All businesses must have risk mitigation strategies, but for those who work with artificial intelligence, risk management is essential. That’s because while traditional software risk management and cybersecurity practices provide a foundation, the unique qualities of AI require a specialized approach.
This blog explains the complexities of adopting AI risk management. It discusses the fundamental concepts of risks associated with AI and implementing effective frameworks that align with the responsible deployment of these technologies.
What Are the Potential Risks Associated With AI Systems?
As artificial intelligence systems become a part of our lives, they present potential negative consequences and threats. We’ve got autonomous vehicles, algorithmic decision-making processes, generative AI systems with natural language processing abilities integrated into chatbots, and so much more. As such, these AI technologies pose risks that must be carefully managed.
AI-related risk encompasses a range of concerns. AI privacy issues can arise from unsecured data collection and analysis. Operational risks arise from security vulnerabilities specific to models that malicious actors could exploit.
There may be fairness and bias concerns in decision-making algorithms and transparency issues related to understanding how AI systems arrive at their conclusions. Finally, malicious inputs could affect safety in applications like autonomous robotics.
Effectively addressing AI-related risk requires a comprehensive understanding of these potential pitfalls and implementing strategies and frameworks to mitigate and manage risks throughout the AI lifecycle and supply chain, from development to deployment.
Difference Between AI Risk and Traditional Software Risk
Traditional software risk management focuses on issues like bugs, system failures, and security breaches. They arise from poor security practices during the build, deployment, or use of AI tools.
The risks associated with AI, on the other hand, extend beyond these concerns. These systems are built using complex algorithms trained on vast quantities of data. As a result, they often pose challenges related to bias, fairness, interpretability, and the ethical implications of automated decision-making. As such, they are ethical and legal, rather than pure security risks.
Existing risk frameworks and methodologies designed for traditional software applications are not suitable for mitigating risks in these instances.
Of course, certain features of AI systems, while risky, can also deliver substantial benefits. For instance, using pre-trained AI models and transfer learning is essential in the risk management process. It can advance research, enhance accuracy, and bolster resilience compared to alternative models and methodologies.
To use AI responsibly, it’s important to understand the context in which it’s being used. This helps AI actors—the people who work with AI, including data scientists, ML engineers, product managers, and business stakeholders—evaluate the level of risk and determine appropriate best practices and management strategies.
Unlike conventional software, data is one of the biggest risks associated with AI technologies. These systems need a lot of data to learn from and improve their output. Not all of this information might accurately or appropriately represent the context or intended use of the system. For example, one of the model risks of a medical AI system might be that it’s intended for older patients but was trained on data from mainly young people.
In some cases, there is no clear ‘correct answer’ or an actual ground truth, which makes the objective evaluation of AI outputs difficult and affects trust in AI. How do you ensure that the AI system is unbiased and accurate when you’ve nothing to base the assessment on? Issues such as harmful bias and other data quality concerns can lower confidence in AI systems.
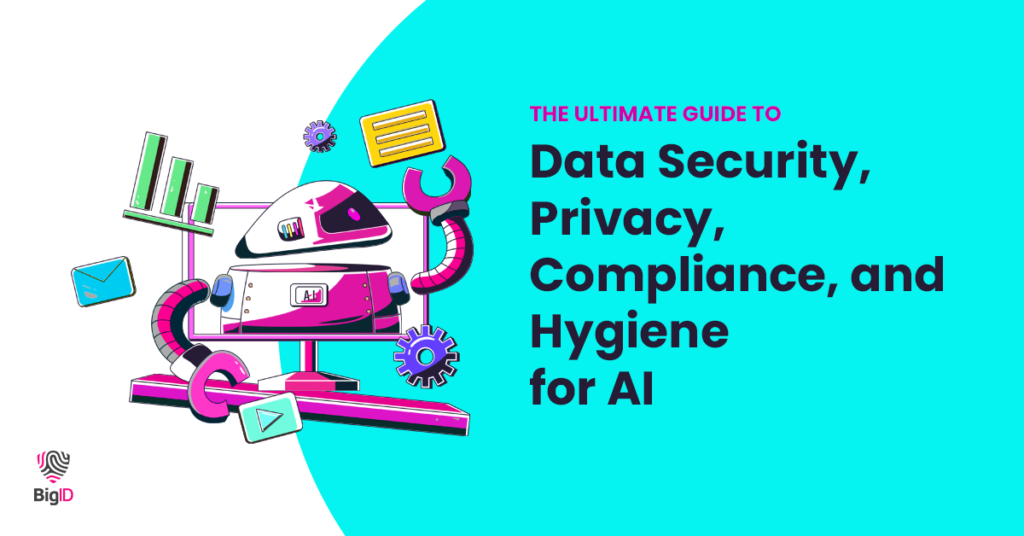
Why You Need an AI Risk Management Framework (AI RMF)
Artificial intelligence technologies can potentially revolutionize industries by automating routine tasks or unlocking valuable insights from data. However, these abilities are not without potential risk. A risk management framework, such as the NIST AI Risk Management Framework (NIST AI RMF) or the EU AI Act, is necessary to introduce AI practices that mitigate these issues and help:
Maintain Compliance
Various jurisdictions are enacting laws to govern AI systems, and compliance with these regulations is essential. However, an AI RMF needs more than just regulatory checkboxes to effectively manage risk factors. It’s a necessity because effective risk management adds to your organization’s resilience.
