6 Steps to Protecting Enterprise Data
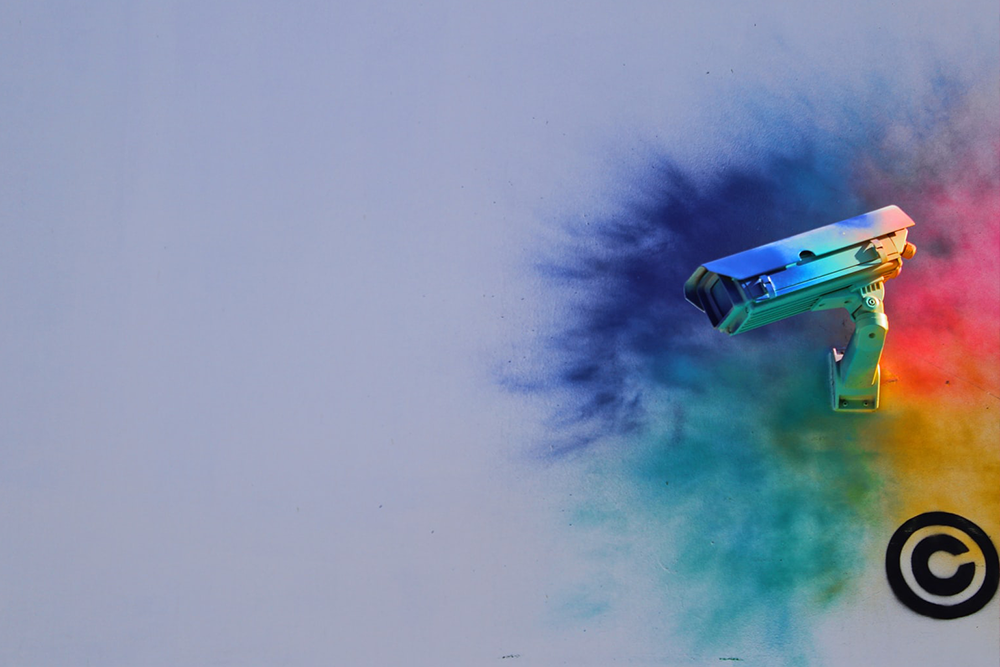
From the dawn of time – or at least the information age, anyways – it’s always been a challenge to manage and protect data. Now more than ever, as data volume explodes and storage types vary, it’s even more of a challenge. Organizations store sensitive information in different types of data formats, across different data stores, and in different ways. Without being able to identify, map, and catalog that personal and sensitive information across an organization’s entire data stores, it’s impossible to take steps to protect that data – the crown jewels of an organization.
The most common type of data targeted in breaches is personal data: in the first six months of 2019 alone, there were over 4.1 billion records compromised from data breaches – and that’s only counting publically reported breaches. That count isn’t even beginning to consider data leaks. Cloud storage like Amazon S3 buckets and overexposed files are notoriously vulnerable to compromise: whether it’s a public university with student social security numbers in a text file, or a national committee with voter records and personal information in open storage.
The majority of sensitive data resides in unstructured data – text documents, excel spreadsheets, emails, pdfs, and image files that store untold volumes of personal, customer, and business data.
Meanwhile, emerging data privacy and protection regulations like the California Consumer Privacy Act (CCPA) are introducing additional penalties and potential legal liability for data breaches, even as they expand the definition of what data needs to be protected.
Organizations of all sizes (and in all industries) need a strong data-centric security policy: start with these six key steps to secure sensitive and personal data.
Step 1: Know Your Data
In order to protect the full scope of your organization’s data, you need to know your data: where it is, whose data it is, and what data is vulnerable.
Traditional data security solutions are often built specifically for one type of data storage or another: it can be difficult to manage, monitor, and protect data in a hybrid environment.
Point tools are siloed, rarely offering a full view of an organization’s data: they may focus on unstructured data in specific on-prem environments, specific types of unstructured data stored in the cloud, or focus purely on unstructured data – leaving organizations unable to build out a full picture of the data that combines insight and mapping on unstructured, semi-structured, and structured data for individual identities, classification types, or entity-based analysis.
Data privacy and security solutions need to be able to cover on-prem, cloud, and hybrid environments. Organizations need to be able to automatically discover and identify sensitive data across their enterprise – regardless of where it’s stored.
Machine learning techniques like cluster analysis are a responsive and scalable way to gain insight into unstructured data, automatically analyzing and inventorying large volumes of unstructured data to identify sensitive and regulated data.
