Data mapping is the cornerstone of understanding data relationships, origins, and destinations. In this blog, we delve into the essence of data mapping, its significance across various sectors, and the evolving landscape shaped by AI.
What is Data Mapping?
Businesses invariably have to move from one system to another or integrate two databases. However, different systems have their own unique data structures to organize information in their data models. Data mapping is the process of matching data fields in both models to ensure no data duplicates from one set to the other.
It’s a visual representation of how your enterprise data moves and transforms, whether between databases or from databases to applications.
Mapping your data tells you if it is usable and accurate in its new environment by identifying, analyzing, and documenting the data flow from source to destination. It is a navigational tool that enables your business to understand its data landscape and is integral to data management.
Why is Data Mapping Important?
Data mapping techniques aren’t just about documentation — they also help your business get more out of its data. They optimize processes, enhance decision-making, and uncover insights crucial for innovation by understanding data flows. From healthcare to finance, every sector benefits from good data mapping.
Here’s why the process is so important:
For Consistency and Accuracy
Use a data map to ensure the data sets from the source match correctly and properly with the destination system. This is especially important during data integration and for smoother data migrations (or the process of moving your data), where you need to reconcile data formats of different types. However, it can also be essential for data transformation if you move data from one format to another according to the requirements of the destination system.
It can also be especially useful for data warehouse automation. The information in a data warehouse comes from various data sources, and has to be consolidated and structured to be analyzed. Data mapping can be invaluable for the data transformation required for this.
For Enhanced Customer Experience
In customer-centric industries such as retail and e-commerce, understanding customer behavior is important. Use data mapping to bring together different customer touchpoints, from website interactions to social media engagement. It creates a holistic view of the customer journey. You can analyze these flows to personalize the user experience and offerings, optimize marketing strategies, and ultimately enhance customer satisfaction.
For Strengthened Cybersecurity Measures
A big part of a strong cybersecurity posture is protecting data from breaches. Data mappers can identify vulnerabilities in the flow of data and highlight potential intrusion points. Cybersecurity professionals can then define the data security measures to protect against cyber threats with this view of the data movement across your networks, applications, and endpoints.
For Improved Data Quality
Sets of data lying in your data systems for a long time can lose quality over time. What do we mean by quality? You may have new data, but the old data may not have been replaced. Alternatively, your business may have changed formats over time and have inconsistent data elements. Improper data transfer might have resulted in duplicate entries. Create data maps to weed out these inconsistencies and duplications, enriching the data.
For Better Decision Making
Business decisions should be based on facts. However, when your facts (or data) are not accurate, your decisions will suffer as a result. Data mapping helps improve the quality of information, making it easier to analyze. That means that you can make better, more informed decisions.
For Streamlining Business Operations
When data is stored without thought and strategic planning, you may not have a clear picture of how it moves across different systems or how it’s used by applications. With a data map, you can see where it’s coming from and where it’s going. You can then make sure it is clear and consistent so it goes smoothly across systems.
For Regulatory Compliance
When you map data, you also follow how it moves around within your systems. This tracking can help with auditing to comply with the various data protection laws, such as GDPR, CCPA, and HIPAA. It tells you exactly where your data goes and how it’s handled.
What is the Data Mapping Process?
Data mapping involves several steps:
- Inventorying Data: Identifying all sources and types of data within an organization.
- Analyzing Relationships: Understanding how data moves between different systems, applications, and stakeholders.
- Documenting: Creating detailed maps or diagrams that illustrate data flows, transformations, and dependencies.
- Implementing Controls: Establishing governance mechanisms to ensure data accuracy, security, and compliance.
- Continuous Monitoring: Regularly updating and reviewing data maps to reflect changes in the organization’s data landscape.
Common Data Mapping Examples
- Healthcare: In healthcare, data mapping is instrumental in ensuring patient privacy, tracking medical records, and optimizing treatment pathways.
- Finance: Financial institutions rely on data mapping to detect fraudulent activities, manage risk, and streamline regulatory reporting.
- Retail: Retailers leverage data mapping to understand customer behavior, optimize inventory management, and personalize marketing efforts.
Data Mapping Challenges
Businesses today deal with large volumes of data. The sheer scale and complexity of these data ecosystems make effective data mapping quite challenging. Here are some common data mapping challenges amplified by large data volumes:
Scalability and Performance
Handling large volumes of data poses significant scalability and performance challenges for data mapping processes. As data volumes continue to grow exponentially, organizations must ensure that their data mapping solutions can efficiently process and map massive datasets without compromising performance.
Data Quality Issues
With large volumes of data, ensuring data quality becomes even more critical. The sheer quantity of data increases the likelihood of inaccuracies, inconsistencies, and incompleteness, making it challenging to maintain data quality standards throughout the mapping process.
Data Complexity and Variety
Dealing with large volumes of diverse data types further complicates data mapping efforts. Organizations must contend with structured, unstructured, and semi-structured data across various platforms and systems, making it challenging to achieve interoperability and consistency in mapping disparate data sources.
Integration with Legacy Systems
Large organizations often grapple with legacy systems that use outdated technologies and proprietary formats. Integrating and mapping data from these legacy systems with modern data platforms becomes increasingly challenging as data volumes grow, requiring organizations to bridge the gap between legacy and modern systems effectively.
