Identifying Identity Data: Will You Know It If You See It?
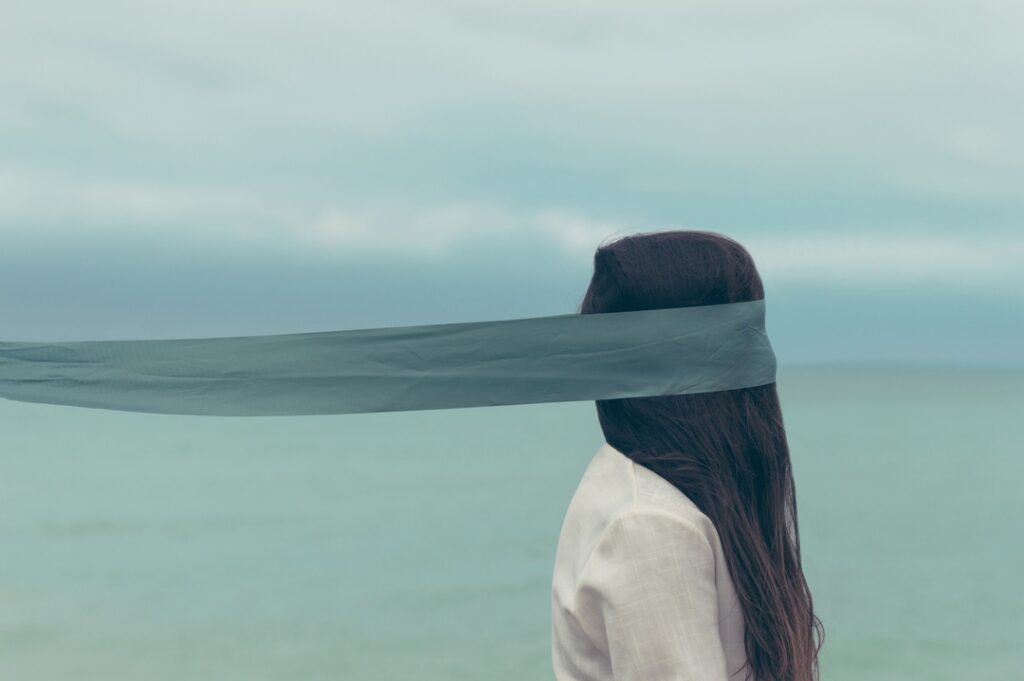
What might have been in the past a clear and binary answer to the question of what constitutes personally identifiable information (PII) will soon become more complex and intricate. Certainly, a data set that explicitly identifies a specific individual and relates their personal details remains definitively PII. However, the definition of what could or might be considered personal data looks to be shifting — and, even more significantly, expanding to personal information that is potentially identifiable. The blurring lines are the outcome of new regulations — especially, but not exclusively, the European Union’s General Data Protection Regulation — but also new concerns about the effectiveness of long standing methods to de-identify data in the online world and the growing potential to re-identify customers by joining related data sets strewn about Big Data infrastructure.
The definition of what qualifies or could be considered personal data is not simply an arcane academic debate or subject for privacy policy wonk deliberations. Instead, the emerging definitions of private data that taken into account degree of identifiability and context has very real implications for how personal data is managed. To better address compliance requirements that have both a broader and more stringent definition of personal data and reduce the attack surface requires a dynamic, flexible data management strategy that is based on real-time visibility and analytics.
Privacy Takes More Than De-identification
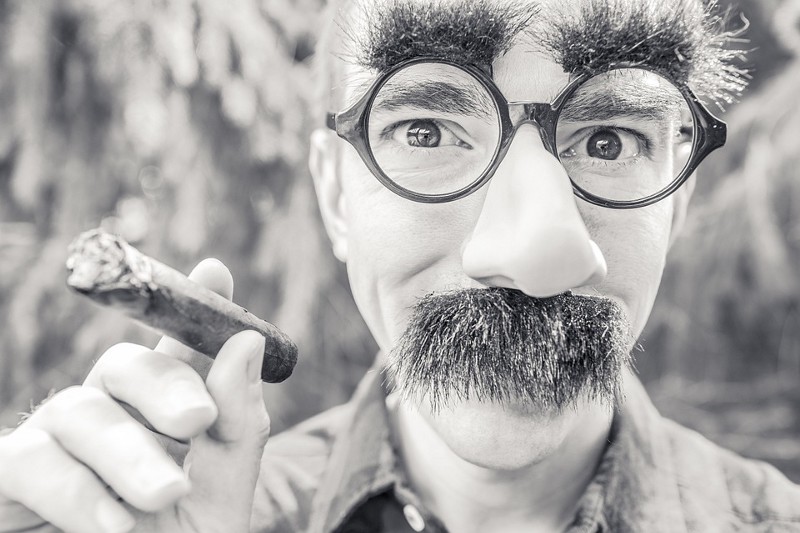
If the direction that the EU’s GPDR has taken is any indication, how to classify personal data, and by extension manage and protect it, is likely to become more of an operational challenge. The EU GDPR regulation for the first time introduces a third category of personal data, with the elegant appellation of “pseudonymization”, in addition to the existing categories of personal and anonymous data. Pseudonymous data is information that no longer allows the identification of an individual without additional information and is kept separate from it.
