More than 2.5 quintillion bytes of data are created every day, and most of it never gets deleted. It flows from multiple business units and into numerous systems in the form of structured and unstructured data, and into business applications. Organizations are building data lakes and data warehouses for business intelligence and analytics purposes that contain hundreds of thousands of tables and data elements with thousands of columns. Some are machine generated and others are derived from other base data.
It’s a lot of data, and businesses are actually struggling to keep up. Most large companies don’t actually know all of the data they are collecting across their organization or where it’s all stored. Data is transforming from an asset to liability.
That will have to change, as the stakes have never been higher. New privacy regulations in the EU, California and around the world require organizations to know all the data they’re storing and where it’s located. For example, companies need to be able to find all data belonging to European citizens to comply with GDPR. They need to be able to find information on minors, and to be able to find and delete any individual’s information if requested. How can that be done when you’re sitting on petabytes of data? If you have a breach, how can you know whose data was stolen? How can you properly respect your customers’ privacy if you don’t have a proper accounting of all of their data you are storing?
Traditional data discovery tools don’t help much when it comes to finding PI/PII residing in BigData. They need to stream the data in order to scan it, which isn’t practical given the large amounts of data organizations have now. They can only find identifiable information, like Social Security number or phone number, but not contextual personal information like date of birth. They can’t help with data subject rights; they only tell you what type of data is there (classification) but can’t tell you whose data it is for the purpose of reporting back to individuals or to delete their data. And they have limited support for different data sources. When a data subject needs to be deleted it must be deleted everywhere, whether it’s Hadoop, Snowflake, AWS EMR, SAP HANA, Cassandra or MongoDB Atlas, among other repositories. With the use of AI analytics and multiple input channels, data resurfaces, which means you need to continuously validate deletion.
The BigID solution – Big Data Native, and Entity Centric
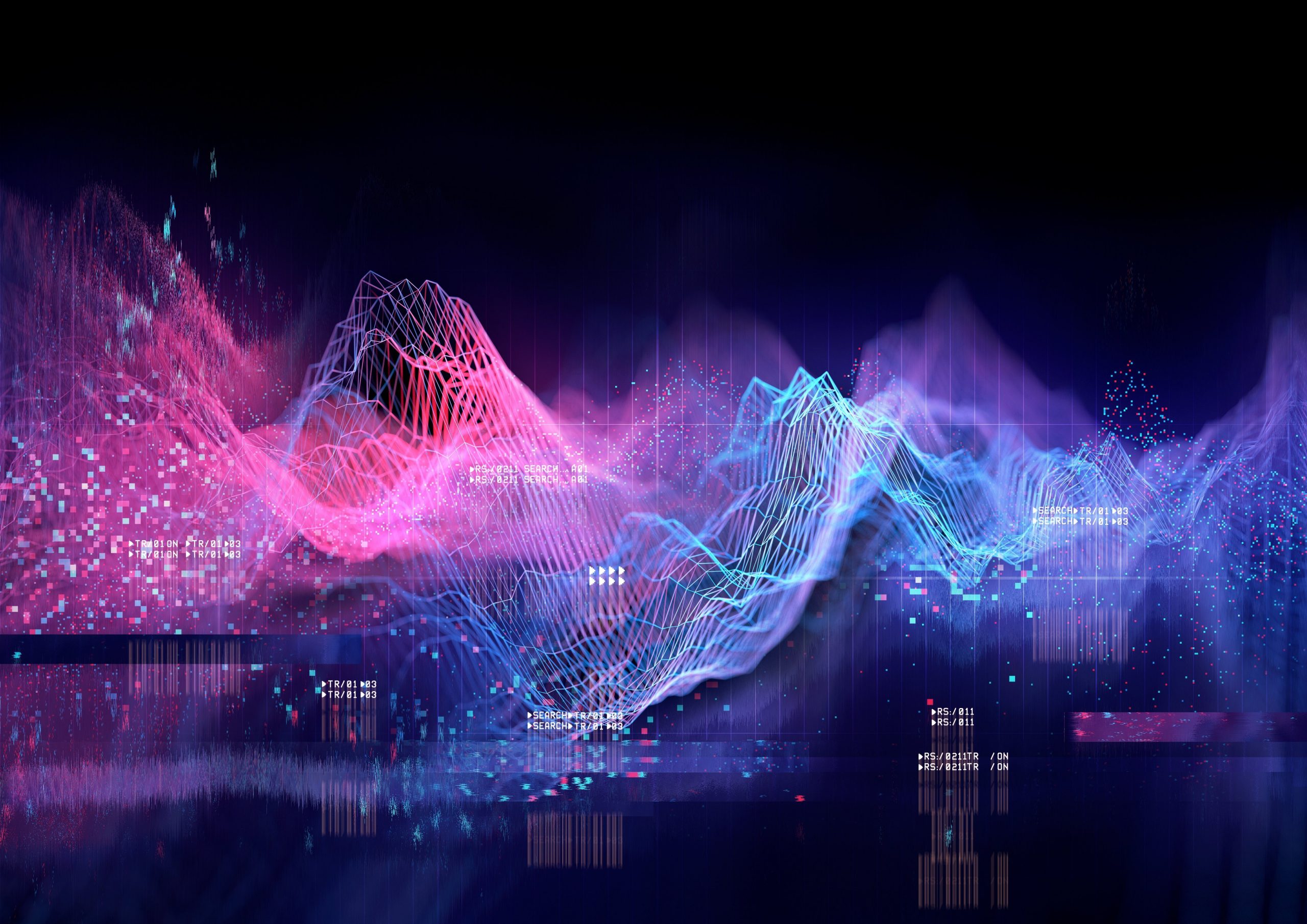
BigID addresses those gaps with the most comprehensive offering, enabling organizations to find and manage all their data, regardless of where it’s stored, what type it is and what format it’s in.