Data Lineage and Traceability
Managing data lineage and traceability becomes more complex with large volumes of data. Tracking the origins and transformations of data throughout its lifecycle becomes increasingly challenging, making it essential for organizations to establish clear data lineage to ensure data quality, compliance, and accountability.
Data Silos and Fragmentation
Large volumes of data exacerbate data silos and fragmentation within organizations. As data volumes grow, data silos become more entrenched, hindering data integration efforts and preventing organizations from creating a unified view of their data assets.
Complex Business Logic and Transformations
Dealing with large volumes of data often involves complex business logic and transformations. Organizations must navigate intricate business rules, data semantics, and transformation requirements, making it challenging to document, manage, and execute complex mapping rules effectively.
Skills and Expertise Gap
The complexity of dealing with large volumes of data requires specialized skills and expertise in data management, integration technologies, and domain knowledge. Organizations may struggle to recruit and retain qualified data mapping professionals with the necessary skills and experience to tackle large-scale data mapping challenges effectively.
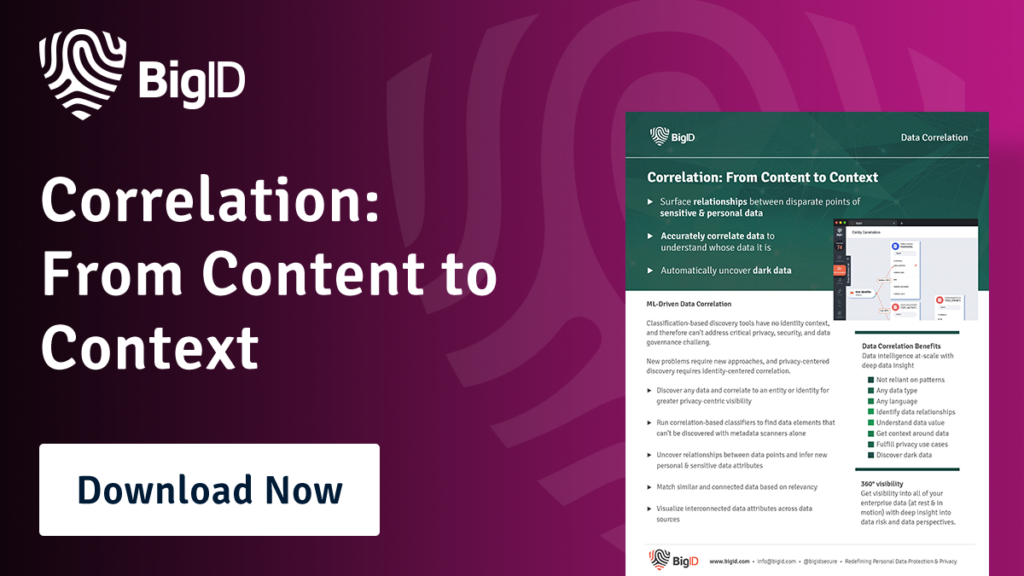
How the Right Data Mapping Tools Help
Data mapping can be automated, semi-automated, or manual. As you can imagine, manual data mapping can be onerous, especially when dealing with large sets of sample data. That’s why automated data mapping is more popular. Data mapping software use AI to analyze and map data in real time. It enables data analysts and architects to view data content, flow, and transformations as they happen.
Good data mapping tools will also help you optimize formats without having to worry about human error. They’ll convert the data from a source format into the destination format instantly, and do so accurately. Their data mapping capabilities will also help you review and update your maps. Essentially, they make data management tasks easier for you.
You can also use semi-automated data mapping, where the tool will carry out certain tasks while others will require human intervention. These may require human approval in certain tasks during the process of moving data. However, they can reduce some of the load that your data managers face.
AI’s Impact on Data Mapping
Artificial Intelligence has revolutionized data mapping by offering automated solutions that can process vast datasets rapidly. Machine learning algorithms can analyze complex data relationships, identify patterns, and even predict future data flows. AI-powered data mapping not only accelerates the mapping process but also enhances its accuracy and scalability.
Data Management with BigID
As organizations continue to use data, the importance of dependable data mapping practices grows. BigID is the leading provider for data privacy, security, and governance empowering organizations to get more value out of their data.
With BigID you can:
- Discover all your data — everywhere: Find and inventory your sensitive, critical, and high-risk data for a clear view of all the data you store and maintain. PI and PII to automate inventory & third-party data flows for continuous compliance.
- Achieve Regulatory Compliance: Map business processes and data flows to fulfill compliance requirements for GDPR Article 30, CCPA, LGPD, and more.
- Automate reporting: Proactively discover, document, and report on third-party data sharing & discrepancies in processing. Create comprehensive audits and reviews to provide data protection regulators with necessary proof of compliance.
- Know your data risk — and reduce it: Prioritize your most high-risk, sensitive data. Identify and minimize risk on sensitive data with risk scores that incorporate data parameters like data type, location, residency, and more.
To see how BigID can help your organization embrace the next-gen of data mapping — get a 1:1 demo with our data experts today.