AI in Healthcare: Transforming Medicine While Tackling Privacy, Security, and Ethical Challenges
Artificial intelligence (AI) is transforming the healthcare industry, improving diagnostics, treatment personalization, and administrative efficiency. However, AI in healthcare also raises critical concerns about data privacy, security, and governance. As AI adoption grows, organizations must navigate the complexities of compliance, cybersecurity risks, and ethical considerations to maximize its benefits while safeguarding patient data. By 2030, AI in healthcare is projected to reach $187 billion
What is Artificial Intelligence in Healthcare?
AI refers to the use of machine learning, natural language processing (NLP), and deep learning algorithms to analyze data, identify patterns, and make informed decisions. In healthcare, AI is used for medical imaging analysis, drug discovery, robotic surgeries, and predictive analytics, helping clinicians improve patient care and operational efficiency.
AI Applications in the Medical Industry
AI’s integration into healthcare has led to significant advancements:
- Diagnostic Accuracy: AI algorithms analyze medical images and data to assist in early disease detection. For instance, AI has been used to detect lung cancer at early stages, potentially saving lives.
- Personalized Treatment: AI assesses patient data to recommend tailored treatment plans, improving efficacy and reducing adverse effects.
- Administrative Efficiency: AI-powered virtual assistants manage administrative tasks, such as scheduling and documentation, allowing healthcare professionals to focus more on patient care.
Types of Sensitive Data Used in Healthcare and AI’s Role
AI relies on vast amounts of sensitive patient data to function effectively. Understanding the types of data used is crucial in addressing privacy, security, and governance challenges.
Key Types of Sensitive Healthcare Data
- Protected Health Information (PHI) – Personal identifiers like names, addresses, Social Security numbers, and medical records.
- Electronic Health Records (EHRs) – Digitized patient records containing medical history, treatments, and prescriptions.
- Genomic Data – Genetic information used for personalized medicine and disease prediction.
- Medical Imaging Data – X-rays, MRIs, CT scans, and pathology slides analyzed by AI for disease detection.
- Real-Time Patient Monitoring Data – Collected from wearable devices, IoT sensors, and remote health monitoring systems.
AI uses these datasets to enhance diagnostics, predict disease progression, and personalize treatments. However, protecting this sensitive information remains a major challenge.
Examples of AI in Healthcare
AI is driving innovation across multiple areas of medicine, improving accuracy, efficiency, and patient outcomes.
1. AI in Medical Imaging
AI-powered algorithms analyze radiology images to detect diseases like cancer, pneumonia, and neurological disorders faster than traditional methods. For example, Google’s DeepMind developed an AI model capable of detecting over 50 eye diseases with accuracy matching top ophthalmologists.
2. AI in Drug Discovery
AI accelerates drug discovery by identifying potential compounds and predicting their effectiveness. In 2023, Insilico Medicine used AI to develop a new drug for pulmonary fibrosis, significantly reducing research timelines.
3. AI in Predictive Analytics
AI models analyze patient data to predict disease outbreaks, hospital readmissions, and individual health risks. For instance, the Mayo Clinic’s AI-driven cardiovascular risk prediction system helps doctors intervene earlier in high-risk patients.
4. AI Chatbots and Virtual Assistants
AI-powered virtual assistants, such as Babylon Health’s chatbot, provide symptom assessments and suggest possible treatments, reducing the burden on healthcare professionals.
5. AI in Personalized Treatment Plans
AI tailors treatments based on genetic and clinical data. IBM Watson, for example, assists oncologists by analyzing vast medical literature and patient records to recommend cancer treatment options.
AI Threats in Healthcare
While AI presents promising advancements, it also introduces serious risks related to cybersecurity, data privacy, and ethical concerns.
1. Data Breaches and Cybersecurity Risks
AI-driven healthcare systems store massive amounts of patient data, making them prime targets for cyberattacks. The 2023 cyberattack on an Australian fertility clinic, where hackers stole nearly a terabyte of patient data, highlighted vulnerabilities in healthcare cybersecurity.
