Understanding Stale Data
Data drives nearly every critical decision-making process in today’s digital world. It fuels innovation but lurking beneath the surface lies a silent adversary known as “stale data.” In this article, we delve into the concept of stale data, exploring its definition, impact on organizations, underlying causes, common occurrences, and the regulatory landscape surrounding its management.
What is Stale Data?
Stale data refers to information within an organization’s databases or systems that has become outdated or obsolete, yet remains accessible and potentially influential in decision-making processes. This phenomenon arises when the information stored in databases fails to reflect the current state of affairs or lacks relevance due to elapsed time or changing circumstances.
Identifying Traits of Stale Data
Identifying stale data involves recognizing certain signs or indicators that suggest data may be outdated or no longer relevant. Here are some simple signs to look out for:
- Inconsistencies with Current Information: If data contradicts or does not align with current information or real-world events, it may be a sign that the data has become stale.
- Lack of Updates or Changes: When data remains unchanged for an extended period, despite expectations of updates or revisions, it could indicate that the data has become stale.
- Obsolete References or Context: If data references outdated technologies, products, or processes that have since been replaced or updated, it may be a sign of stale data.
- Errors or Anomalies in Analysis: Data that produces unexpected or inconsistent results when analyzed may indicate that it is outdated or inaccurate, leading to errors in decision-making or analysis.
- Data Aging Beyond Thresholds: If data exceeds predefined thresholds for acceptable age or relevance, it may be considered stale and in need of refresh or validation.
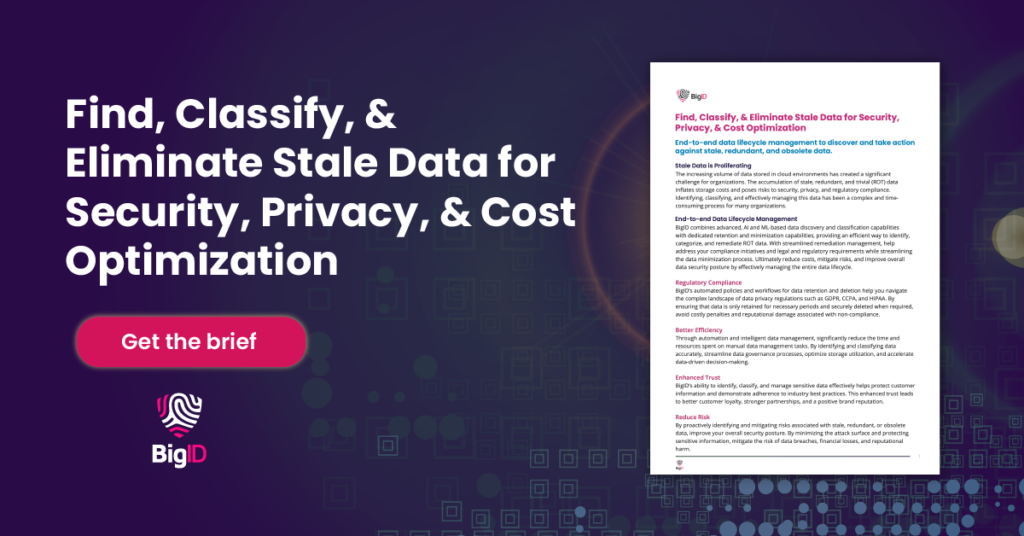
Unveiling the Root Cause of Stale Data: Strategies for Prevention
Several factors contribute to the emergence of stale data within organizational databases. To seamlessly overcome theses causes of stale data, organizations can implement a comprehensive approach that addresses each of the identified factors:
Data Aging
Over time, data naturally becomes outdated as circumstances change, rendering previously accurate information obsolete.
- Regular Data Refresh: Establish protocols for regular data refresh cycles to ensure that information remains current and reflects the latest developments or changes in relevant circumstances. This may involve automating data updates through scheduled processes or triggers based on predefined criteria.
- Timestamps and Versioning: Implement timestamping and versioning mechanisms to track the age of data and identify when it becomes outdated. By maintaining historical versions of data, organizations can accurately trace changes over time and assess the validity of information.
- Dynamic Data Sources: Integrate dynamic data sources that provide real-time or near-real-time updates, reducing the reliance on static datasets prone to aging. Leveraging APIs, streaming data sources, and event-driven architectures can facilitate the ingestion of fresh data as it becomes available.