Instill Stakeholder Confidence
An AI RMF provides a structured framework to identify, assess, and mitigate potential risks. Risk management practices help organizations demonstrate a commitment to transparency, accountability, and responsibility. This approach to AI adoption instills confidence among stakeholders, including customers, investors, and partners.
Uphold Reputation
Social media allows news, especially bad news, to travel fast. Any incident involving AI, whether it involves biased algorithms or data breaches, can result in severe reputational damage. An RMF acts as a protective shield, which helps to anticipate and respond effectively to potential risks.
NIST AI Risk Management Framework (NIST AI RMF)
The National Institute of Standards and Technology Artificial Intelligence Risk Management Framework is a set of guidelines that help an organization develop, use, and govern its AI systems responsibly. It considers the organization’s risk tolerance, measurement, and prioritization to help it make better AI decisions. It offers a structured approach for identifying potential harm and enhancing the trustworthiness of AI systems.
The EU Artificial Intelligence Act (EU AI Act)
Where the NIST Artificial Intelligence Risk Management Framework is a guideline, the EU AI Act is a regulation that ensures that AI systems are safe and transparent. It also ensures that they uphold the fundamental rights of users through responsible AI practices. This Act classifies AI systems into four risk levels: minimal, limited, high, and unacceptable. Each level has its own set of requirements and risk responses. Systems with minimal and limited risks require little to no human oversight, while high-risk systems have stricter controls. The use of AI systems that pose unacceptable risk is forbidden.
Identifying AI Risks
To manage risks related to AI, you must identify and categorize them first. The key dimensions of AI risks span various critical areas:
Privacy
AI introduces concerns related to invasive data collection and usage. You must also be vigilant against unauthorized access to sensitive information. It’s important to recognize that, if not carefully managed, AI systems can inadvertently compromise individuals’ privacy.
Security
Critical systems face the potential of unauthorized access and vulnerability to cyber threats. As AI algorithms become increasingly integrated into your organizational frameworks, you must safeguard against these dangers, if you want to maintain integrity of operations.
Fairness
AI systems are not immune to biases. Fairness concerns arise when there is a skew in decision-making processes. You must identify and remove bias to prevent discrimination in algorithmic outcomes, to achieve equitable results across diverse user groups while using AI responsibly.
Transparency
AI decision-making is often hidden behind complex algorithms, which creates a lack of visibility into how the model makes decisions. This leads to concerns about unexplainable or opaque models. Transparency can help build trust and understanding within and outside your organization.
Safety and Performance
AI introduces a spectrum of risks associated with safety and performance. Unforeseen operational failures can have effects across the business. The performance of the model may degrade over time. You must diligently address these challenges to ensure the reliability and longevity of AI systems.