2. Algorithmic Bias and Discrimination
AI systems trained on biased datasets may produce inaccurate diagnoses, disproportionately affecting underrepresented groups. For example, studies have shown that some AI dermatology models struggle to identify skin conditions in darker-skinned patients due to a lack of diverse training data.
3. AI Manipulation and Adversarial Attacks
Hackers can manipulate AI models by feeding them altered data, leading to misdiagnoses or incorrect treatment recommendations.
4. Ethical and Legal Issues
Who is responsible when an AI-powered diagnosis is incorrect? The deployment of AI in healthcare raises ethical questions about decision-making autonomy and accountability. Legal frameworks often lag behind technological advancements, creating uncertainties in liability and regulatory compliance.
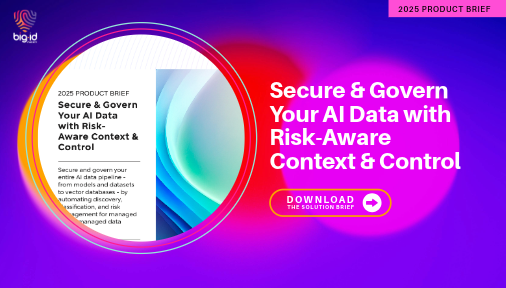
AI Integration Impacts on Data Privacy, Security, and Governance
While AI offers numerous benefits, its integration into healthcare systems poses several challenges:
Data Privacy Challenges
The reliance on vast amounts of patient data raises significant privacy concerns. Healthcare organizations must comply with regulations like HIPAA to protect patient information. However, the increasing use of AI complicates compliance, as traditional privacy protections may be inadequate. Consider the following:
- HIPAA Compliance Risks: AI models must comply with HIPAA and GDPR regulations to ensure patient data confidentiality.
- Data Re-identification: Even anonymized data can be re-identified when combined with other datasets, risking patient privacy.
- Cross-Border Data Regulations: AI applications often process data across jurisdictions, complicating regulatory compliance.
Cybersecurity Threats
AI systems are vulnerable to cyberattacks, which can compromise sensitive patient data and disrupt healthcare services. A notable example is the cyberattack on Genea, an Australian IVF clinic, where hackers obtained nearly a terabyte of sensitive patient data, including medical records and personal information. Consider the following:
- Ransomware Attacks: AI-driven healthcare systems are increasingly targeted by ransomware, locking access to critical patient data.
- Insider Threats: Employees with AI access may misuse or leak sensitive data, necessitating strict access controls.
Governance Strategies for AI in Healthcare
To ensure AI’s ethical and secure use, healthcare organizations must implement strong governance policies:
- Transparent AI Models: Organizations must document AI decision-making processes to ensure accountability.
- Bias Mitigation Strategies: Training AI with diverse datasets reduces discrimination risks.
- Robust Cybersecurity Frameworks: Multi-layered security protocols safeguard AI systems from cyber threats.
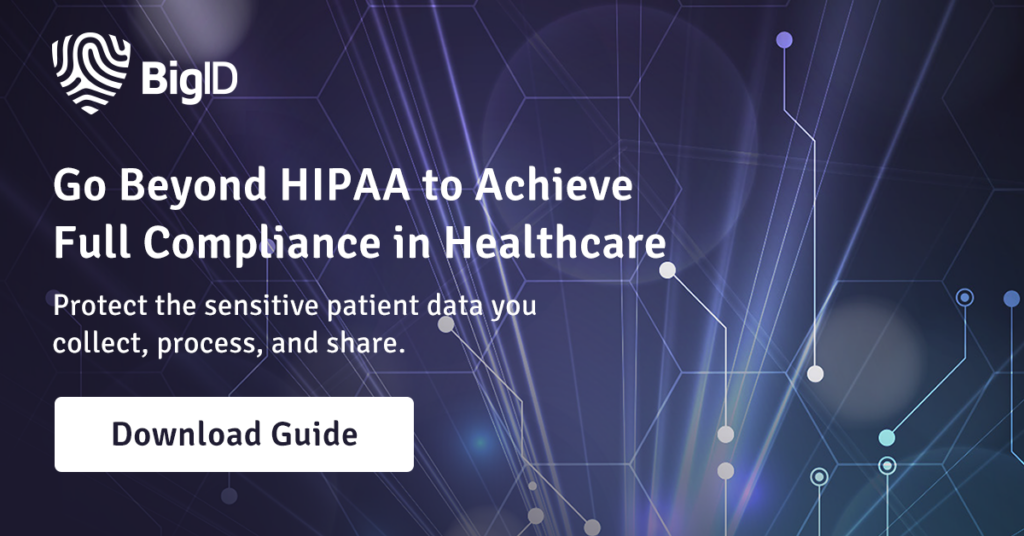
The Future of AI and Healthcare
AI’s role in healthcare is rapidly evolving, with emerging innovations poised to reshape the industry.
