7 Data Quality Dimensions You Should Know
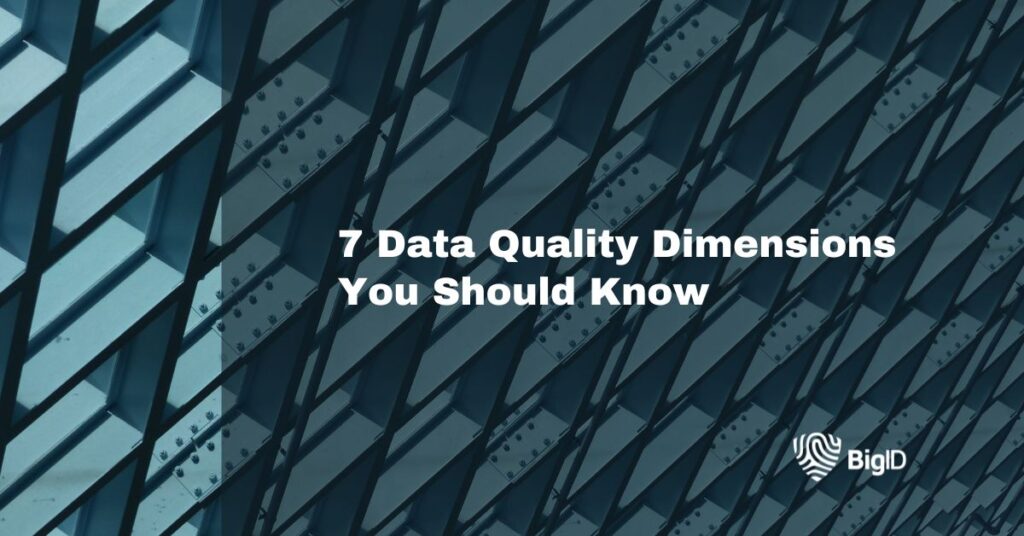
Everyone knows the saying “quality over quantity”—and this is especially true when applied to data quality. Businesses are continually harvesting vast amounts of data, but its true value lies in its quality. Poor data quality can result in costly mistakes, missed opportunities, and even security breaches. As the landscape of AI and machine learning continues to evolve, understanding the dimensions of data quality becomes critical. Read on to explore the meaning of data quality, its dimensions, how it’s measured, and what organizations should do to ensure data quality and avoid privacy and security risks.
What is Data Quality?
Data quality refers to the accuracy, completeness, reliability, and relevance of data. It’s not just about having tons of data— it’s about having the right data, at the right time, in the right format, and without errors. To achieve this, data quality is typically evaluated across seven core dimensions:
The 7 Dimensions of Data Quality
While there are different frameworks for understanding data quality, one commonly used framework defines the 7 dimensions of data quality as follows:
- Accuracy: This dimension assesses the correctness of the data. Are the values in your dataset free from errors, discrepancies, and inconsistencies? Inaccurate data can lead to misguided decisions.
- Completeness: Complete data ensures that you have all the necessary information for a specific purpose. Missing data can create gaps in your analysis, which can result in misinformed strategies.
- Consistency: Data consistency verifies that data across various sources or systems is uniform and coherent. Inconsistencies can lead to misunderstandings and mistakes when data is integrated.
- Reliability: Reliable data is data that can be consistently depended upon to be accurate and consistent over time. It’s a key factor in building trust in data-driven decisions.
- Relevance: Relevant data is data that actually matters to your organization’s goals. Unnecessary data can obscure what’s important and waste resources.
- Timeliness: Timely data is data that’s available when it’s needed. Delays in data availability can hinder decision-making and cause missed opportunities.
- Integrity: Data integrity refers to the overall quality and trustworthiness of data. It encompasses accuracy, consistency, and reliability, ensuring data is uncorrupted and trustworthy.
