Streamlining Data Remediation: Best Practices Guide
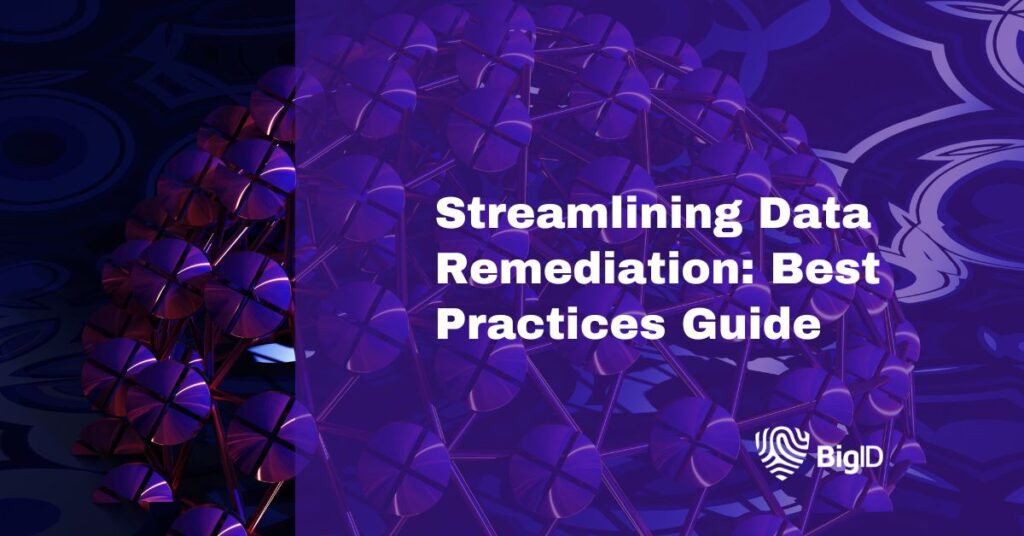
What is data remediation?
Data remediation refers to the process of identifying, cleaning, and correcting inaccurate, incomplete, or irrelevant data within a dataset. The purpose of data remediation is to improve the quality and reliability of data so that it can be used for accurate analysis, reporting, and decision-making. This may involve activities such as removing duplicates, correcting spelling or formatting errors, filling in missing information, and deleting irrelevant data. Data remediation is important because it helps ensure that organizations can rely on accurate and trustworthy data to make informed decisions.
Why is it important?
Inaccurate or incomplete data can lead to flawed analysis and decision-making. If decisions are based on bad data, it can result in wasted resources, missed opportunities, or even financial losses. Data remediation helps ensure regulatory compliance. Organizations are often required by law or industry regulations to maintain accurate and complete records, and failure to do so can result in legal and financial consequences.
Additionally, data remediation can improve operational efficiency. By cleaning up and organizing data, organizations can streamline processes, reduce errors, and make better use of their resources.
Who is responsible?
The responsibility for managing data remediation can vary depending on the organization and the specific situation. In general, data remediation is a collaborative effort between different departments and individuals within an organization.
The IT department is often responsible for managing the technical aspects of data remediation, such as identifying and correcting data errors and inconsistencies. Data stewards or data owners are responsible for maintaining data quality and ensuring that data is accurate and up-to-date. Business analysts or data analysts may also be involved in the process, as they are responsible for analyzing data and using it to make informed decisions.
Ultimately, everyone in the organization has a role to play in data remediation, as it requires a collaborative effort to identify, correct, and prevent data issues. It is important to establish clear roles and responsibilities for data management to ensure that everyone understands their role in maintaining data quality.
Leveraging the benefits
Data remediation, the process of identifying and correcting inaccurate, incomplete, or irrelevant data, offers several benefits for businesses. Here are some of the key benefits:
- Improved data quality: Data remediation helps improve the accuracy, completeness, and consistency of data, which can lead to better decision-making, improved customer service, and increased operational efficiency.
- Compliance with regulations: Data remediation is crucial for compliance with data protection regulations, such as the GDPR and CCPA. By identifying and correcting inaccurate or incomplete data, organizations can reduce the risk of non-compliance and potential fines.
- Reduced risk of data breaches: Data remediation helps reduce the risk of data breaches by identifying and removing sensitive or personal data that is no longer needed. This can help prevent unauthorized access to sensitive information and protect against data loss.
- Cost savings: Poor data quality can be costly for businesses, leading to lost revenue, wasted resources, and decreased productivity. By investing in data remediation, organizations can save money by reducing the cost of data errors and improving the efficiency of their operations.
Consider the trends & statistics
Recent trends and statistics demonstrate the increasing importance of data remediation for businesses. Here are some examples:
- The cost of poor data quality: Poor data quality can have a significant financial impact on businesses. According to Gartner, poor data quality costs organizations an average of $15 million per year.
- The rise of regulatory compliance: Regulatory compliance continues to be a major driver of data remediation efforts. According to a recent survey by Experian, 82% of organizations reported that they are subject to data protection regulations.
- The importance of data privacy: Data privacy concerns are also driving the need for data remediation. A recent survey by Talend found that 70% of organizations see data privacy as a top priority.
- The increasing use of data analytics: The use of data analytics continues to grow, and organizations are increasingly relying on data to make business decisions. However, inaccurate or incomplete data can lead to flawed analysis and decision-making, making data remediation more important than ever.