Lack of Data Governance
Inadequate data governance practices, such as poor data quality management, insufficient metadata management, and lax data lifecycle management, can exacerbate the accumulation of stale data.
- Establish Data Governance Framework: Develop a robust data governance framework that encompasses policies, procedures, and accountability mechanisms for managing data quality, metadata, and lifecycle management. Define clear roles and responsibilities for data stewards, custodians, and governance committees to oversee data governance initiatives.
- Data Quality Management: Implement data quality management processes to ensure the accuracy, completeness, consistency, and timeliness of data. This may involve data profiling, cleansing, enrichment, and validation activities to identify and rectify discrepancies or anomalies.
- Metadata Management: Invest in metadata management solutions to catalog and document data assets, including their definitions, lineage, usage, and governance policies. Metadata repositories serve as a centralized source of truth for understanding data semantics and fostering data transparency and discoverability.
- Data Lifecycle Management: Define clear policies and procedures for managing the lifecycle of data from creation to archival or disposal. This includes specifying retention periods, archival criteria, data purging mechanisms, and compliance with regulatory requirements.
Integration Challenges
Data integration processes involving multiple systems and sources may result in inconsistencies and discrepancies that lead to stale data.
- Data Integration Framework: Implement a robust data integration framework that standardizes data formats, protocols, and mappings to facilitate seamless interoperability between disparate systems and sources. Utilize integration platforms, ETL (Extract, Transform, Load) tools, and API management solutions to streamline data movement and transformation processes.
- Data Mapping and Transformation: Conduct thorough data mapping exercises to identify and reconcile inconsistencies or discrepancies between integrated systems. Establish data transformation rules and mappings to harmonize disparate data schemas and ensure data coherence and consistency.
- Data Quality Assurance: Implement data quality assurance measures, such as validation checks, reconciliation processes, and exception handling mechanisms, to detect and rectify integration errors or discrepancies in real-time. Establish data quality metrics and KPIs to monitor the effectiveness of integration processes and identify areas for improvement.
By adopting these strategies, organizations can effectively mitigate the risks of stale data by addressing the underlying causes of data aging, lack of data governance, and integration challenges. This enables organizations to maintain data integrity, accuracy, and relevance, thereby enhancing decision-making capabilities and driving business value from their data assets.
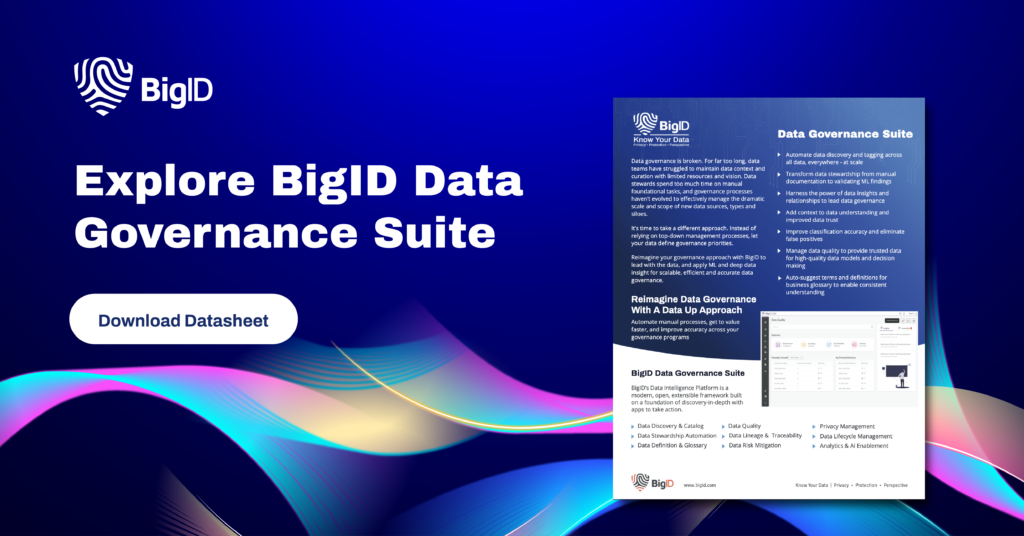
Impact of Stale Data on Organizations
The presence of stale data can have far-reaching implications for organizations across various industries:
- Inaccurate Decision-Making: Relying on outdated or irrelevant data can lead to erroneous conclusions and flawed decision-making, jeopardizing business outcomes and competitive advantage.
