Many of us have heard that data is the new oil, because it powers modern digital commerce. But the analogy isn’t limited to the idea that data fuels the information economy. Like oil, data is also fluid: it can seep and flow into almost any reservoir or store. It can easily be “mined” (collected or generated), “piped” (spread or transferred), “refined” (edited and bifurcated), “sold”, and “used up” (erased). In fact, tracking data throughout its lifecycle from creation to disposition has not kept pace with innovations for its production, sharing, and storage. However, as companies come to rely on data to fuel how they engage customers, empower their employees, and optimize their business performance, knowing and understanding their data has taken on a new dimension and priority.
Data Everywhere
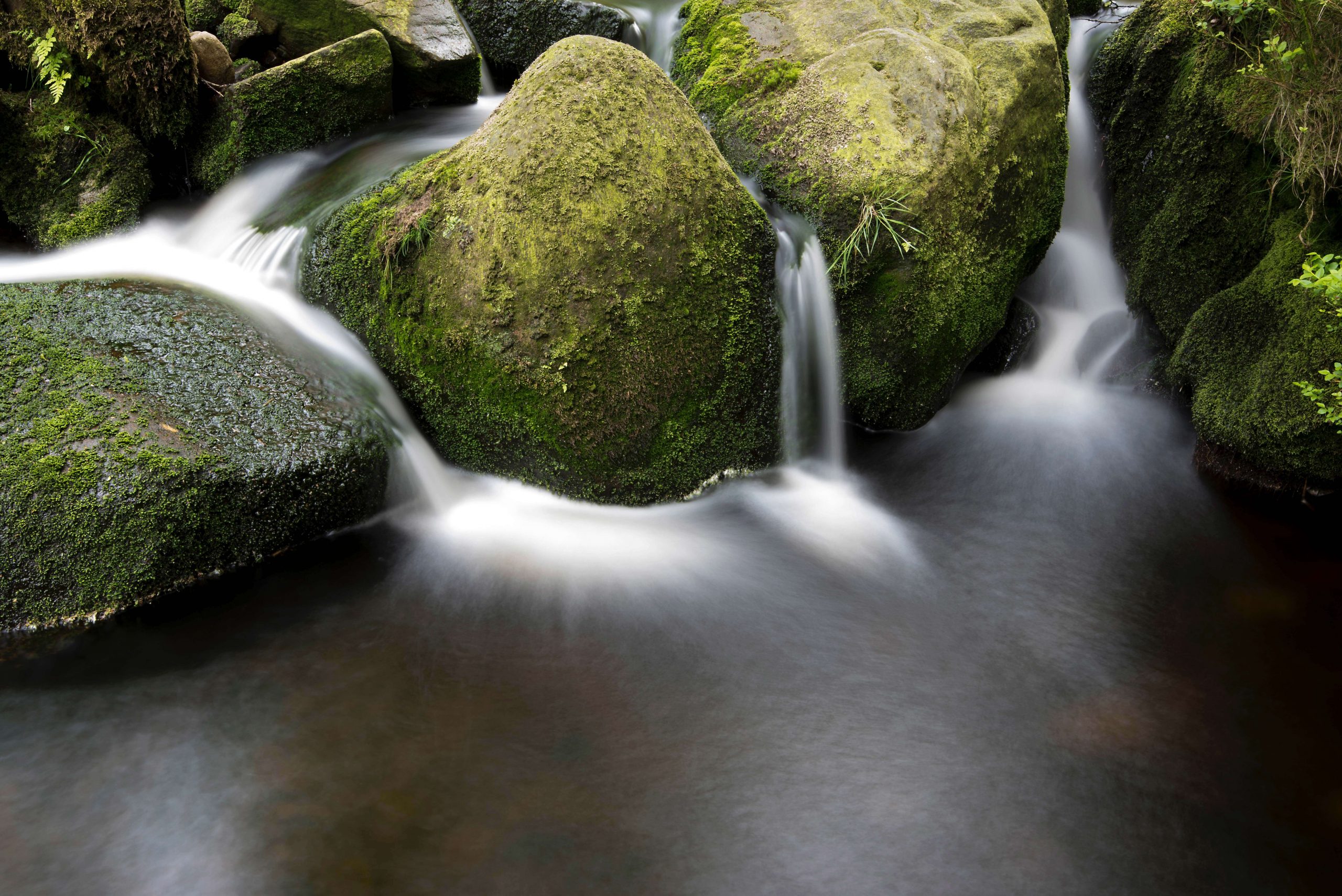
If customers are the lifeblood of modern digital business, then knowing customers’ data takes on commercial “life or death” urgency. For years, companies tried to get a picture of their customers–first through MDM technology, and later through advanced Big Data analytics. But as companies expand the number of digital touch points from just the desktop web to mobile, wearables, AI attendants, and IoT, yesterday’s methods for unifying data knowledge by centralizing data becomes less tenable. Today data easily “seeps” across structured, semi-structured and unstructured data stores; it spans the data center and the cloud; it spreads across Big Data, data lakes, and countless applications–both internal and external. Customer data is everywhere, encoded in all manner of ways, and in all types of languages.
