AI Security Framework: Reduce Risks & Leverage Benefits With Artificial Intelligence
Artificial intelligence (AI) is helping organizations, including those in healthcare, finance, transportation and many more. However, as AI tools take center stage, securing them should be given the same spotlight.
Building trust and reliability around AI applications is the first step for fostering acceptance and unlocking its full potential. In this blog, we’ll explore the risks, implementation, governance, and benefits of streamlining workflows for AI security compliance.
What is Artificial Intelligence Security?
AI platforms face potential threats and risks that could compromise their integrity, confidentiality, availability, and reliability. AI Security refers to the measures and techniques used to protect these systems from those weaknesses.
Creating secure software involves implementing safeguards and countermeasures so that AI systems are resilient to attacks, misuse, or unintended consequences related to AI cybersecurity.
AI Data Security Risks & Concerns
As AI technologies become integral to software development and security solutions, they also introduce new dangers. Their rapid adoption across various use cases demands adherence to rigorous security standards (such as those proposed by the EU AI Act) for AI systems to be safe and secure.
Security analysts and cybersecurity teams are crucial in implementing security best practices to mitigate these risks. To address the data security challenges associated with AI, they must balance leveraging its potential while maintaining robust security measures to safeguard against emerging threats.
Some of the most common concerns include:
Data Privacy and Confidentiality
The vulnerability of sensitive data within AI systems is a pressing concern, given the potential for data breaches and unauthorized access. As AI relies heavily on large datasets for training, the security of this data becomes pivotal.
Adversarial Attacks
These threats are designed to manipulate or deceive AI, often with malicious intent. To understand the scope of adversarial threats, you must explore real-world examples that underscore the implications of such attacks. It’s important to recognize and address adversarial weaknesses to fortify AI frameworks against intentional subversion.
Bias and Fairness
Biased training data can significantly impact the AI model’s outcomes. It leads to unfair or discriminatory results. To tackle this concern, you need a nuanced understanding of how AI algorithms may inadvertently perpetuate biases. Strategies to address fairness concerns in these algorithms must be implemented to ensure equitable and unbiased outcomes.
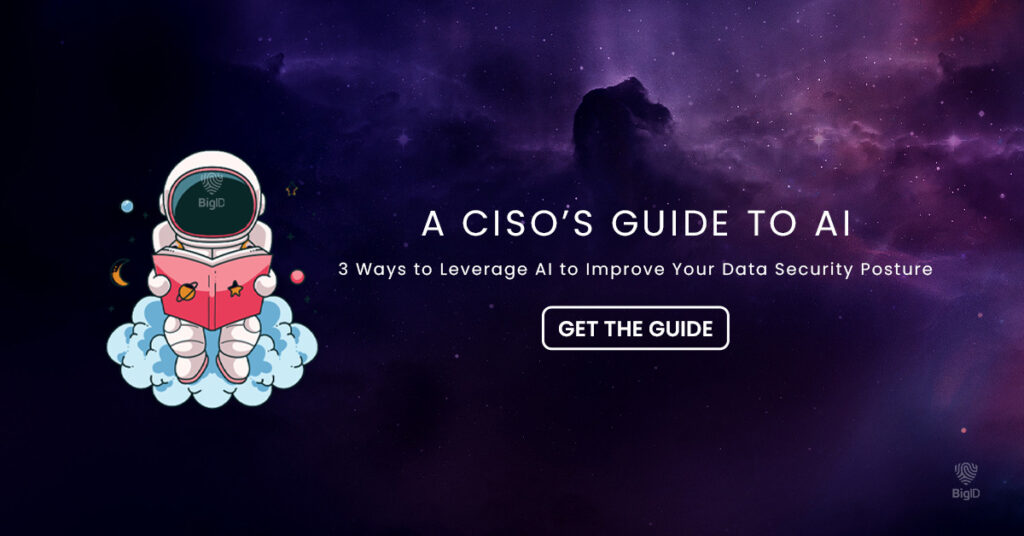
Best Practices for a Secure AI Framework
Here are key considerations for building secure AI processes against potential threats:
- Robust Model Architecture: To build resilient AI models, you need security from the ground up. You can strengthen its security posture by implementing defense mechanisms against potential attacks at the development stage, starting with the training dataset.
- Encryption and Secure Communication: Data transmission within AI systems has to be secure. Encryption is pivotal in safeguarding communication within AI software by preventing unauthorized access to sensitive data.
- Continuous Monitoring and Auditing: You can detect anomalies or suspicious activities within AI systems through real-time monitoring. Regular audits provide a systematic approach to assess and enhance overall AI infrastructure security. They also provide a proactive stance against potential exposures.
Key Components and Benefits of Security Automation
- Threat Detection and Response Automation: Imagine having an AI sentinel tirelessly scanning the digital horizon for security vulnerabilities. Automated security helps identify potential threats the moment they emerge.