1. AI-Powered Precision Medicine
AI will further personalize treatments by integrating genetic, environmental, and lifestyle data to optimize patient care.
2. Real-Time AI Diagnostics
Wearable AI devices will enable real-time health monitoring, providing instant insights into vital signs and early disease detection.
3. AI-Driven Robotic Surgery
AI-assisted surgical robots will enhance precision and reduce risks in complex procedures, improving patient recovery rates.
4. Federated Learning for Secure Data Sharing
New AI approaches like federated learning will allow hospitals to collaborate on AI model training without sharing raw patient data, improving privacy and security.
5. AI Regulations and Ethical AI Development
Governments and regulatory bodies will enforce stricter AI guidelines, ensuring ethical implementation and reducing risks associated with bias and security breaches.
Recommendations for Healthcare Organizations
To effectively integrate AI, healthcare organizations should:
- Invest in Cybersecurity: Implement robust security measures to protect AI systems from cyber threats, safeguarding patient data and maintaining trust.
- Ensure Regulatory Compliance: Stay abreast of evolving data protection laws and ensure AI applications comply with all relevant regulations.
- Promote Transparency: Maintain clear documentation of AI decision-making processes to build trust among patients and healthcare professionals.
- Foster Collaboration: Engage with stakeholders, including patients, clinicians, and policymakers, to address ethical concerns and align AI applications with societal values.
Secure Your AI Ecosystem with BigID Next
AI is revolutionizing healthcare, offering breakthroughs in diagnostics, treatment personalization, and patient care. However, the widespread adoption of AI use in healthcare also presents significant challenges, including privacy concerns, security threats, and ethical dilemmas. Healthcare organizations must proactively address these issues by implementing tailored security solutions.
BigID Next is the first modular data platform to address the entirety of data risk across security, regulatory compliance, and AI. It eliminates the need for disparate, siloed solutions by combining the capabilities of DSPM, DLP, data access governance, AI model governance, privacy, data retention, and more — all within a single, cloud-native platform.
BigID Next helps organizations get:
- Complete Auto-Discovery of AI Data Assets: BigID Next’s auto-discovery goes beyond traditional data scanning by detecting both managed and unmanaged AI assets across cloud and on-prem environments. BigID Next automatically identifies, inventories, and maps all AI-related data assets — including models, datasets, and vectors.
- First DSPM to Scan AI Vector Databases: During the Retrieval-Augmented Generation (RAG) process, vectors retain traces of the original data they reference, which can inadvertently include sensitive information. BigID Next identifies and mitigates the exposure of Personally Identifiable Information (PII) and other high-risk data embedded in vectors, ensuring your AI pipeline remains secure and compliant.
- AI Assistants for Security, Privacy, and Compliance: BigID Next introduces the first-of-its-kind agentic AI assistants, designed to help enterprises prioritize security risks, automate privacy programs, and support data stewards with intelligent recommendations. These AI-driven copilots ensure compliance stays proactive, not reactive.
- Risk Posture Alerting and Management: AI systems introduce data risks that go beyond the data itself — and extend to those with access to sensitive data and models. BigID Next’s enhanced risk posture alerting continuously tracks and manages access risks, providing visibility into who can access what data. This is especially critical in AI environments, where large groups of users often interact with sensitive models and datasets. With BigID Next, you can proactively assess data exposure, enforce access controls, and strengthen security to protect your AI data.
To see how BigID Next can help you leverage AI without sacrificing security— get a 1:1 demo with our experts today.