What’s needed now is a way for companies to get a centralized view of a customer without centralizing their data. This requires an ability to search across all data stores, regardless of their location, or language, while still reconciling which data belongs to what user or entity. This necessitates fresh thinking on finding and correlating data by person or data subject, something which is not possible with Motorola StarTAC-era data classification technology found in today’s DLP and DAM products.
Centralizing The Data View Without Centralizing The Data
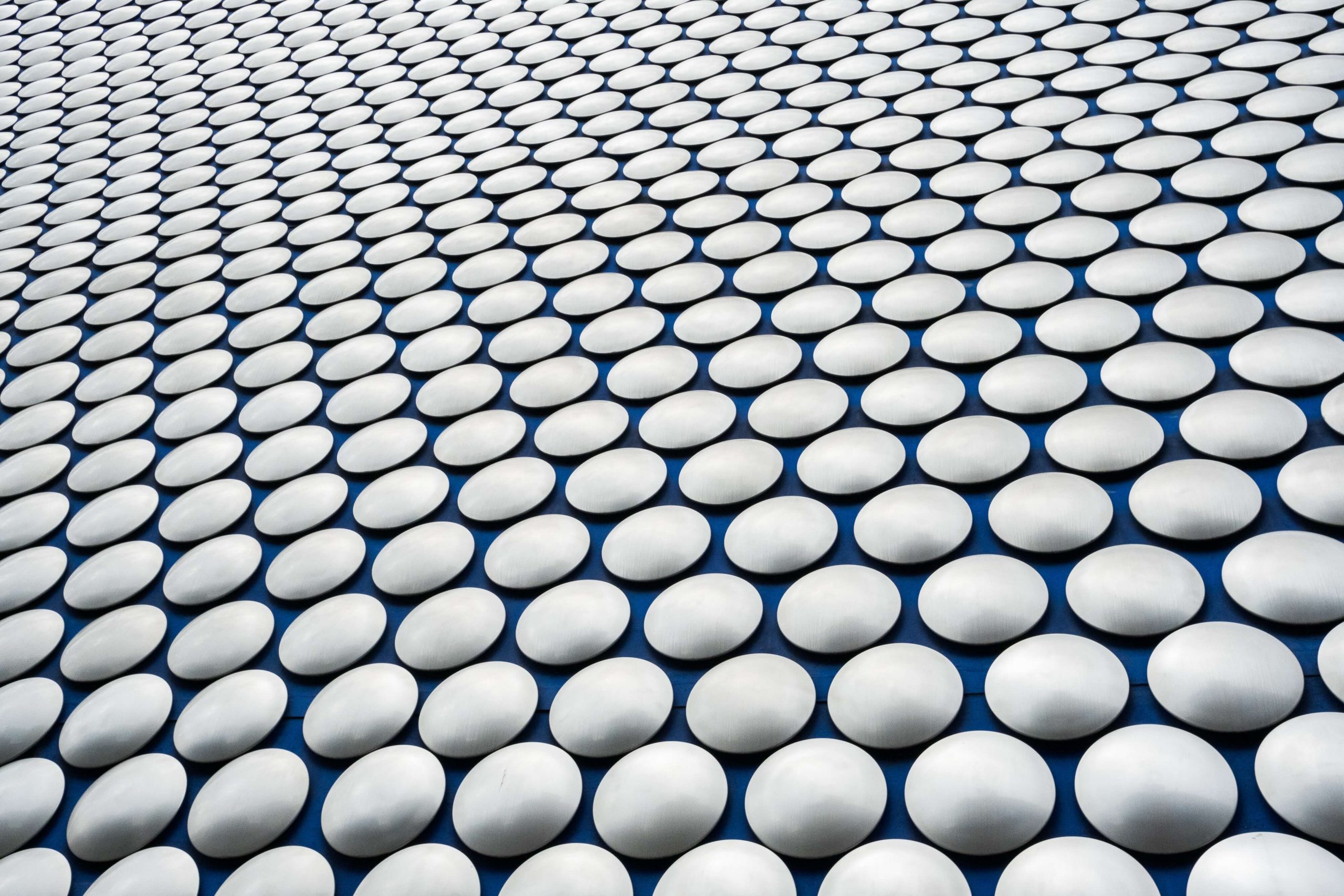
BigID takes advantage of the latest approaches in Internet-scale search and ML-based entity resolution to provide organizations a way to rapidly locate and inventory their identity-centric data without moving or copying said data. BigID algorithms automatically organize, catalogue, and map data across the enterprise at petabyte scales, regardless of where the data is stored, or how it’s encoded. It provides a virtual index of all data a company keeps, mapped by data subject, data type, data store, or residency, so that security, compliance or governance professionals can accurately navigate and analyze their core data assets. It can therefore realize a centralized view of customer data across structured data stores, but perhaps more importantly, also all the various unstructured locations where companies keep data, such as file shares, Hadoop clusters, data lakes, log repositories, and more.
