Advanced Threat Detection: Leveraging AI and Machine Learning for Proactive Security
In today’s digital age, the amount of data generated and stored by organizations is staggering. This data includes everything from customer information and financial records to proprietary business strategies. Protecting this sensitive data from threats is crucial, yet increasingly complex. This is where advanced threat detection, powered by artificial intelligence (AI) and machine learning (ML), comes into play. But what exactly does this mean, and how does it help protect sensitive data both on-premises and in the cloud?
Understanding Advanced Threat Detection
Advanced threat detection refers to the use of sophisticated tools and technologies to identify potential security threats before they can cause significant damage. Traditional security methods, like firewalls and antivirus software, are often reactive—they respond to threats after they’ve been identified. In contrast, advanced threat detection uses AI and ML to predict and identify threats proactively.
AI and ML in Threat Detection
- Artificial Intelligence (AI): AI can mimic human intelligence to perform tasks such as analyzing vast amounts of data, identifying patterns, and making decisions based on that analysis.
- Machine Learning (ML): ML is a subset of AI that enables systems to learn from data, identify patterns, and improve their performance over time without being explicitly programmed.
How Advanced Threat Detection Works
Advanced Threat Detection leverages AI and ML to protect data by continuously monitoring for and identifying potential security threats. Here’s a breakdown of how it works:
Data Collection and Analysis
- Continuous Monitoring: AI systems gather and analyze data from various sources, such as network traffic, user behavior, and system logs.
- Anomaly Detection: Machine learning algorithms identify patterns and detect anomalies that may indicate a threat.
Behavioral Analysis
- Baseline Establishment: ML models learn the normal behavior patterns of users and systems.
- Deviation Detection: Any significant deviation from these patterns triggers alerts for potential threats.
Predictive Analytics
- Historical Data Examination: AI analyzes historical data to predict future threats and vulnerabilities.
- Threat Forecasting: This helps in fortifying defenses against anticipated attacks.
Automated Responses
- Immediate Action: Upon detecting a threat, the system can automatically isolate affected areas, block malicious activities, and notify security teams.
- Remediation: AI tools assist in analyzing and addressing the threat, including applying patches and restoring systems to a safe state.
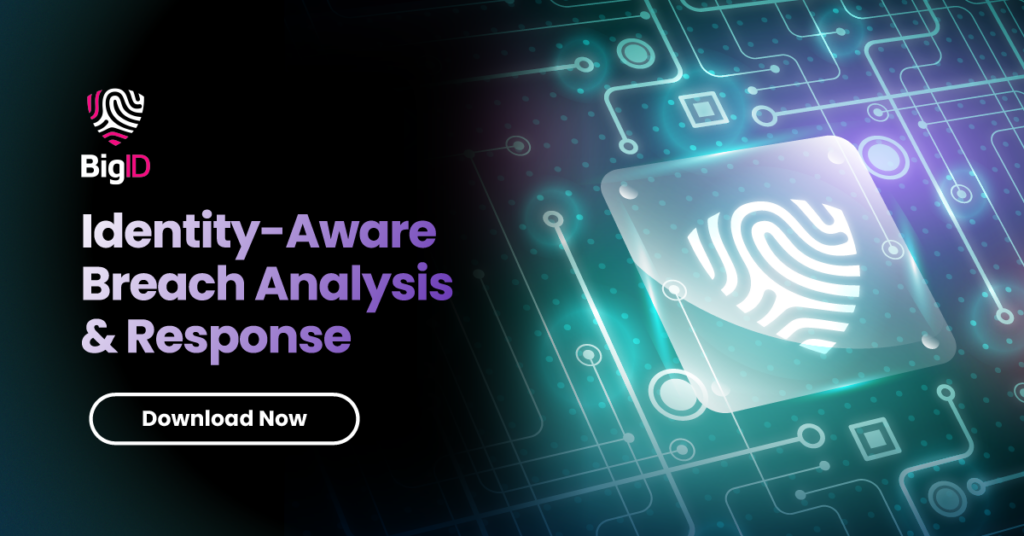
Benefits of Advanced Threat Detection
- Enhanced Security: Continuous monitoring and real-time threat detection provide a robust defense against both external and internal threats.
