), and other emerging technologies continue to reshape industries, they are also revolutionizing Data Lifecycle Management (DLM). Let’s explore the profound impact of these innovations on DLM and how organizations can navigate this evolving landscape to maximize the value of their data assets.
Biometric Data: Redefining Data Collection and Security
As biometric data, artificial intelligence (AI), and other emerging technologies continue to reshape industries, they are also revolutionizing Data Lifecycle Management (DLM). In this article, we explore the profound impact of these innovations on DLM and how organizations can navigate this evolving landscape to maximize the value of their data assets.
- The Rise of Biometric Authentication: Biometric identifiers such as fingerprints, facial recognition, and iris scans are increasingly being used for authentication purposes, revolutionizing how organizations collect and manage sensitive data.
- Challenges in Biometric Data Management: Biometric data presents unique challenges in terms of privacy, security, and regulatory compliance, necessitating robust DLM practices to safeguard against misuse and unauthorized access.
- Implementing Secure Biometric Data Lifecycle Management: Organizations must establish clear policies and procedures for collecting, storing, and processing biometric data, ensuring compliance with regulations such as GDPR and HIPAA while leveraging encryption and access controls to enhance security.
AI and Machine Learning: Optimizing Data Processing and Analysis
- Leveraging AI for Data Insights: AI-powered analytics tools and machine learning algorithms enable organizations to extract actionable insights from vast volumes of data, driving informed decision-making and enhancing business agility.
- Data Quality and Accuracy: AI technologies can improve data quality by automating data cleansing, normalization, and enrichment processes, ensuring that organizations derive maximum value from their data assets.
- Ethical Considerations: As AI becomes increasingly integrated into DLM processes, organizations must address ethical concerns related to bias, transparency, and accountability, emphasizing the importance of responsible AI governance.
Blockchain Technology: Enhancing Data Security and Transparency
- Immutable Data Records: Blockchain technology offers a decentralized and tamper-resistant ledger for recording data transactions, providing enhanced security and transparency throughout the data lifecycle.
- Smart Contracts for Data Governance: Smart contracts enable automated enforcement of data management policies, streamlining compliance processes and reducing the risk of human error or manipulation.
- Integration Challenges: While blockchain holds promise for enhancing data security and integrity, organizations must overcome integration challenges and scalability issues to realize its full potential within DLM frameworks.
Internet of Things (IoT): Managing Data from Edge to Cloud
- Proliferation of IoT Devices: The proliferation of IoT devices generates vast amounts of sensor data, presenting both opportunities and challenges for DLM.
- Edge Computing and Data Processing: Edge computing enables real-time processing and analysis of IoT data at the source, reducing latency and bandwidth requirements while enhancing data privacy and security.
- Data Fusion and Integration: Integrating IoT data with existing enterprise systems requires robust data integration and management capabilities to ensure data consistency, accuracy, and accessibility across the organization.
Biometric data, AI, blockchain, and IoT are just a few examples of the transformative technologies reshaping Data Lifecycle Management. By embracing these innovations and implementing robust DLM practices, organizations can harness the full potential of their data assets while ensuring compliance, security, and agility in an increasingly digital and data-driven world. As technology continues to evolve, organizations must remain adaptable and proactive in navigating the complexities of data lifecycle management to stay ahead of the curve.
BigID’s Approach to Data Lifecycle Management
BigID’s approach to data lifecycle management is designed to provide organizations with a scalable, efficient, and secure way to manage their data throughout its lifecycle. This includes solutions for data discovery, classification, cataloging, and protection, as well as tools for compliance and risk management.
With BigID, businesses can:
- Identify All Data: Discover and classify data to build an inventory, map data flows, and gain visibility on all personal and sensitive information.
- Automate Data Rights Management: Automatically manage privacy requests, preferences, and consent, including opting out of data selling, targeted advertising, and user profiling.
- Data Retention: Apply consistent data retention management policies across all data types and all data sources.
- Minimize Data: Apply data minimization practices by identifying, categorizing, and deleting unnecessary or excessive personal data to efficiently manage the data lifecycle.
- Implement Data Protection Controls: Automate data protection controls to enforce data access and other security measures, which are crucial to safeguarding data.
- Assess Risk: Automate privacy impact assessments, data inventory reports, and remediation workflows to identify and remediate risks to maintain compliance.
To ensure compliance and streamline your data lifecycle management— get a 1:1 demo with BigID today.