Better Data Compliance Through Better Data Accounting
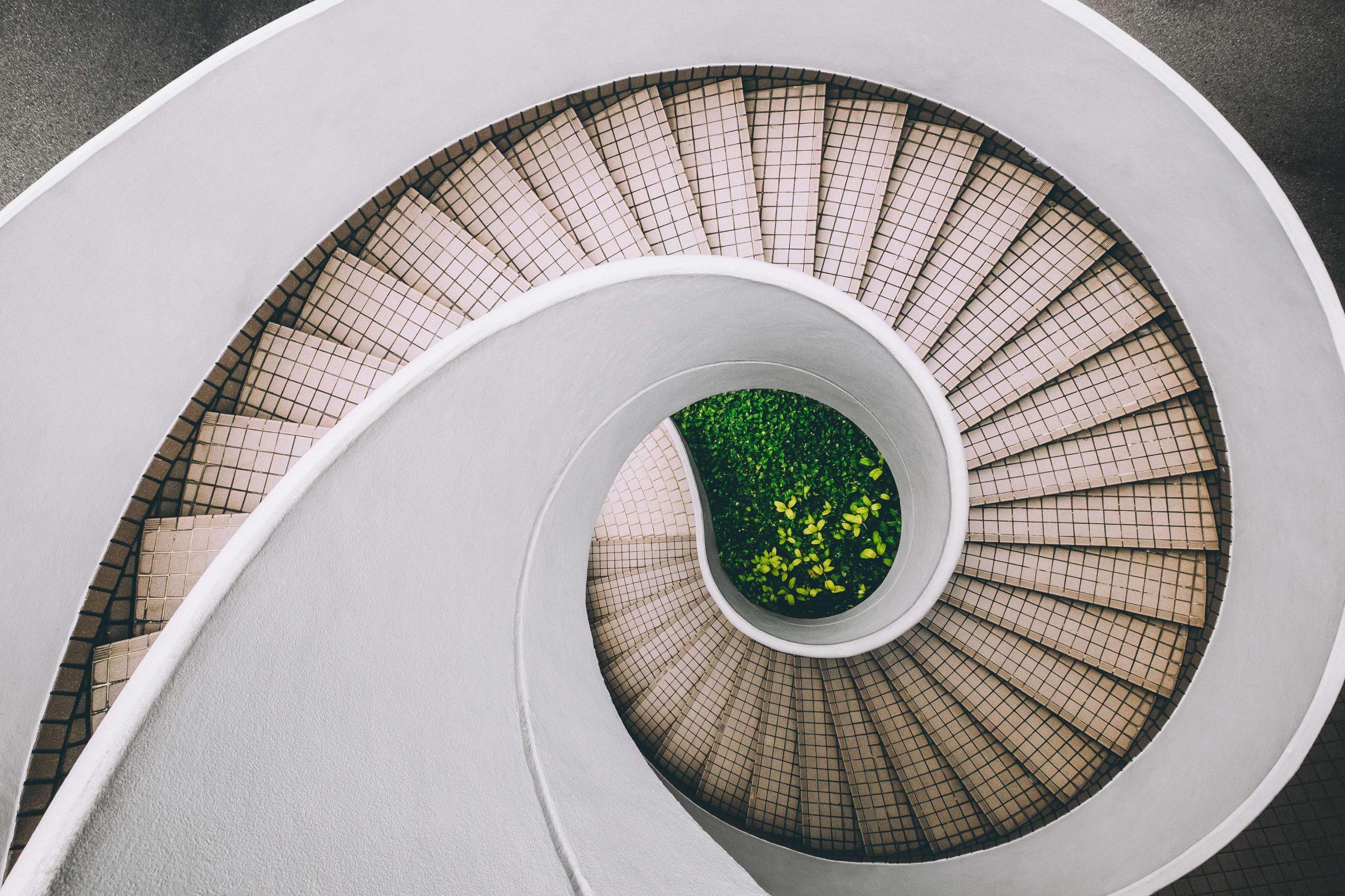
The importance of being able to discover and map personal data across unstructured data stores is not just a necessity for a more scalable, decentralized alternative to old-school MDM. It is also key if companies hope to comply with emerging data protection regulations like GDPR.
