Modern Data-centric Organizations Require a New Outcome-based Approach to DLP
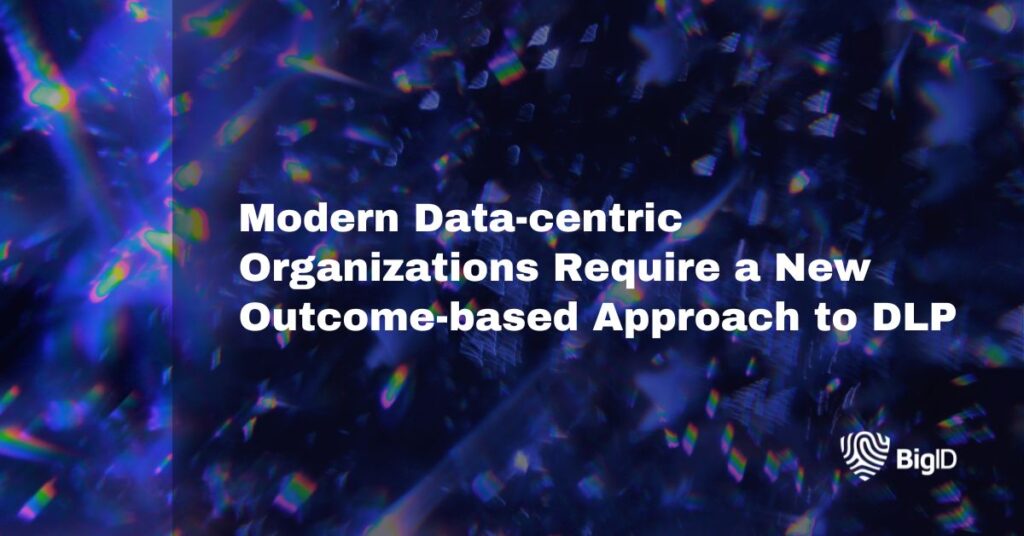
Implementing Data Loss Prevention (DLP) technology has historically been a struggle for organizations and security teams as there is a constant battle of tuning just right to stop sensitive data from needlessly leaving the organization while not impeding the course of business at the same time.
Modern data usage requirements are leading organizations to re-evaluate utilizing traditional DLP approaches and instead focus on outcome-based results.
When you build an outcome-based approach to DLP, you achieve flexibility and expand your options for data control.
(Forrester – How to Build an Outcome-based DLP Approach, November 24, 2023).
While legacy DLP monitors data in transit across the network, newer approaches — such as Cloud DLP -— starts at the source and secures data stored and shared in cloud environments. Cloud DLP, is by nature outcome-based as it focuses beyond technology controls to also encompass processes and practices.
This approach more efficiently detects and prevents violations to corporate policies regarding the use, storage, and transmission of sensitive data; enabling organizations to enforce policies to prevent unwanted dissemination of sensitive information — without hampering the flow of business.