The new category does more than add complexity, however. On the one hand, it addresses some of the concerns about an overly broad definition of private data restricting research activities. On the other, the category is intended to undermine and discourage many accepted practices of de-identification, especially in the online world. In effect, what the category does is recast a legal definition as a technical definition.
De-identification, as the term would suggest, involves redacting specific information related to the identity of the data subject to move it into the anonymous category. In the online and mobile worlds, where cookies, tags and apps can capture vast amounts of information related to an individual, de-identification processes such as replacing personal data with a random number or hash has been used as way to anonymize data and reduce the scope of compliance requirements. By and large, advertising industry standards in the US consider such data as non-PII.
The degree of skepticism is evident in the report issued by the EU Article 29 Working Party in the run up to the finalization of the GPDR: “If pseudonymization is based on the substitution of an identity by another unique code, the presumption that this constitutes a robust de-identification is naïf and does not take into account the complexity of identification methodologies and the multifarious contexts where they might be applied.”
Hiding Identity Is Not Protecting Identity
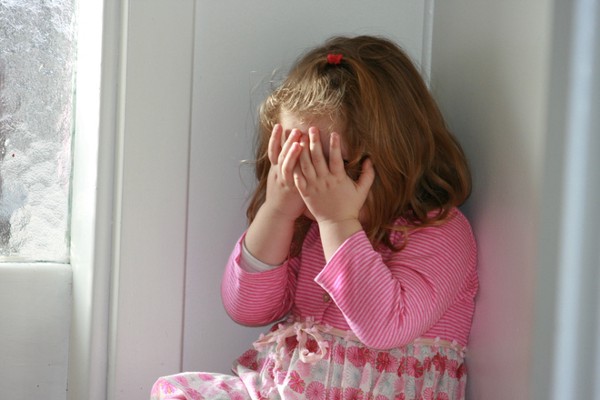
This reason for skepticism is that EU regulators believe that existing de-identification techniques fall short of stopping what they are intended to do: re-identifying specific individuals. This skepticism is also evident in the incorporation of MAC addresses as a direct identifier under the new definition of private data in the GDPR as well as proposed rules from the FCC.
Also, reading between the lines, regulators are concerned that as organizations gather, store and process large amounts of data related to an individual through online identities, cookies, tags or mobile apps, both attackers and the organizations that hold the data themselves can easily re-identify users. The potential now exists to easily thwart linear “unlinkability”.
The challenge facing organizations looking to comply with the Regulation is not only implementing data minimization to prevent accumulating copies of the same data that can be relatively easily linked. also managing what’s called data proximity within their Big Data infrastructure. Not only is the concern that the de-identification process is easily reversed by merging or linking two related data sets, but also that in the era of Big Data, attackers can easily join pieces of public and private data in a few trivial steps to re-identify a specific individual.
Privacy Compliance In An Era of Simplified Re-identification
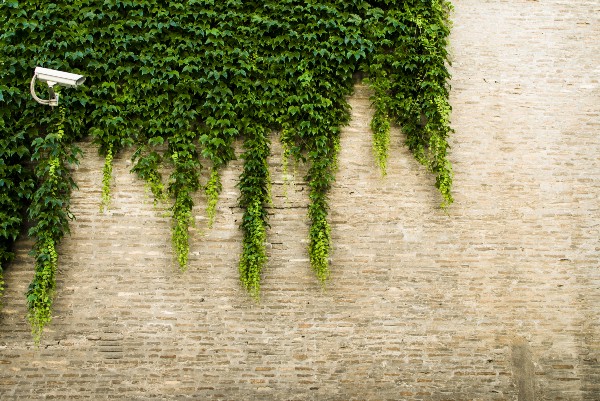
Limiting re-identification shouldn’t only be a compliance concern. While privacy, governance, data residency regulation and data security might seem at times to be at odds, this an area where risk mitigation efforts actually converge. Understanding the degree of data proximity can also help understand not only where there is a risk for falling foul of compliance concerns, and inadvertently moving data from one category to another. If data can be re-identified, it also presents a liability for risk of breach or violation of privacy policies and user consent agreements.
Security safeguards, segmentation and access controls placed on the way data are obtained, used or disseminated can mitigate risk, but a more proactive approach is needed to not only flag when explicitly private data is at risk being exposed, but also if it could be re-identified as it moves through processing flows.
Managing the risk of both inadvertent and malicious re-identification by attackers is no straightforward task, especially when organizations having to align with a mosaic of regulations, and gain visibility in multiple dimensions.
In fact, organizations could even take a probabilistic approach with both compliance and security benefits to better pinpoint the potential for re-identification if two data sources are accessed by administrators, services, APIs, employees or third parties. However, this approach is only feasible if organizations can maintain real-time visibility into their data, automate detection of risky data proximity, dynamically apply controls, or modify policies when risk is detected.