- Proactive Risk Management: Early detection of anomalies and predictive analytics help organizations anticipate and mitigate risks before they escalate.
- Regulatory Compliance: Advanced threat detection aids in complying with stringent industry regulations by ensuring data integrity and security.
- Customer Confidence: Protecting sensitive financial and personal data enhances customer trust and loyalty.
- Operational Efficiency: Automation of threat detection and response processes reduces the workload on security teams, allowing them to focus on more strategic tasks.
By implementing advanced threat detection, financial services and insurance companies can safeguard their operations, protect their customers, and maintain a competitive edge in an increasingly digital and threat-prone landscape.
Advanced Threat Detection Example – Financial Services
Scenario: Preventing Fraudulent Transactions
A large multinational bank implements advanced threat detection to protect its vast network of financial transactions. The bank processes millions of transactions daily, making it a prime target for fraudsters.
Implementation:
- Continuous Monitoring: The bank’s advanced threat detection system continuously monitors transaction data in real-time.
- Behavioral Analysis: Machine learning models analyze customer transaction patterns to establish a baseline of normal behavior.
- Anomaly Detection: Any deviation from this baseline, such as an unusual large transfer from a normally low-activity account, triggers an alert.
- Automated Response: The system automatically flags the suspicious transaction, temporarily halts it, and notifies the fraud investigation team for further review.
Benefits:
- Early Fraud Detection: By identifying anomalies early, the bank can prevent fraudulent transactions before they are completed.
- Reduced Financial Loss: Quick response to potential fraud reduces the financial impact on the bank and its customers.
- Enhanced Customer Trust: Customers feel more secure knowing their financial transactions are continuously monitored for suspicious activity.
By implementing advanced threat detection, financial services can safeguard their operations, protect their customers, and maintain a competitive edge in an increasingly digital and threat-prone landscape.
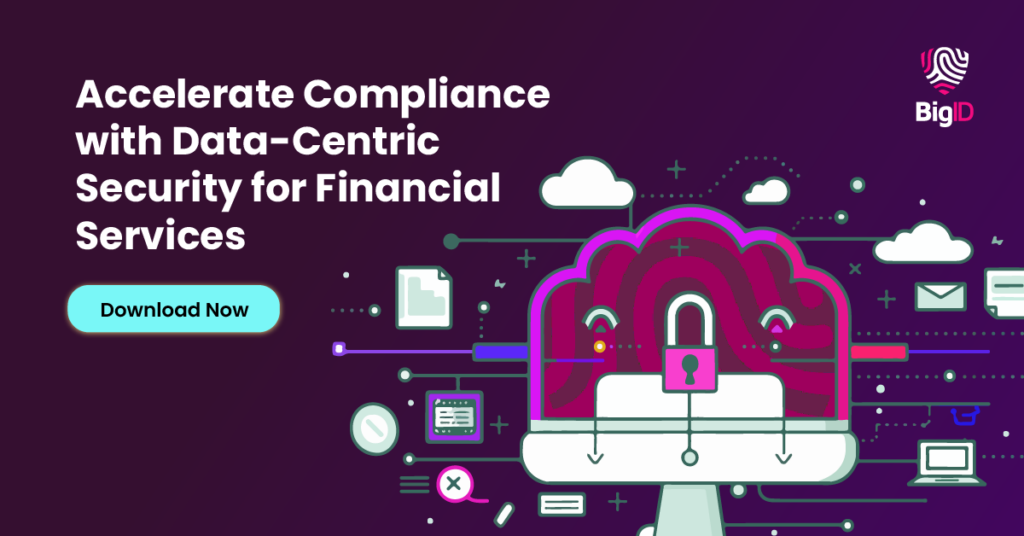
Selecting the Right Threat Detection Software
When choosing advanced threat detection software, consider the following key features:
- Real-Time Monitoring: The ability to continuously monitor systems and networks in real-time is crucial for early threat detection.