DLP technology controls are more effective in protecting key organizational data when incorporated with other data management and data security measures including: discovery, classification, access controls, remediation, etc. Once sensitive data has been identified, secured and governed this is a basis for an effective data security backstop including the following use cases:
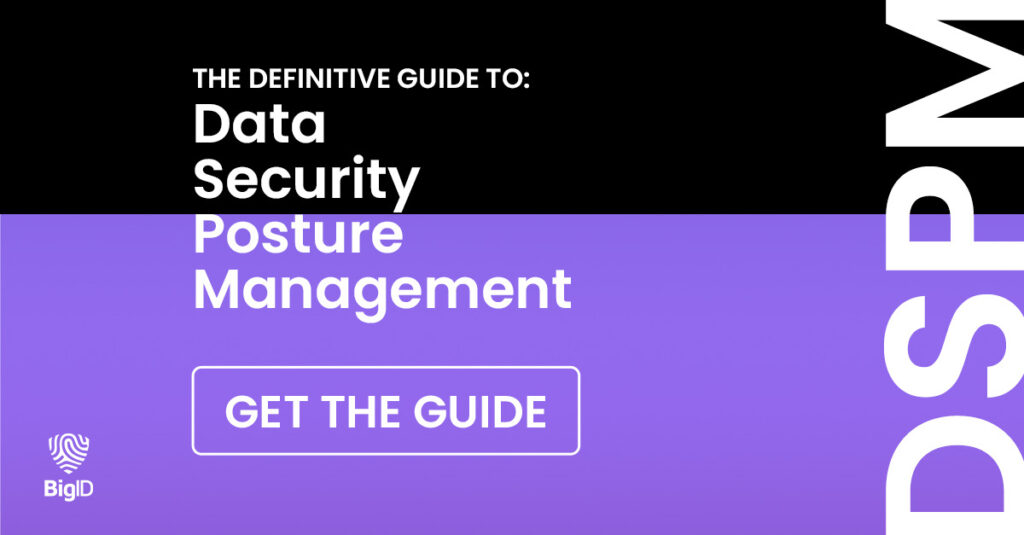
Legacy network-based DLP is often too little too late when used as a primary control for insider risk
Unfortunately, too often effectively mitigating insider risk and DLP have been used as synonymous terms. That is comparing “apples to oranges”. DLP is simply a final control attempting to prevent sensitive data from leaving the organization. Whereas managing insider risk is a much broader discipline that includes:
- Identifying data and individuals impacted
- Defining and controlling your data
- Creating and applying policies
- Creating rules of engagement
- Building a risk team
- Refining consistent processes
- Training users
- Monitoring data use an user activity
The legacy DLP approach attempts to prevent data that meets poorly defined criteria from leaving the organization. Whereas cloud DLP is far more effective in managing insider risk as it is more preventative and starts with identifying, classifying, securing and governing sensitive data before users even get a chance to access, move or send it.
AI-enabled data discovery & classification, identifying improper access and applying remediation can reduce access to sensitive data by well over 90% when done properly. This takes a lot of pressure off of the security team when trying to apply other policies and security controls and DLP. This also increases engagement from the business team and users as the managing insider risk process seems more manageable.
Generative AI requires new data-centric controls to ensure data security and governance
Generative AI is a generational transformational technology. Just like the personal computer and smartphones it isn’t a matter of “if” but a matter of “when” for adoption. As such, organizations are scrambling to secure sensitive data that might flow through GenAI training models. The main debate is where during the gen AI lifecycle is the best place to apply security controls and ensure that the organization protects its key data assets. The possible points are:
- Inclusion of sensitive data controls in the creation of the algorithm for the model
- Selection of APIs
- Consumption of data into the model
- Management of LLM
- Creation of output through user interface
While all steps are logical options, all have limitations. Trying to include sensitive data management controls in the algorithm, or even through the selection of APIs, leaves too much to chance and can also reduce the effectiveness of the model. Management of the LLM is difficult as the LLM is often stored in a vector database as mathematical equations without any direct data visibility. Finally, users have too many options at the output stage including a wide variety of ways to access the model through different interfaces and browsers.
What’s left is applying governance and security at the point of source data that is feeding the model. Network-based DLP won’t help, but a cloud DLP outcome-based approach starting at the data element can. Deep and broad discovery and classification, especially for unstructured data sets, can identify key organizational data and controls can be applied to prevent crown jewel data, privacy data, and other sensitive data from being used to populate the LLMs. Applying proactive controls on data before it ever starts the generative API controls greatly mitigates risk and makes further controls down through the process including DLP is much more manageable.
