6 Steps to Securing & Governing Your Data In a Generative AI World
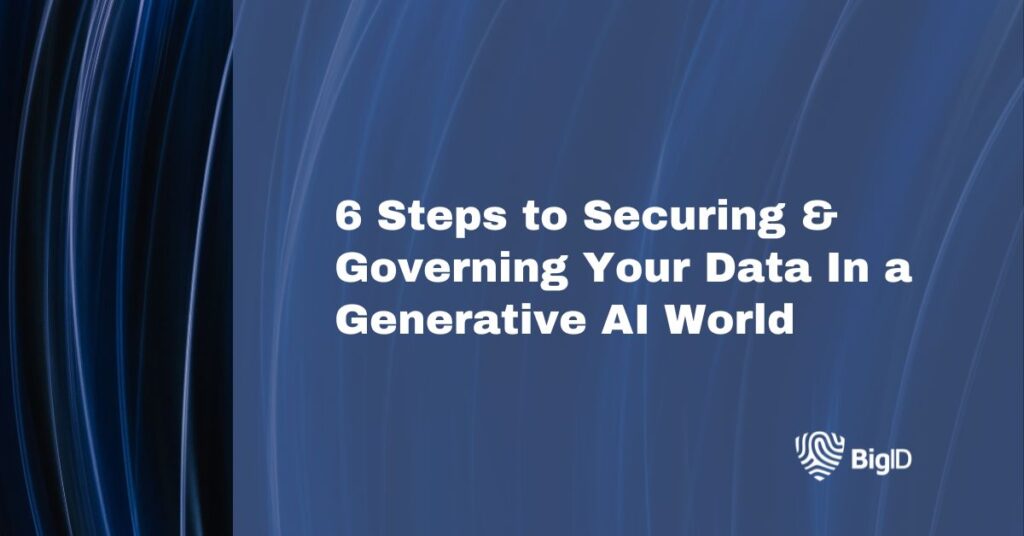
Generative AI is ushering in a new era of intelligence and automation, fundamentally reshaping the way we process information. Generative AI excels at producing imagery, text, and audio, while seamlessly synthesizing diverse datasets. By harnessing vast amounts of both structured and unstructured data for training, generative AI can uniquely identify patterns before creating something new, as opposed to just analyzing existing data.
Organizations leverage generative AI, which leverages large language models (LLMs), to transform operations, enhance productivity, optimize processes, and improve decision-making across various functions. Common use cases include:
- Customer Support: LLMs automate responses, aid in creating knowledge bases, and power chatbots for quick internal and external support.
- Internal Communication: LLMs assist in drafting documents, emails, and reports, and enhance communication within the organization.
- Data Analysis: LLMs enable natural language queries, making data analysis more accessible, and assisting in trend analysis.
- Project Management: LLMs automate tasks and improve communication, enhancing project management.
While generative AI has rapidly enhanced our lives, work, and learning, it’s crucial to understand the potential risks tied to its use. Specifically, there are concerns about how sensitive and private financial, personal, and intellectual property data are being used as training feedstock for LLMs. Unfortunately, this generative AI opens up another avenue of risk and associated data security, privacy, and governance implications.
Risks & Concerns with Generative AI
Sensitive Data Exposure
Information used to train LLMs may inadvertently include sensitive, personal, or regulated data. Exposing this type of data to the wrong people (both inside and outside the organization) can lead to unauthorized exposure, access, use, and ultimately a breach – compromising individuals, third parties, and the organization itself. Strong data governance and controls are crucial to prevent sensitive data from being included within training datasets.
Bias & Discrimination
LLMs are susceptible to learning and perpetuating biases present in their training data. This could lead to unfair or discriminatory outcomes. Continuous monitoring, bias detection, and mitigation are strategies to ensure fairness and prevent unintended consequences.
Hallucinations & Misinformation
Poor quality data to train LLMs may produce content that is not factual, leading to the creation of hallucinations or misleading information. Better data quality and governance programs should be implemented along with data validation mechanisms, ensuring that outputs uphold accuracy and reliability standards.
Manipulation & Adversarial Attack
Malicious actors and insider threats can exploit vulnerabilities in AI models to purposefully retrieve sensitive information and successfully carry out a breach from the inside. Organizations must be able to monitor and detect suspicious activity on top of implementing the right measures and controls to protect their most important data.