GDPR at its heart requires organizations to account for data they keep on their customers and employees. It requires them to inventory not just where they store personal data, but also where they store each person’s data. Traditional approaches to data discovery–which rely on surveys or classification–struggle locating non-PII style personal data, and can’t discern which data belongs to what person. Moreover, they have limited efficacy searching across the expanding universe of unstructured data stores, since in most cases the discovery technologies pre-date development of new modern unstructured data repositories.
GDPR & The Changing Data Protection Imperative
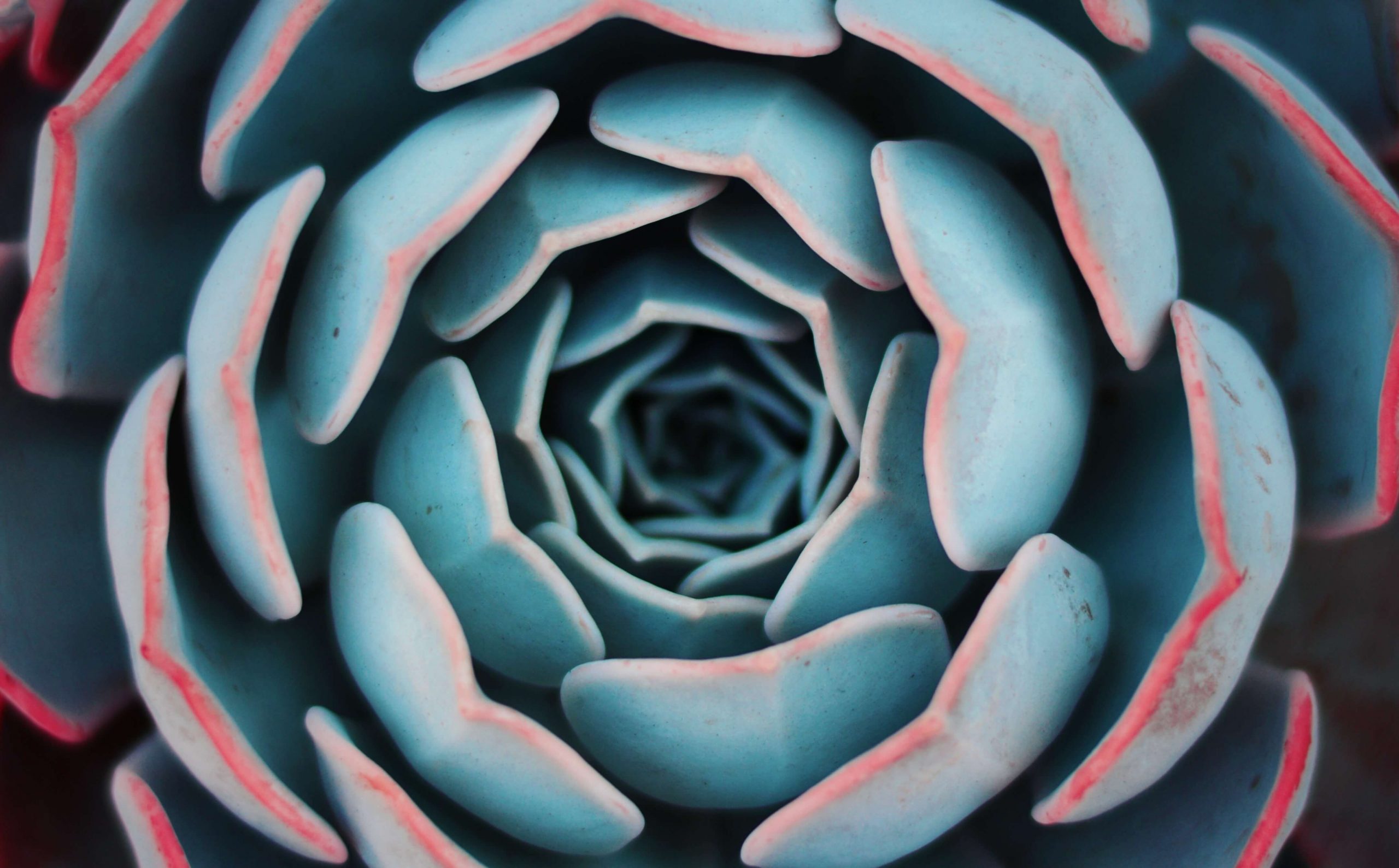
BigID can not only identify a broader set of PI/I based on how “personal” it is, but it can also do so across any data type, and independent of language. This obviously provides a powerful complement, or even alternative, to MDM. But it also helps organizations address some of the most essential GDPR data subject and record-keeping requirements by being able to find and track data from creation, through processing, and disposition.
While data may be the new “oil” in terms of its value to a modern business, unlike oil, data is not fungible. Each piece of data is distinct in its type, provenance, and associations. However, new technologies like BigID can give organization a modern way to discover and map their data accurately, at scale, and across an unlimited range of data stores. Knowing and understanding your customers through their data has never been more essential nor more feasible.