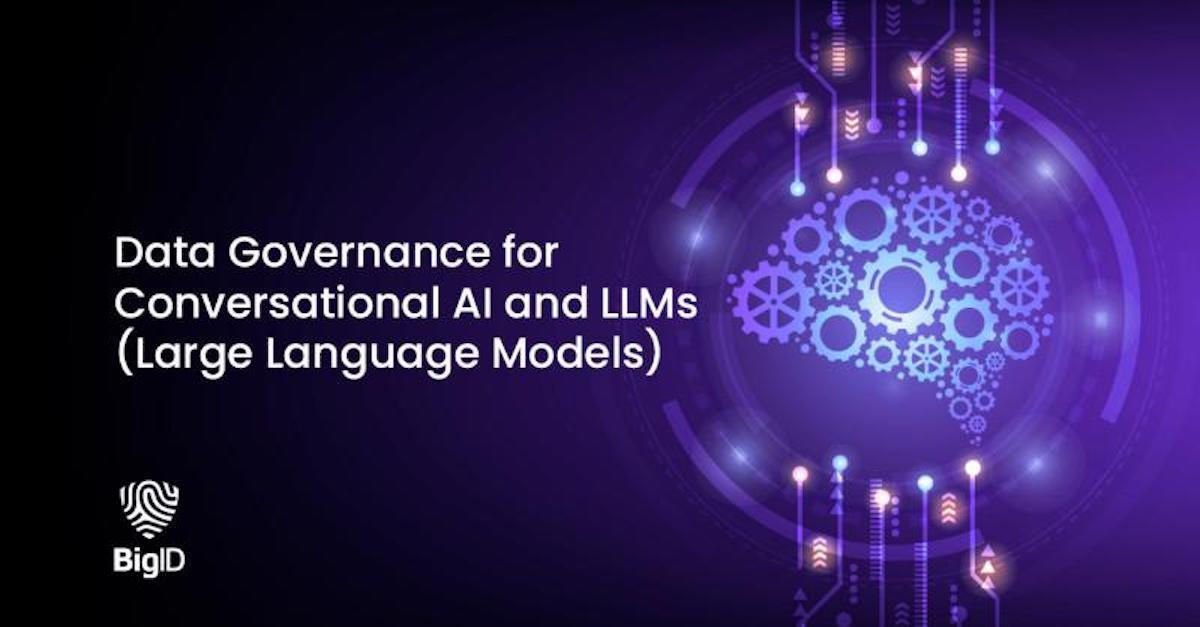
DLP ≠ Zero Trust
Legacy DLP approaches can’t come close to guaranteeing Zero Trust. Instead, Zero Trust requires a variety of data management capabilities combined with data security controls.
The initial, preparatory phase to reach intermediate Zero Trust maturity includes two major initiatives for data and devices: discovery and classification.
(Forrester – Chart Your Course To Zero Trust Intermediate, March 8, 2023).
You can’t govern and control what you don’t know. AI-enabled data discovery not only surfaces the “what”, but also the context and relationships of data. Sensitive data is identified through the discovery process and in-depth classification ensures that data and metadata is reliably tagged.
Once initial and ongoing discover and classification is established, data security controls can be effectively applied including:
- Data Access Governance
- Automated Remediation
- Rights Management
- Data Minimization
- Secure browsers
- DLP
- Obfuscation (encryption, masking, redaction, tokenization)
Data access governance also starts at the discovery process. As data is scanned, overexposed and over-privileged is identified and remediation of access violations needs to be automated as much as possible. By removing improper access and identifying and deleting stale data the workload for DLP and obfuscation technologies is greatly reduced.
Incorporating a data-centric cloud DLP approach into a Zero Trust strategy enhances data security controls and ensures a comprehensive approach to safeguarding sensitive information.
Building an outcome-based DLP approach involves that it is just one of the data management and security controls applied to scenarios such as Insider Risk, Generative AI, and Zero Trust. Tailoring DLP to an outcome-based approach ensures a robust and adaptive data protection framework in today’s dynamic cybersecurity landscape.
To learn more about how BigID enables data security, including DLP— schedule a 1:1 demo with our experts today.