- Automated Incident Response: Swift and automated actions kick in to minimize downtime, giving you a proactive defense that’s faster than the blink of an eye.
- Continuous Vulnerability Assessment: This means proactive identification of weaknesses — no stone is left unturned as automated systems tirelessly seek out and identify weaknesses before they become entry points for cyber threats.
- Automated Remediation: When a potential risk is detected, automated processes spring into action, which speeds up threat mitigation and provides a robust shield against potential breaches.
- Scalability and Resource Efficiency: As your business expands, you need your security to scale up with it. Automation keeps your AI infrastructure protected within security protocols.
- Optimizing Resource Allocation: Automation through AI can enhance security measures efficiently. It makes smart decisions about resource allocation to ensure your defenses are strong without unnecessary overhead.
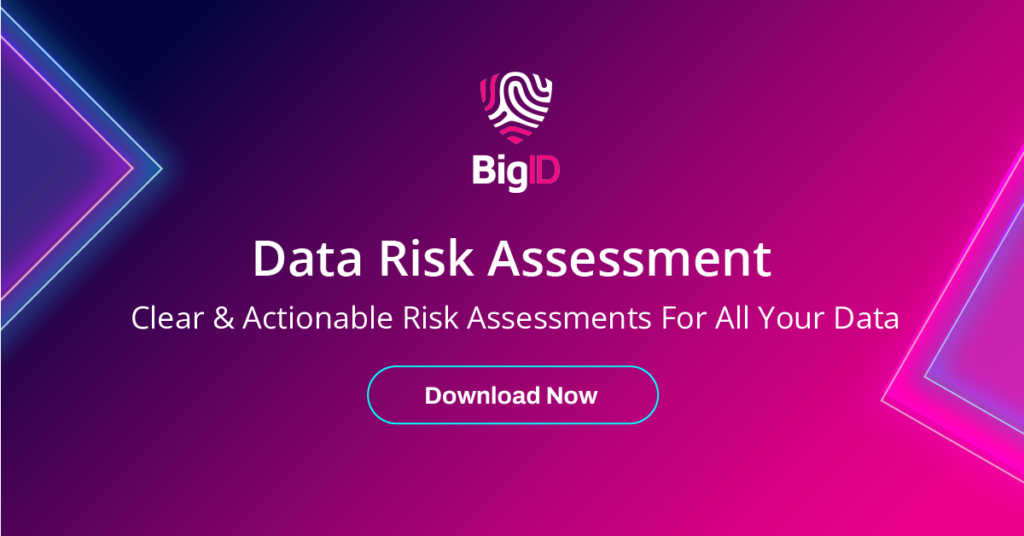
AI Application in Cybersecurity
AI, particularly through machine learning and generative AI, has revolutionized cybersecurity. Automated security operations centers learn from common cyber threat patterns and indicators to proactively identify and mitigate evolving threats before they become critical. This capability enhances the entire lifecycle of cybersecurity defense, from prevention and detection to response and recovery.
Additionally, such solutions use gen AI to meet rigorous security standards. Here’s how AI is becoming invaluable in digital security and privacy:
Threat Detection
To identify threats in real time, you need to analyze large amounts of data to detect potential cyber threats, such as malware, viruses, and phishing attacks, utilizing applications of AI in cybersecurity. This becomes easy with automation. AI-powered threat detection systems can identify patterns, anomalies, and behavioral changes that may indicate a cybersecurity incident. They alert you as soon as they detect something, allowing for timely response and mitigation.
User Behavior Analysis
Anomalies in user behavior can indicate insider threats or unauthorized access, which AI can help identify through advanced analytics. These aberrations can be unusual login activities, file access, and network usage. AI-powered user behavior analytics can identify suspicious activities that may pose security risks and help prevent data breaches to improve security.
Vulnerability Assessment
Cybercriminals tend to exploit any weaknesses in IT systems, networks, and applications. AI can conduct automated vulnerability assessments to identify these potential weaknesses. AI-powered assessment tools can prioritize exposures, so security teams can take proactive measures to mitigate risks.
Security Automation and Orchestration
You can improve the efficiency and effectiveness of cybersecurity operations by automating security processes and workflows. AI-powered security automation and orchestration platforms can automatically detect, analyze, and respond to security incidents. That reduces response time and minimizes the impact of cyber attacks.
Threat Hunting
Certain threats may not be detected by traditional security tools. You can, however, use AI to assist in proactively hunting for them and mitigating AI security risks. AI-powered threat hunting tools can analyze large datasets, conduct anomaly detection, and generate actionable insights to identify advanced threats or zero-day attacks that may bypass traditional security defenses.
Malware Analysis
AI can analyze and classify malware samples to identify their behavior, characteristics, and potential impact. AI-powered malware analysis tools can detect new and unknown malware strains, generate signatures, and develop behavioral models to improve the accuracy and speed of malware detection and prevention.