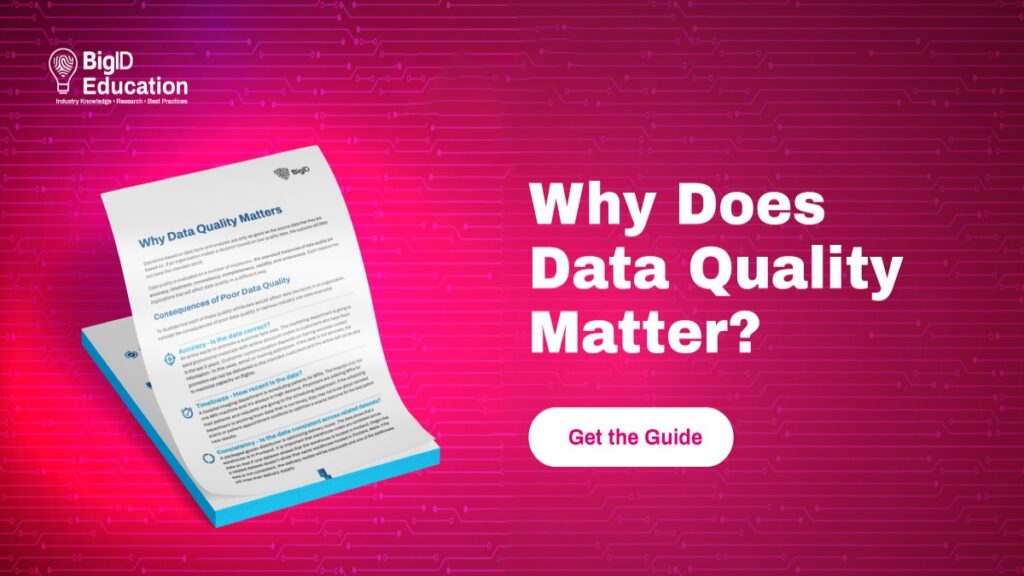
Measuring Data Quality
Measuring data quality involves using various tools and techniques, often encompassing data profiling, data cleansing, and data validation. These processes identify errors, missing data, and inconsistencies, allowing organizations to take corrective actions.
Supporting Stats: According to a Gartner report, poor data quality costs organizations an average of $15 million per year.
Ensuring Data Quality and Mitigating Risks
Data Governance: Establish robust data governance policies and procedures to ensure data quality. This includes data ownership, data stewardship, and data quality standards.
Data Quality Tools: Invest in data quality tools and software that can automate data cleansing and validation processes.
Data Privacy and Security: Implement strong data privacy measures to protect sensitive information. Encryption and access control are essential components.
Regular Audits: Conduct regular audits of your data to identify and rectify quality issues. Continuous monitoring can help maintain data quality over time.
The Future of Data Quality in the AI and ML Era
As AI and machine learning continue to be integrated into solutions, data quality becomes even more crucial. These technologies rely on high-quality data for training and decision-making. Organizations need to adopt forward-thinking strategies, such as:
Automated Data Quality: Implement AI-driven data quality tools that can proactively detect and correct data issues in real-time.
Ethical AI: Ensure that AI and ML models are trained on unbiased, high-quality data to avoid perpetuating discrimination and biases.
Data Governance for AI: Create specific data governance frameworks for AI and machine learning projects to ensure data quality and compliance.
Data quality is a fundamental aspect of any data-driven organization. Understanding the dimensions of data quality and how to measure it is vital for success. As AI and machine learning become increasingly prevalent, organizations must invest in data quality to harness the full potential of these technologies and avoid privacy and security risks. With a forward-thinking approach, organizations can stay ahead in the evolving landscape of data quality.
BigID’s Approach to Ensuring & Enhancing Data Quality
BigID is the industry leading platform that enables organizations to know their enterprise data and take action for privacy, protection, and perspective. Leveraging advanced machine learning and deep data insight, BigID transforms data discovery and data intelligence to address data privacy, security, and governance challenges across all types of data, at petabyte-scale, on-prem and in the cloud.
BigID’s Data Quality App enables you to actively monitor the consistency, accuracy, completeness and validity of your data— so you can make critical decisions with trustworthy data. Get 360° data quality insights by business entities and data sources, all in a unified inventory — across all of your data, wherever it lives. Monitor the consistency, accuracy, completeness and validity of your data in one place.
Some of the features include:
- Unified dashboard with data quality scores across all types of data types & data sources
- Relevant data quality scores as a result of a dynamic profiling across all data, rather than insights coming from a sample set
- Out-of-the-box dimensions like Profiling, Patterns and Outliers for a holistic immediate view into data quality
- ML recommendations for data quality metrics
- Integration to enable retention and remediation workflows
For better data quality without the headache— get a 1:1 demo with BigID today.