The Intersection of DataOps & Data Governance
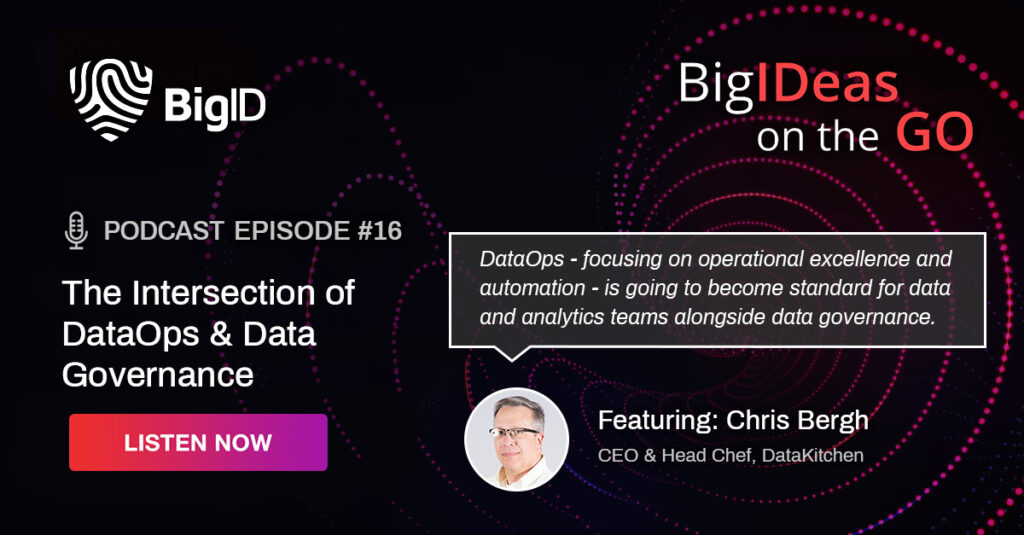
Chris Bergh, CEO of DataKitchen, joins BigIDeas on the Go to talk about the growing importance of DataOps and its intersection with data governance.
DataOps — or DevOps for Data Science
As a Software Engineer in 2005, Bergh split his professional time between building software and managing teams. “Then I got the bright idea to focus on data and analytics — and manage the teams that did data science and data engineering and visualization and governance, even before that word existed,” says Bergh.
Bergh’s idea drew inspiration from two different groups: manufacturing and software. “The sort of principles that are used in software and the principles that are used in manufacturing — they really apply to data and analytics.
“Factories have learned how to make really high-quality things like cars. Software has really learned how to deploy things into production quickly. Let’s take those two ideas and just apply them to the value chain and data analytics,” says Bergh of his plan at the time. He called this approach “DevOps for data science,” which evolved into “DataOps.”
Iterating Is Hard … Unless You Automate
Imagine, for example, something goes wrong in production — not an unusual event. Suddenly, “you’ve got to pull 10 people together and find out where it is. Is it the database? Is it the transformation? Is it the raw data?”
Alternatively, you might want to change something — but naturally, that change affects everything else. “Say I’m going to add a column to a database. Well, okay — add a column. And then what’s the transformation? What’s the visualization? What’s the model? What’s the data catalog update? All those things are changes that need to be deployed together — and deployed quickly — because the best analytic teams, I think, are focused on learning, and learning comes from iterations. Iterations are hard unless you automate.”
Check out the full podcast for more from Bergh on the future of DataOps for analytics teams.