- Diminished Operational Efficiency: Stale data can impede operational efficiency by causing delays, errors, and inefficiencies in processes that rely on accurate and timely information.
- Erosion of Customer Trust: Organizations risk eroding customer trust and loyalty if they provide inaccurate or outdated information, leading to dissatisfaction and potential reputational damage.
Regulatory Requirements for Handling Stale Data
In an increasingly regulated environment, organizations must adhere to data protection and privacy regulations that govern the handling of stale data:
- Data Retention Policies: Regulatory frameworks such as GDPR (General Data Protection Regulation) and CCPA (California Consumer Privacy Act) mandate organizations to establish data retention policies that define the permissible duration for retaining data and specify procedures for securely disposing of stale data.
- Data Security Measures: Regulations often require organizations to implement robust data security measures, including encryption, access controls, and audit trails, to safeguard stale data from unauthorized access or breaches.
- Compliance Reporting: Organizations may be obligated to demonstrate compliance with regulatory requirements pertaining to data management, including the handling of stale data, through regular reporting and auditing processes.
The prevalence of stale data poses significant challenges for organizations striving to maintain data integrity, operational efficiency, and regulatory compliance. By implementing proactive data governance strategies, leveraging advanced data management technologies, and adhering to regulatory requirements, organizations can mitigate the risks associated with stale data and unlock the full potential of their data assets in driving business success.
Cloud Computing and AI: Catalysts for Data Freshness
Cloud computing and AI have both contributed to addressing the issue of stale data rather than exacerbating it. Here’s how:
Real-time Data Processing
Cloud computing platforms offer scalable and flexible infrastructure for processing data in real-time. With cloud-based solutions, organizations can ingest, analyze, and act upon data as it is generated, minimizing the risk of data becoming stale. AI technologies, such as machine learning and predictive analytics, can leverage this real-time data processing capability to make timely and informed decisions based on the most up-to-date information available.
Data Quality and Governance
Cloud-based data management services often include features for data quality monitoring, cleansing, and governance. AI algorithms can be employed to detect and correct data quality issues in real-time, ensuring that only accurate and reliable data is used for analysis and decision-making. Additionally, cloud platforms provide tools for implementing data governance policies and enforcing compliance with regulatory requirements, further reducing the likelihood of stale data accumulation.
Advanced Analytics
AI and machine learning algorithms excel at uncovering patterns, trends, and insights from large volumes of data. By leveraging cloud-based analytics services, organizations can continuously analyze and derive value from their data, identifying potential issues or anomalies before they lead to stale data. These advanced analytics capabilities enable organizations to proactively manage their data assets and maintain data freshness.
Automated Data Integration and Management
Cloud computing platforms offer automated data integration and management capabilities that streamline the process of ingesting, transforming, and distributing data across disparate systems and sources. AI-driven automation tools can intelligently handle data integration tasks, ensuring that data remains synchronized and up-to-date across the organization. By automating routine data management processes, organizations can reduce the risk of data becoming stale due to manual errors or delays.
BigID’s Approach to Minimizing & Mitigating Stale Data
For organizations looking to improve their data quality and mitigate the risk of stale data— BigID has you covered. Our data-centric approach to privacy, security, compliance, and AI data management combines deep data discovery, next-gen data classification, and risk management. Know where your data is located, how sensitive it is, and who’s accessing it.
With BigID you can:
- Know Your Data: The ability to identify your data is the first critical step in both reducing risk and eliminating stale data. Organizations need to identify all their data, everywhere. BigID’s data discovery and classification helps organizations automatically identify their sensitive, personal, and regulated data across the entire data landscape.
- Data Classification: To classify all data, everywhere in order to meet compliance for data privacy and data protection BigID intuitive platform classifies by category, type, sensitivity, policy, and more.
- Reduce Risk: Manage access to sensitive and critical business data – organizations need to incorporate access control to identify who has (and who should have) access to sensitive data. BigID’s Access Intelligence App helps organizations identify and remediate high-risk data access issues with ML-based insight to identify and prioritize file access risk.
- Incident Response: When incidents happen, every second counts. BigID’s identity- aware breach analysis effectively assesses the scope and magnitude of a data breach. Quickly determine which users and personal data have been compromised and respond accordingly.
To eliminate stale and obsolete data from your organization’s data landscape— book a 1:1 demo with BigID today.