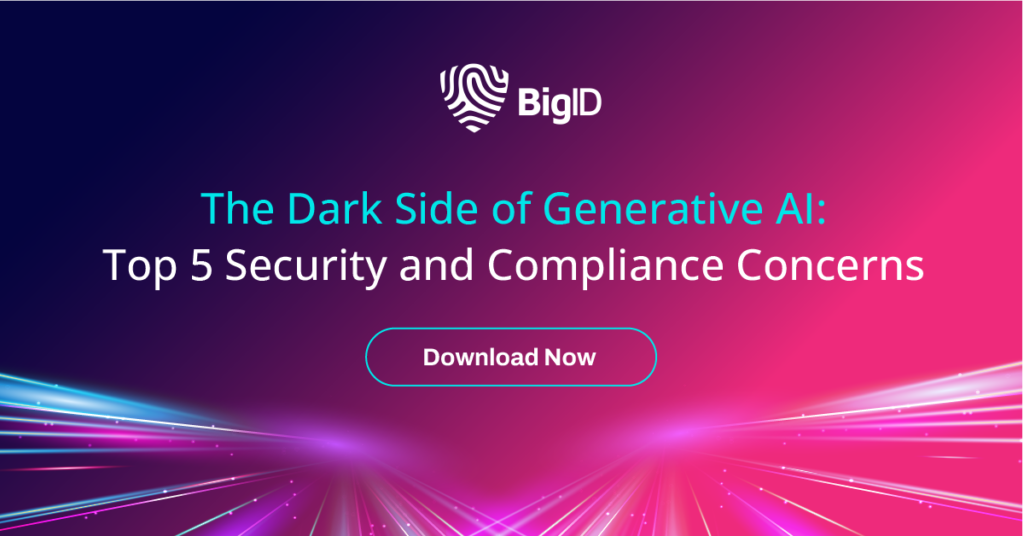
Govern Generative AI Without Compromising Data Security & Privacy
Here are six tenets towards better governing generative AI without compromising data security and privacy:
1. Discover Your Data
Data security, privacy, and governance start with understanding your data environment. Comprehensive data discovery is foundational to securing and protecting your most sensitive and valuable data, enabling you to better implement the risk remediation efforts – especially when generative AI tools are being trained on data located across particular parts of your environment.
With BigID, connect to and scan for sensitive data across any data source and type – cloud or on-prem – from unstructured and structured data to mainframes, messaging, pipelines, big data, NoSQL, IaaS, SaaS, applications, and beyond. Scan unstructured data 95% faster with Hyperscan. Save time and avoid sensitive data blind spots across AWS, GCP, and Azure with Cloud Auto-Discovery capabilities. Discover your data across your estate – regardless of where it lives.
2. Classify Your Data with Context
Finding your data is one thing – knowing it is another. Classifying your data with deeper context, insight, and meaning allows you to better understand the nature of the data – what type of data it is, who it belongs to, how sensitive it is, where it lives, and who’s got access to it. This enables you to better manage data risk and remediation to prevent unwanted exposure and use from LLMs.
With BigID, combine traditional pattern-matching techniques with advanced, ML and NLP-based classification to achieve unparalleled accuracy and scalability in data classification. Customize and fine-tune classifiers to locate specific types of sensitive data unique to your organization. Ultimately build a complete and dynamic sensitive data inventory with contextual attributes for holistic understanding.
3. Identify Similar Data
Identifying similar data is essential to ensure that the AI can effectively apply its learned knowledge to new, unseen data, leading to more robust and reliable performance in real-world applications. The process involves exposing the AI to a wide range of data to ensure robust learning and avoid undesirable behaviors to specific instances.
BigID is the first and only vendor that provides cluster analysis, a patent-pending approach that BigID leverages to compare data in order to identify similarity and score dispersion from a mean. Using the BigID cluster analysis in tandem with BigID’s labeling capability makes it easy for security and data governance professionals to find related documents and soon databases to ensure consistent security, consolidation, retention and minimization strategies.