- The impact of the COVID-19 pandemic: The COVID-19 pandemic has highlighted the importance of accurate and reliable data for decision-making. Businesses have had to rely on data to make quick and informed decisions during the pandemic, making data remediation a critical priority.
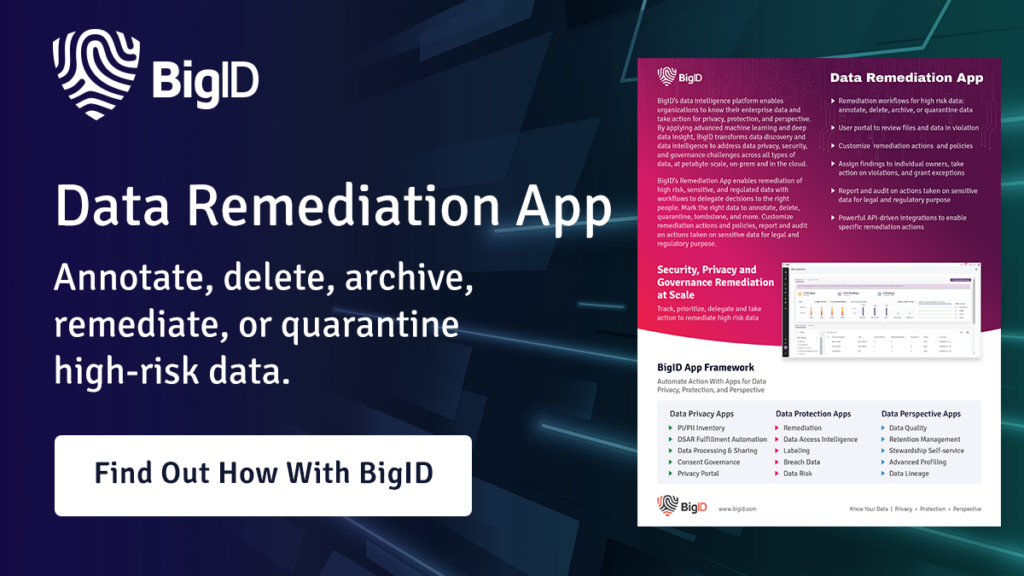
Overcoming data remediation challenges
Organizations can encounter several common challenges when it comes to data remediation, including:
- Lack of data governance: Without proper data governance policies and procedures, data can become fragmented, inconsistent, and difficult to manage. This can make data remediation efforts more challenging. To prevent this, organizations should establish clear data governance practices and ensure that data is properly managed and maintained.
- Limited resources: Data remediation can be a resource-intensive process, and organizations may not always have the necessary resources to devote to it. To prevent this, organizations should prioritize their data remediation efforts and allocate the necessary resources to ensure that they can effectively remediate the most critical data issues.
- Resistance to change: Data remediation often requires changes to processes and systems, and some stakeholders may resist these changes. To prevent this, organizations should communicate the benefits of data remediation and involve stakeholders in the process to ensure that they understand the importance of maintaining data quality.
- Complex data environments: Organizations may have complex data environments that make it difficult to identify and remediate data issues. To prevent this, organizations should ensure that their data environments are properly architected and that data is properly integrated and managed.
- Limited data understanding: Organizations may not always have a clear understanding of their data, including its quality, sources, and lineage. This can make it difficult to identify and remediate data issues. To prevent this, organizations should conduct regular data assessments to ensure that they have a clear understanding of their data and its quality.
Creating a data remediation plan
Strategize your data remediation plan by following these steps:
- Define the scope and goals: Clearly define the scope of the data remediation effort and establish the goals you want to achieve. Identify the data sources, systems, and stakeholders involved in the process.
- Assess the data quality: Conduct a data quality assessment to identify the accuracy, completeness, consistency, and relevancy of the data. Use data profiling tools or data quality metrics to identify areas that need remediation.
- Prioritize data remediation: Prioritize the data that requires the most urgent attention. This can be based on the impact of the data on business operations or the risk associated with data errors.
- Develop a remediation plan: Develop a detailed plan that outlines the steps required to remediate the data issues. This plan should include the resources, timelines, and responsibilities needed to carry out the remediation effort.
- Implement the plan: Implement the remediation plan and execute the steps identified in the plan. This may involve cleaning, standardizing, or enriching the data, as well as updating processes and systems to ensure ongoing data quality.
- Monitor and measure progress: Monitor the progress of the remediation effort and measure the effectiveness of the plan. Use data quality metrics to track improvements and identify areas that still require attention.
- Continuous improvement: Establish a culture of continuous improvement by regularly reviewing and updating the data remediation plan to ensure that it remains effective and relevant.
Data Remediation in Action with BigID
BigID is a data intelligence platform that helps organizations with data remediation by providing advanced data discovery, classification, and remediation capabilities. BigID can help in the following ways:
- Data discovery: BigID helps organizations discover and identify sensitive and personal data across their entire data landscape, including on-premises, cloud, and third-party systems including structured, unstructured, and dark data. Read more on how to overcome data classification challenges.
- Define policies: BigID’s Data Remediation App gives you the power to define policies and specify controls around specific sets of data based on sensitivity level or regulation.
- Remediation workflows: BigID’s Data Remediation App provides customizable workflows to help your organization track and manage data remediation tasks and ensure that they are completed on time.
- Integrations: BigID integrates with a wide range of systems and applications, making it easy to automate data remediation tasks and ensure that data is properly protected and managed.
To learn more about how BigID can improve the security posture of your organization and automate remediation efforts— get a free 1:1 demo today.