The most comprehensive coverage – Machine Learning-driven discovery and classification of data covers a huge set of big data repositories: Hadoop, Hive, HBase, Snowflake, AWS Redshift, AWS EMR, AWS DynamoDB, Cassandra, CouchBase, MongoDB, SAP HANA, ElasticSearch, and Redis. Beyond big data, BigID supports unstructured files in Windows shares, Exchange, Gdrive, Box, AWS S3, Azure Storage, NetApp and EMC, among others. And all the major business applications are supported. While business applications contribute data to and consume data from big data repositories, BigID can scan those systems to provide a holistic umbrella to the data. BigID has integrations with Collibra, ASG, SAP, Salesforce’s SFDC, Microsoft, Ionic, Immuta, ServiceNow, NetSuite, Workday, Zendesk, Jira, ServiceNow, SurveyMonkey, and others.
Big Data Native – BigID offers flexibility, able to operate in different environments. It runs natively in big data environments like MapReduce, or as user-defined functions in data warehouses leveraging their parallel processing capabilities to run large-scale scans without having to stream the data out of the data warehouse. Cloud-native architecture allows the deployment of BigID in any Kubernetes environment with automated lateral scaling that supports hybrid deployments for both on premise and in the cloud. Smart AI-augmented sampling provides accurate results by auditing the petabytes of data leveraging AI to reduce false positives and false negatives, and providing data quality indicators that help manage data quality at scale.
Entity Centric Correlation – ML-driven identity correlation offers the ability to find all the data for a specific individual across all data sources at extremely high and measurable accuracy. This enables the operationalization and automation of data subject requests. Compliance features continuously validate and send alerts when data of an individual who asked to have it deleted resurfaces and data owners are notified of new data sets when they are discovered. BigID enforces consent and validates that individuals whose data was found in the data warehouse actually provided their consent.
Additionally, if there is a breach, BigID can tell you whose data was impacted.
AI-augmented PI discovery – Organizations can quickly and easily find the exact data they are looking for. Smart AI-augmented sampling provides accurate results by auditing the petabytes of data. ML Driven discovery enables the discovery of any data correlated to an individual, not only data by itself being sensitive. This allows finding all of a user’s transactions, running routes, date of birth, Gender, Religion, Etc.
Organizations can’t afford to overlook any of their data given today’s regulatory environment. They need to understand exactly what data they have and they need to use a comprehensive and integrated approach to do that. With BigID they are able to comply with regulations, protect their data and be better privacy stewards.