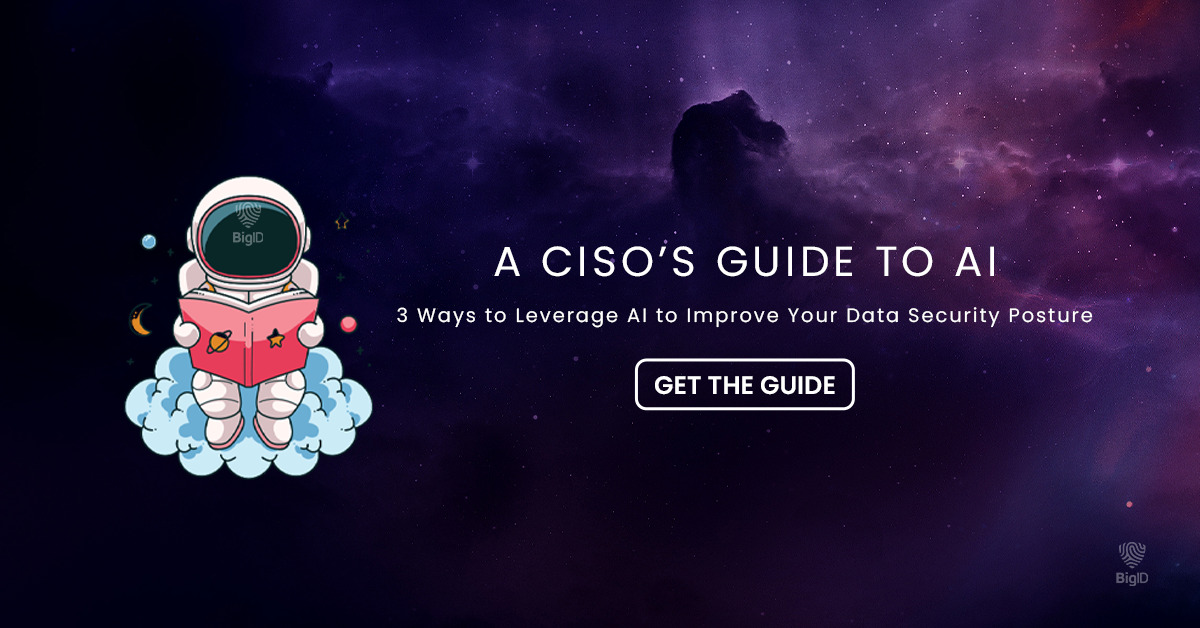
4. Label and Tag Your Data
Precisely labeling and tagging with detail and context allows for better management and enforcement when handling and monitoring sensitive data like credit card info (PCI), personal information (PI) and regulated health information (PHI). In addition, data labeling, tagging, or annotation is essential for training large language models (LLMs) by providing labeled datasets that serve as a ground truth for supervised learning. It enables the models to understand language nuances, learn task-specific associations, and improve overall performance.
Leverage BigID’s advanced ML and AI-based discovery and classification to establish a foundational and comprehensive data labeling practice that’s thorough, precise, and consistent for all your data – strengthening your ability to manage, regulate, and control how that information flows. Integrate with and enrich native DLP and labeling frameworks across the cloud and on-prem, including Microsoft Purview, Google Drive, and more. Better label your data to better enforce data policies around privacy and protection – as well as supervised LLM learning.
5. Detect & Remediate Your Data Risks
Proactive data security and risk posture management are vital to mitigating your data risks and vulnerabilities to prevent exposure, access, and use. Having the ability to detect, investigate, and remediate your data at risk – whether that data is being unintentionally leveraged by LLMs, or accessible to the wrong users or groups – will help to quickly neutralize the chance of a breach.
BigID’s industry-leading data and risk posture management platform allows you to quickly and precisely find and fix your biggest data risks and vulnerabilities across your environment – with automation, intelligence, and ease. Identify, score, and prioritize critical data risks by severity level according to sensitivity, location, accessibility, and more. Remediate data your way – centrally manage data remediation workflows or decentralize them across your data security stack.
6. Perform Data Risk Assessments
Conducting regular assessments of your data security and risks is important when trying to maintain a strong data security risk posture. These assessments are easy ways to continually drive awareness and decision-making when it comes to your data, especially across the security organization as well as with other stakeholders, given that cybersecurity and risk have become board-level concerns.
BigID’s Data Risk Assessment reports stand out from typical assessments. With comprehensive coverage across all data types and locations (structured and unstructured, cloud, hybrid, and on-premises), our assessments incorporate all your information, wherever it resides. BigID’s broad range of data security use cases aggregates diverse risk indicators to save you time and provide actionable insights.
Want to learn more about how you can better govern, protect, and secure your AI data? Schedule a 1:1 Demo with one of our data experts today!