- AI and ML Capabilities: Ensure the software uses robust AI and ML algorithms for accurate anomaly detection and predictive analytics.
- Scalability: The software should scale easily to accommodate growing data volumes and increased network traffic, especially in cloud environments.
- Integration: Seamless integration with existing security infrastructure and other tools (e.g., SIEM, endpoint security) is vital for a comprehensive security strategy.
- Automated Response and Remediation: Look for software that can not only detect threats but also automate response actions and remediation processes to minimize damage and recovery time.
- User-Friendly Interface: An intuitive interface that provides clear insights and actionable alerts can help security teams respond more effectively.
- Vendor Reputation and Support: Choose software from a reputable vendor that offers reliable customer support and regular updates to keep up with evolving threats.
By focusing on these features, organizations can select advanced threat detection software that effectively protects their sensitive data against modern cyber threats.
Why AI and ML are Game-Changers
The traditional approach to threat detection often falls short because it relies on predefined rules and signatures to identify threats. This method can miss new, unknown threats and is often too slow to respond to rapidly evolving cyberattacks. AI and ML, on the other hand, can analyze huge datasets in real-time, detect anomalies, and adapt to new threats quickly.
On-Premises vs. Cloud Environments
- On-Premises: Data is stored on physical servers within an organization’s facilities. The organization has complete control over its security but must also bear the full responsibility of managing and updating security measures.
- Cloud: Data is stored on remote servers managed by third-party cloud service providers. While the provider ensures some level of security, the organization must implement additional measures to protect sensitive data.
Proactive Threat Detection
Proactive Measures:
- Anomaly Detection: AI and ML systems continuously monitor data traffic and user behavior to identify any anomalies. For instance, if an employee’s account suddenly starts downloading large amounts of data at odd hours, it could indicate a potential breach.
- Behavioral Analysis: ML models learn normal behavior patterns within the organization. Any deviation from these patterns is flagged for further investigation.
- Predictive Analytics: By analyzing historical data, AI systems can predict potential future threats and vulnerabilities, allowing organizations to fortify their defenses in advance.
Reactive Measures and Remediation
Despite the best proactive measures, some threats may still penetrate defenses. This is where reactive measures and remediation come in.
Reactive Measures:
- Incident Response: Once a threat is detected, an incident response plan is activated. This involves identifying the threat, containing it, and eradicating it from the system.
- Forensic Analysis: AI tools help in analyzing the attack to understand how it happened, what data was affected, and how to prevent similar attacks in the future.
- Automated Remediation: AI systems can automate the remediation process, such as isolating affected systems, applying patches, or rolling back changes made by the attacker.
Implications for Sensitive Data
On-Premises:
- Organizations have full control over their data security infrastructure.
- They can implement customized AI and ML solutions tailored to their specific needs.
However, they must also manage all hardware, software, and security updates, which can be resource-intensive.
Cloud:
- Cloud providers offer built-in security features and updates.
- AI and ML solutions can be scaled easily to handle large datasets and increased traffic.
- Shared responsibility model: While cloud providers ensure the security of the cloud, organizations must secure the data within it, necessitating additional layers of protection.
BigID’s Approach to Threat Detection
Today’s organizations are at greater risk for data breaches than ever before. Protecting against cyber threats starts with knowing what data you have, where it lives, and who has access to it— all information BigID can help discover with its industry leading platform for data privacy, security, compliance, and AI data management.
With BigID business can:
- Identify All Data: Discover and classify data to build an inventory, map data flows, and gain visibility on all personal and sensitive information.
- Minimize Data: Apply data minimization practices by identifying, categorizing, and deleting unnecessary or excessive personal data to efficiently manage the data lifecycle.
- Implement Data Protection Controls: Automate data protection controls to enforce data access and other security measures, which are crucial to safeguarding data and complying with various regulations.
- Assess Risk: Automate privacy impact assessments, data inventory reports, and remediation workflows to identify and remediate risks to maintain compliance.
You can’t protect what you don’t know. To make sure your organization is fully prepared to face impending cyber threats— book a 1:1 demo with our security experts today.