The EU GDPR at its heart is about privacy risk. Avoiding privacy violations is about understanding and mitigating privacy risk. But what is privacy risk? The EU GDPR references it 75 times but never elaborates how to measure it. Yes, it warns that risky behavior includes large-scale data processing with the intent of personal profiling. It even outlines a recommendation for removing identification risk through de-identification — but it doesn’t spell out how an organization can operationalize privacy risk monitoring and make it actionable.
Identifying Insecurity
Risk measurement has become all the rage in security over the last couple of decades. Turns out insecurity doesn’t just appear out of nothing. It’s the accumulation of small, unintentional missteps. Little errors, mistakes and poor behavior give rise to vulnerabilities. Identifying and eliminating these risk contributors won’t guarantee zero security violation but can go a long way to reducing its probability. Thus, organizations today avail themselves of tools to measure code security risk, open source risk, firewall risk, Web site risk and partner risk to give some examples. But risk in privacy has not achieved the same level of attention or operationalization.
Privacy Risk Redux
There are several reasons why privacy risk measurements never achieved the same popularity as measuring security risk. One, lawyers — the traditional overseers of privacy policy — had a natural hesitation about the term. Secondly, there was never much emphasis on technology in privacy; people and process, yes, but not product — which made quantifying risk difficult and making it actionable near impossible. Lastly, the idea of risk scoring in privacy had no catalyst to drive it forward. Security had the threat of breach or big fine regulations like SOX; privacy risk had no similar urgency — until GDPR that is.
Risk By The Numbers
The General Data Protection Regulation (GDPR), along with other new national privacy regulations, while not spelling out how to measure privacy risk nevertheless details a set of clear privacy expectations from collectors and processors of personal data. Not meeting these expectations is a potential violation of the regulation. Therefore, the data rights and obligations organizations must satisfy define one set of metrics for measuring privacy risk — assuming these metrics can be easily quantified and benchmarked. However, most enterprises will also want the flexibility to supplement these regulation-driven risk parameters with company-specific settings based on found data, metadata and data access behaviors like data type, data usage, data subject sensitivity, business process sensitivity (via data flow map), consent or access behavior. This kind of data is not always easy to discover. However, new data mapping tools like BigID make finding and using this information practical, thus making their utility in risk analysis feasible.
But once an organization adopts a data-driven data mapping tool other objective measures of risk become available too. For instance, many organizations promulgate internal policies for data retention, x-border flows, re-identifiability of anonymized data, or what data to tokenize. All can be measured and therefore used within a risk score with a data mapping tool like BigID.
Know It If You Measure It
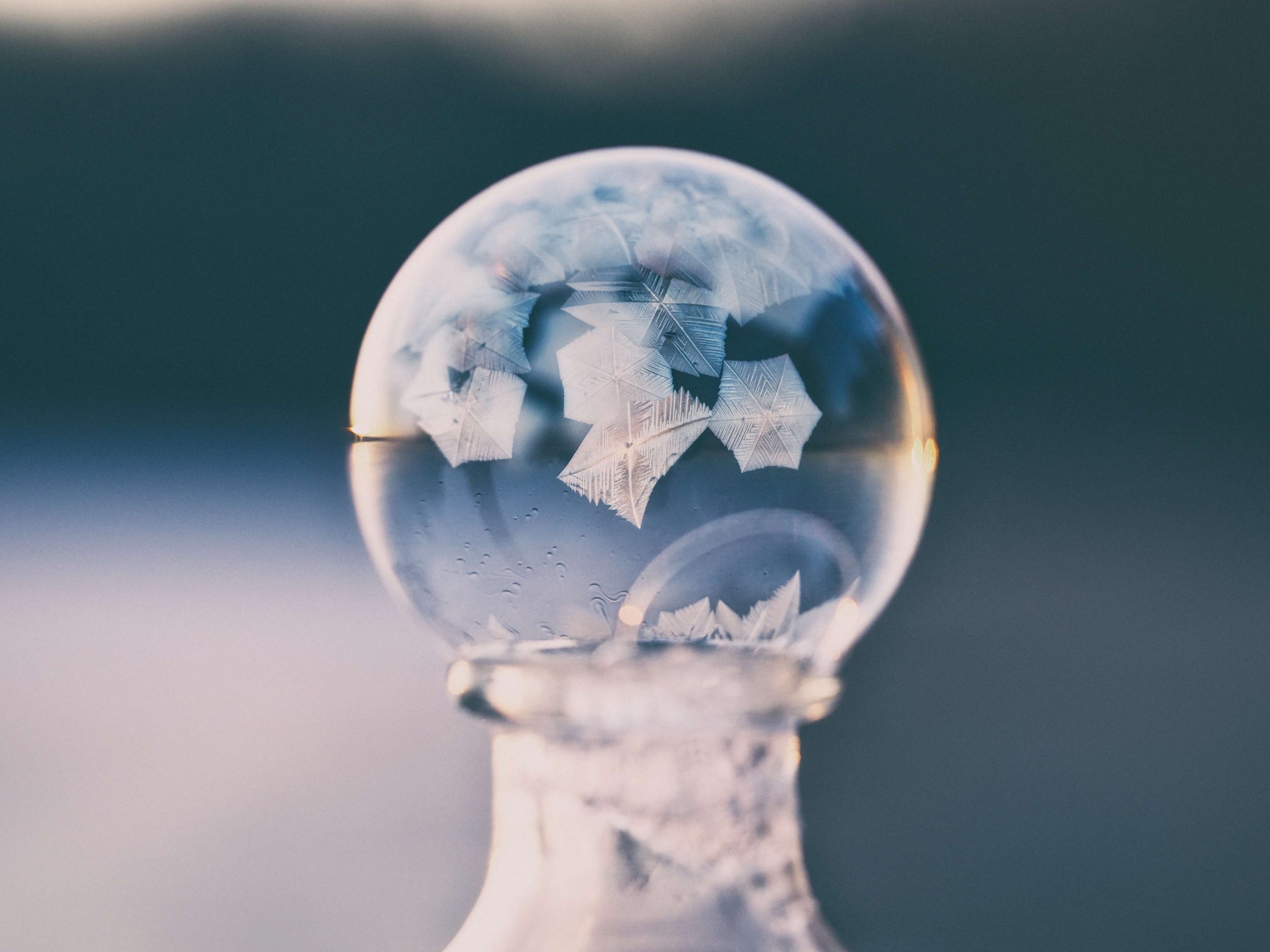
As security practitioners learned long ago measuring security risk can be instructive to managing security risk. Historically, the idea of actionable risk measurement was problematic in privacy since benchmarks were more legal than data driven. As new regulations like GDPR are phased in, however and organizations gain better insight into the data they collect and process in response, it becomes possible to move risk from a “know it if I see it” realm to one that is prescriptive and exact.