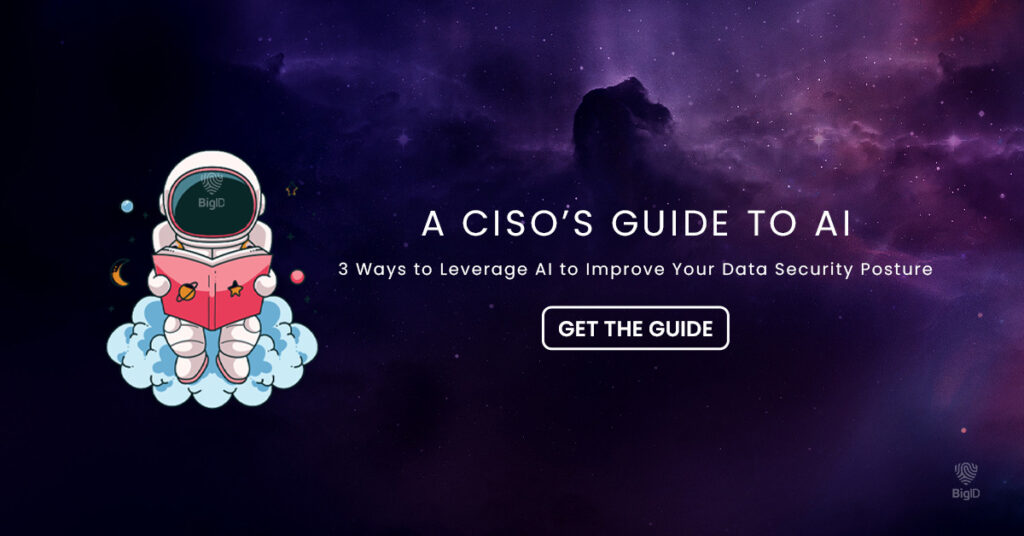
Identifying Context of These Risks
Understanding the context in which risks emerge is essential for targeted risk management and responsible AI use. The following contexts provide a comprehensive framework:
- Data: Quality, source, and usage of training material
- Model Selection and Training: Algorithmic choices and training methodologies
- Deployment and Infrastructure: Challenges associated with deploying the system
- Contracts and Insurance: Legal agreements and risk transfer mechanisms
- Legal and Regulatory: Compliance with applicable laws and regulations
- Business and Culture: Internal policies, ethical guidelines, and organizational culture
Avoiding Common Artificial Intelligence Risk Management Failures
The consequences of not managing the risks of AI can be far-reaching. You must adopt proactive strategies to avoid common pitfalls. Here are some key approaches:
Automate AI Risk Management
Manual assessment of AI risks can be time-consuming, and humans are prone to oversights. To address this, you should use AI-driven tools for risk assessment. These tools can quickly analyze vast datasets, identify potential risks and manage risks better to create trustworthy AI systems.
Real-time Validation
Static risk assessments may not be appropriate for the dynamic nature of AI operations. Instead, you should implement adaptive risk models and real-time validation mechanisms during AI operation. These continuously monitor and evaluate risk, allowing immediate responses to emerging threats and vulnerabilities.
Comprehensive Testing
Effective risk management requires thorough testing. Comprehensive evaluation across various scenarios and use cases can help identify potential weaknesses and vulnerabilities in AI systems. This includes simulated situations that mimic real-world conditions, which can provide insights into how AI performs under different circumstances.
Resource Efficiency
Inefficient use of resources can hinder the effectiveness of efforts to manage risk. Optimize resource allocation, so that the right tools, technologies, and expertise are allocated to areas where they can have the most significant impact on managing AI risks. This also helps streamline operations.
Managing AI Risks with BigID
BigID is the industry-leading DSPM platform for data privacy, security, and governance, offering intuitive and tailored solutions for enterprises of all sizes. Using advanced AI and machine learning technologies, the platform automatically scans, identifies, and correlates your organization’s data at scale—whether in the cloud or on prem, in all of its stored forms. It ensures AI systems are secure by implementing robust risk mitigation strategies such as:
- Identifying PII & Other Sensitive Data: Discover and classify both structured and unstructured data automatically to identify PII like credit card numbers, social security numbers, customer data, intellectual property, and more sensitive data across your entire landscape. Understand exactly what data you’re storing—before it’s misused in AI systems or LLM.
- Align with AI Governance Frameworks: The rapid development and use of AI is accompanied by new evolving frameworks and regulations like the AI Executive Order and the Secure AI Development Guidelines— both of which require the responsible and ethical use of AI. Our secure-by-design approach allows your organization to achieve compliance with emerging AI regulations.
- Data Minimization: Automatically identify and minimize redundant, similar, and duplicate data. Improve the data quality of AI training sets—all while reducing your attack surface and improving your organization’s security risk posture.
To start reducing the associated risk with your organization’s AI systems— schedule a 1:1 demo with BigID today.