Security Analytics
To identify potential security incidents and generate actionable insights, you must analyze security logs, network traffic, and other security-related data. Again, this is easily done with automation. AI-powered security analytics platforms can detect patterns, trends, and anomalies that may indicate cybersecurity threats, enabling security teams to take proactive measures to mitigate risks.
Security Governance for Generative AI
To secure AI architecture, you require a strategic governance approach — one that marries advanced AI capabilities with stringent security standards and ethical considerations. It requires more than just robust technology — it demands a strategic governance structure that aligns with both regulatory demands and ethical considerations.
Regulatory Compliance: For a solid governance structure, you need to understand and adhere to relevant AI regulations and safety standards. This involves a meticulous overview of the labyrinth of laws, ensuring compliance with data protection regulations and industry standards. You not only safeguard sensitive information but also fortify your organization’s credibility with a proactive approach to regulatory compliance.
Ethical Considerations: Innovation shouldn’t come at the cost of responsible AI practices. An organization’s security posture can be ethical as it integrates AI while using it to streamline and automate processes.
Role of AI Security Officers (CISOs): AI Security Officers, or Chief Information Security Officers (CISOs), are the stakeholders who oversee the complexities of AI safety and security best practices. These professionals are responsible for navigating the evolving landscape, implementing best practices, and ensuring that the organization’s AI initiatives align with security objectives. Their role extends beyond technological expertise; they help manage and mitigate the AI risk management challenges. As AI continues to shape the future, appointing a dedicated CISO will be necessary.
Building Security Controls for Privacy
Companies can leverage AI technologies to accelerate business initiatives without compromising privacy compliance by implementing these key practices.
- Data Privacy by Design: Privacy considerations must be incorporated into the design and development of secure AI systems from the outset. Implement privacy-preserving techniques — such as data anonymization, aggregation, and encryption — to protect sensitive data used in AI solutions.
- Robust Data Governance: Strong data governance practices guarantee that data used in AI models is collected, stored, and processed in compliance with relevant privacy regulations. You need to obtain proper consent from data subjects, define data retention policies, and implement access controls to restrict unauthorized access to data.
- Ethical Data Use: The data used in AI models must be obtained and used ethically, and in compliance with applicable laws and regulations. You would have to avoid biased data, be transparent with its usage, and obtain consent for data sharing, when required.
- Model Explainability: For complete transparency, you should strive to understand and explain how AI models make decisions. This can help ensure that the use of AI is transparent and accountable, and in compliance with privacy regulations. Techniques such as explainable AI (XAI) can provide insights into how these models arrive at their predictions or decisions.
- Regular Audits and Monitoring: To detect and address any privacy compliance gaps, you should conduct regular audits and monitor model security. This includes ongoing monitoring of data handling practices, model performance, and compliance with privacy regulations, and taking corrective actions as needed.
- Employee Training and Awareness: Employees who develop and deploy AI systems should be offered training and awareness programs to ensure they understand the importance of privacy compliance and adhere to best practices.
- Collaborating with Privacy Experts: To make your AI initiatives secure and aligned with privacy standards, you should leverage the expertise of privacy professionals or consultants. Collaborate with privacy experts to identify potential compliance risks and develop appropriate mitigation strategies.
Improve Security in AI Technologies with BigID
BigID is an industry leading data platform for privacy, security, and governance. The platform is equipped to reduce AI security threats by:
- Identifying PII & Other Sensitive Data: BigID’s powerful data discovery and classification capabilities help your business automatically identify and classify PII like credit card numbers, social security numbers, customer data, intellectual property, and more sensitive data across your entire data landscape, including structured and unstructured data. Understand exactly what data you’re storing — before it’s misused in AI systems or LLM.
- Enforcing Data Privacy Policies: BigID allows you to consistently define and enforce data privacy policies. You can create automated workflows to detect and flag any AI model that processes sensitive data without proper authorization or consent. This proactive approach enables your models to comply with privacy regulations, such as GDPR, CCPA, and HIPAA, minimizing the risk of data breaches and associated legal liabilities.
- Align with AI Governance Frameworks: The rapid development of AI is accompanied by new evolving regulations like the AI Executive Order and the Secure AI Development Guidelines — both of which require the responsible and ethical use of AI. BigID utilizes a secure-by-design approach, which allows your organization to comply with emerging AI regulations.
- Data Minimization: Automatically identify and minimize redundant, similar, and duplicate data. Improve the data quality of AI training sets — all while reducing your attack surface and improving your organization’s security risk posture.
- Secure Data Access: Manage, audit, and remediate overexposed data — especially the data you may not want used in AI training models. Revoke access from overprivileged users, both internally and externally, to reduce insider risk.
Get a free 1:1 demo to see how BigID can reduce your organization’s risk of data breaches and ensure your AI systems are compliant.