Intelligent data discovery is the first step in protecting enterprise data: you can only protect what you can see.
Step 2: Classify Your Data
Classify your data to effectively drive policy and enforcement. Unstructured, semi-structured, and structured data should be classified for better data management, protection, and processing.
The definitions of what constitutes personal and sensitive information – or different types of regulated information – is expanding: it’s no longer exclusively based on regular expressions, but more often (as we see with the CCPA) identity-based.
This means being able to automatically identify and classify all types of sensitive information based on the content and structure of the data –personal information (PI), personally identifiable information (PII), and sensitive data – without being limited to a specific classifier.
Organizations should be able to automatically classify data by person, type, category, attribute, and more: from names to political activities to geographic data; from regulated data like medical records to financial statements to legal documents; from security attributes like passwords to product keys to encrypted private keys.
Once you’re able to identify and discover all of your data, you can apply labels, tag types of data, and enrich it with additional context to better automate data management and data security.f
Step 3: Correlate Your Data
Data without context is dangerous: it’s essential to establish and map relationships between data in order to not only understand what data you have, but so that you can implement policies to protect it. Correlation builds context around data sets and relationships so that organizations are better able to understand what sensitive data they have, where it lives, and how to protect it.
By leading with correlation, organizations can uncover dark data that’s otherwise vulnerable to compromise – and link that dark data to specific identities, entities, or other sets of sensitive data.
Applying machine learning and neural entity recognition (NER) extends greater insight into data intelligence: data protection solutions need to be able to automatically interpret data in order to create deep data insights.
Step 4: Identify and Manage Risk
A key factor in any security approach is to identify, manage, and reduce risk. CISOs and security teams are now facing increased scrutiny to minimize risk and protect sensitive data – starting with visibility and coverage of data at risk.
Organizations need to align with privacy in order to minimize risk, leveraging advanced data intelligence and insights. Set policies around data movement and compliance in order to monitor data transfer, misuse, and policy violations – in order to better enforce security policies and best practices.
Insights on access intelligence provide greater visibility of data at risk: global access groups represent one of the biggest vulnerabilities in unstructured data. By being able to identify overexposed data, organizations can easily identify high-risk data sources and particularly vulnerable records, with prioritized insights on where to reduce risk.
Risk modeling can help organizations understand and compare data risk based on data sensitivity, residency, data security, and application access – and should be customizable to the specific organization’s industry, type of data, and company profile.
In order to adequately manage and reduce risk, organizations need to take a privacy-centric approach to data protection, following the principles of privacy-by-design, getting 360º visibility of data at risk, and managing a unified inventory of sensitive data across the enterprise.
Step 5: Breach Response & Investigations
Data breaches are no longer an if, but a when.
The most important factor is how well organizations are able to respond to breaches: how to mitigate the fallout, determine the impact, notify those affected, and simplify investigations.
Organizations can minimize the impact of a breach by being able to quickly and accurately determine exactly what – and whose – data was compromised.
Step 6: Get the Most out of Security Investments
When you start with intelligent discovery, next-generation classification, and full visibility on your most sensitive data, you can get more out of existing and future security investments, from DLP enforcement to GRC integrations.
Simplify security orchestration and enforcement by integrating your security policy with DRM, DLP, encryption, tagging, and other point tools – all with a core foundation of understanding what your sensitive data is, where it lives, and what type of security policies should be applied to specific categories of data.
Automatically label and tag files based on existing classification for easier governance and retention management – and align labels with automated workflows for advanced data protection and lifecycle management.
Conclusion: Start with BigID to Protect your Unstructured Data
BigID is the first data protection solution to take a privacy-based approach to protecting sensitive data. BigID discovers, maps, and classifies sensitive data at scale across an organization’s global infrastructure and operations: supporting data-centric security, privacy, and governance programs.
Enterprise-ready and purpose-built to handle today’s volume and breadth of data, BigID supports agile and responsive scale & performance, with unmatched unstructured data coverage including CIFS/SMB; NFS; AWS; Azure; Box; Google Drive; Gmail; Office 365 (OneDrive); SharePoint; and Exchange.
Get broad unstructured coverage in context with other types of data across data centers and in the cloud – and seamlessly integrate data intelligence insights in a single pane of glass.
BigID enables organizations to get visibility and complete coverage of sensitive and high-risk data, uncover dark data, manage risk, automate & enforce security policy, and align a security-by-design approach with privacy-centric security.
Find out more at www.bigid.com/demo – or download 6 Steps to Protecting Enterprise Data: The White Paper.